Many machine learning algorithms are sensitive to the scale and magnitude of the features. In particular, the coefficients of the linear models depend on the scale of the feature, that is, changing the feature scale will change the coefficients' value. In linear models, as well as algorithms that depend on distance calculations, such as clustering and principal component analysis, features with bigger value ranges tend to dominate over features with smaller ranges. Thus, having features within a similar scale allows us to compare feature importance, and also helps algorithms converge faster, thus improving performance and training times. We discussed the effect of feature magnitude on algorithm performance in more detail in the Comparing feature magnitude recipe of Chapter 1, Foreseeing Variable Problems when Building ML Models. In this chapter...
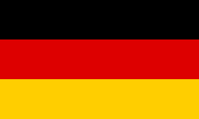
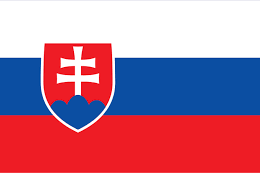
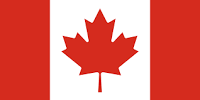
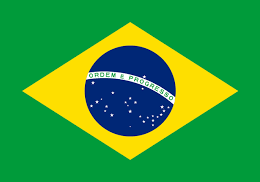

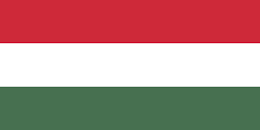
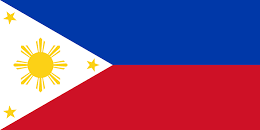
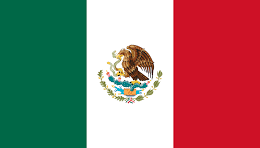
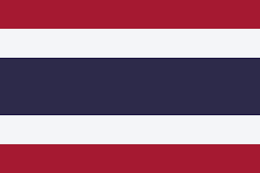
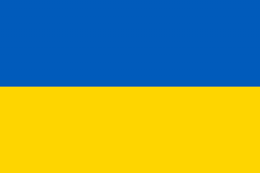
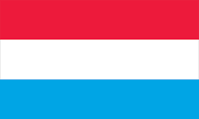
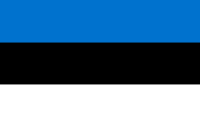
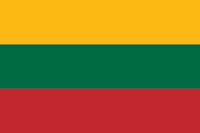
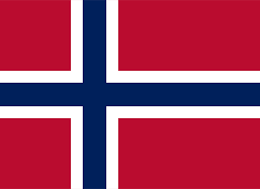
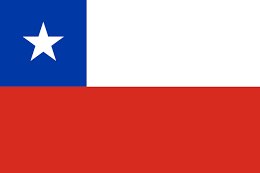
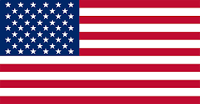
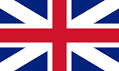
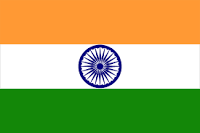
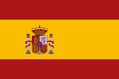
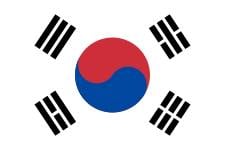
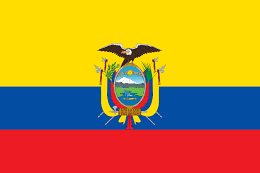
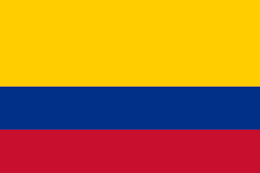
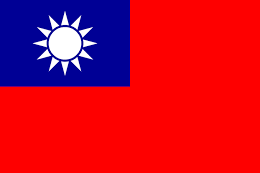
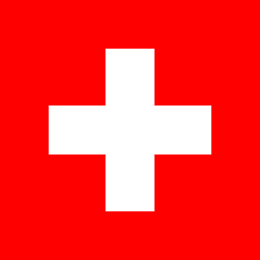
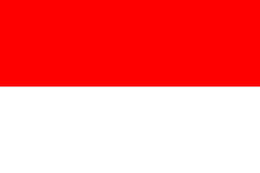
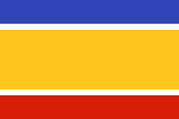
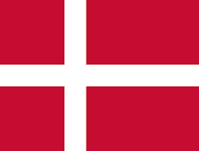
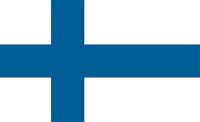
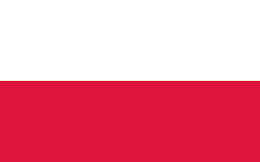
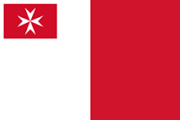
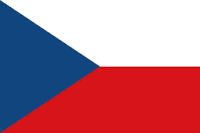
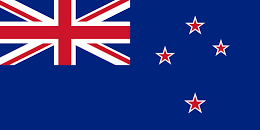
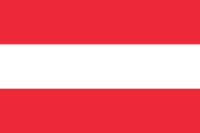
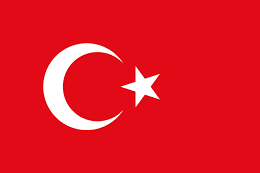
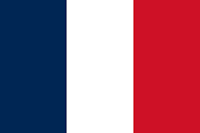
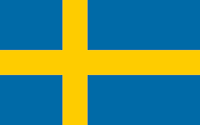
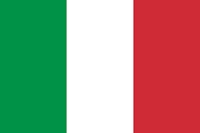
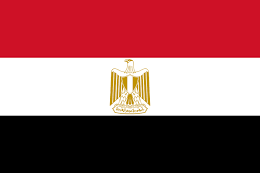
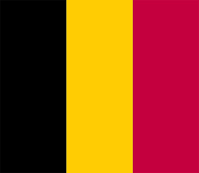
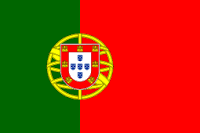
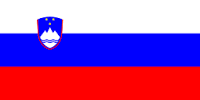
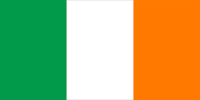
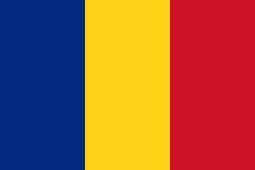
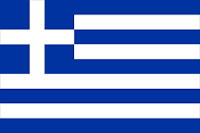
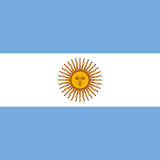
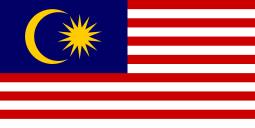
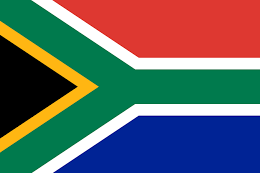
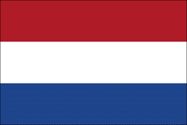
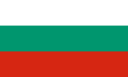
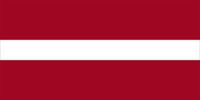
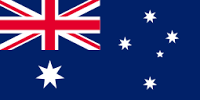
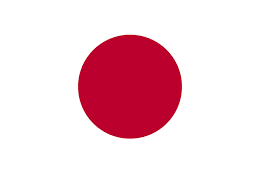
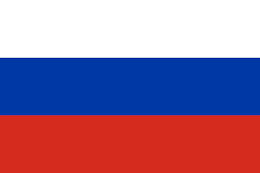