Fourier analysis uses the Fourier series concept thought up by the mathematician Joseph Fourier. The Fourier series is a mathematical method used to represent functions as an infinite series of sine and cosine terms. The functions in question can be real- or complex-valued:
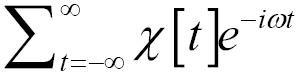
For Fourier analysis, the most competent algorithm is Fast Fourier Transform (FFT). FFT decomposes a signal into different frequency signals. This means it produces a frequency spectrum of a given signal. The SciPy and NumPy libraries provide functions for FFT.
The rfft() function performs FFT on real-valued data. We could also have used the fft() function, but it gives a warning on this Sunspot dataset. The fftshift() function moves the zero-frequency component to the middle of the spectrum.
Let's see the following example to understand FFT:
- Import the libraries and read the dataset:
# Import required library
import numpy...