Chapter 2
Overview of the Projects
Our general plan is to craft analytic, decision support modules and applications. These applications support decision-making by providing summaries of available data to the stakeholders. Decision-making spans a spectrum from uncovering new relationships among variables to confirming that data variation is random noise within narrow limits. The processing will start with acquiring data and moving it through several stages until statistical summaries can be presented.
The processing will be decomposed into several stages. Each stage will be built as a core concept application. There will be subsequent projects to add features to the core application. In some cases, a number of features will be added to several projects all combined into a single chapter.
The stages are inspired by the Extract-Transform-Load (ETL) architectural pattern. The design in this book expands on the ETL design with a number of additional steps. The words have been changed because the legacy terminology can be misleading. These features – often required for real-world pragmatic applications — will be inserted as additional stages in a pipeline.
Once the data is cleaned and standardized, then the book will describe some simple statistical models. The analysis will stop there. You are urged to move to more advanced books that cover AI and machine learning.
There are 22 distinct projects, many of which build on previous results. It’s not required to do all of the projects in order. When skipping a project, however, it’s important to read the description and deliverables for the project being skipped. This can help to more fully understand the context for the later projects.
This chapter will cover our overall architectural approach to creating a complete sequence of data analysis programs. We’ll use the following multi-stage approach:
Data acquisition
Inspection of data
Cleaning data; this includes validating, converting, standardizing, and saving intermediate results
Summarizing, and modeling data
Creating more sophisticated statistical models
The stages fit together as shown in Figure 2.1.
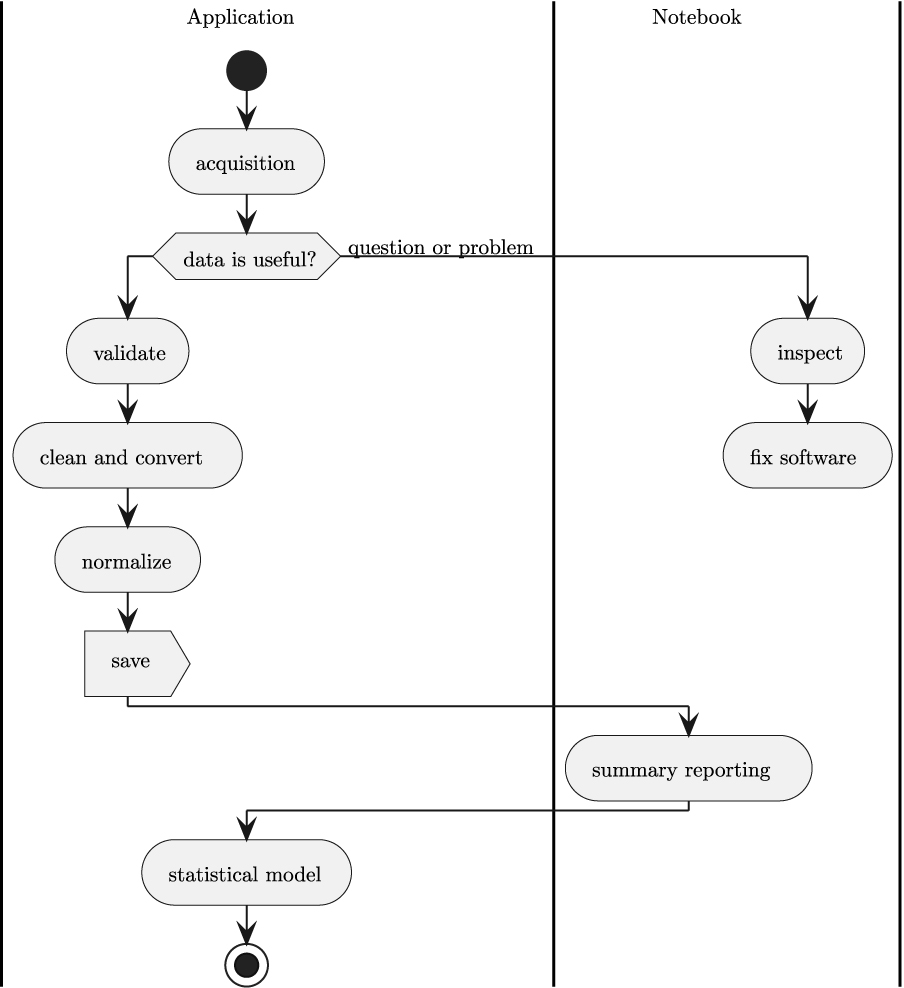
A central idea behind this is separation of concerns. Each stage is a distinct operation, and each stage may evolve independently of the others. For example, there may be multiple sources of data, leading to several distinct data acquisition implementations, each of which creates a common internal representation to permit a single, uniform inspection tool.
Similarly, data cleansing problems seem to arise almost randomly in organizations, leading to a need to add distinct validation and standardization operations. The idea is to allocate responsibility for semantic special cases and exceptions in this stage of the pipeline.
One of the architectural ideas is to mix automated applications and a few manual JupyterLab notebooks into an integrated whole. The notebooks are essential for troubleshooting questions or problems. For elegant reports and presentations, notebooks are also very useful. While Python applications can produce tidy PDF files with polished reporting, it seems a bit easier to publish a notebook with analysis and findings.
We’ll start with the acquisition stage of processing.