In the last chapter, we learned about the Meta-SGD and Reptile algorithm. We saw how Meta-SGD is used to find the optimal parameter, optimal learning rate, and the gradient update direction. We also saw how the Reptile algorithm works and how it is more efficient than MAML. In this chapter, we'll learn how gradient agreement is used as an optimization objective for meta learning. As you saw in MAML, we were basically taking an average of gradients across tasks and updating our model parameter. In gradient agreement algorithm, we'll take a weighted average of gradients to update a model parameter and we'll see how adding weights to the gradient helps us to find the better model parameter. We'll explore exactly how gradient agreement algorithm work in this chapter. Our gradient agreement algorithm can be plugged...
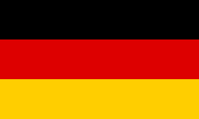
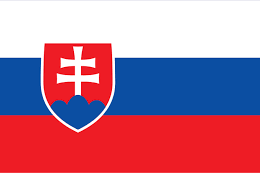
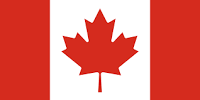
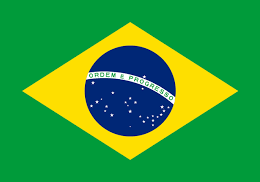

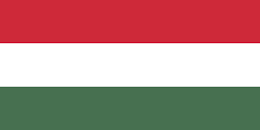
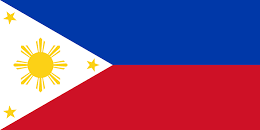
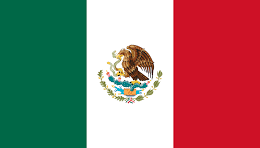
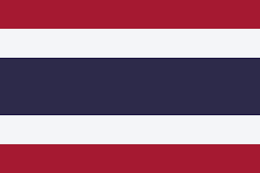
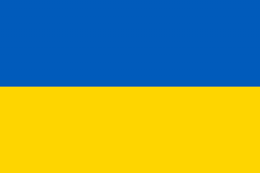
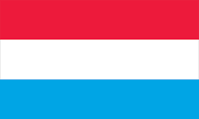
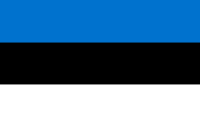
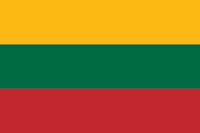
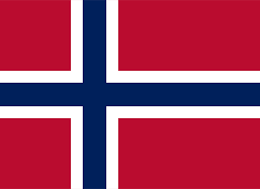
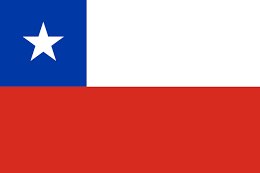
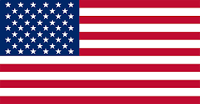
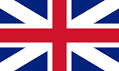
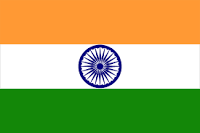
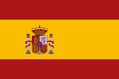
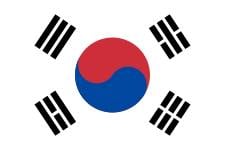
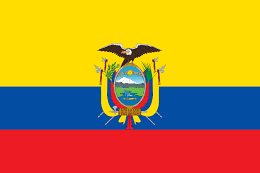
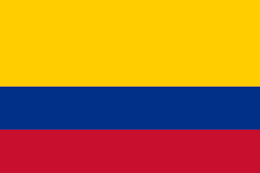
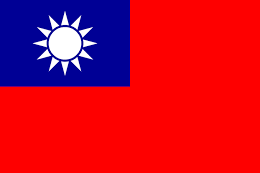
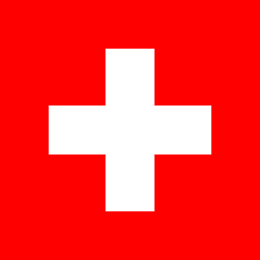
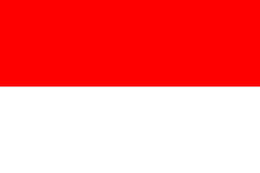
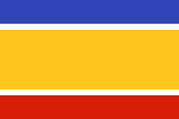
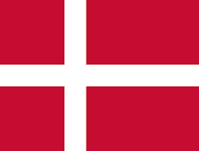
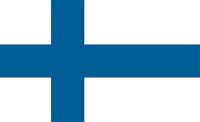
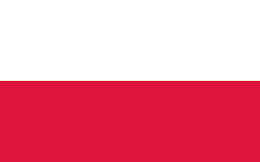
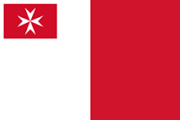
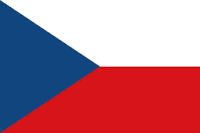
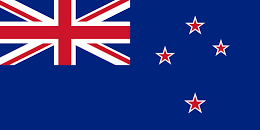
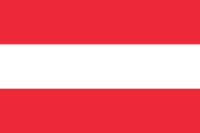
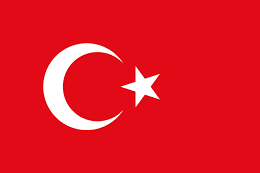
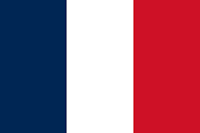
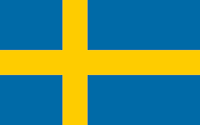
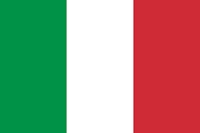
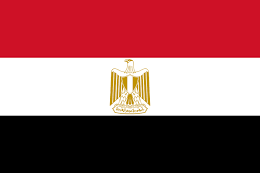
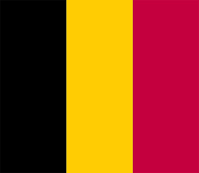
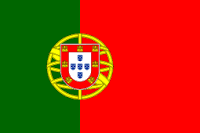
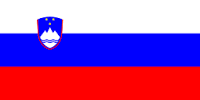
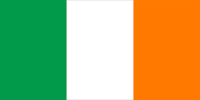
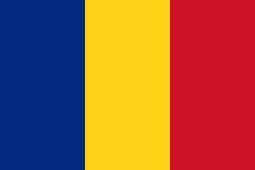
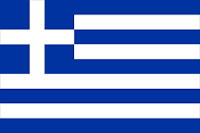
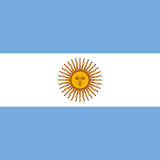
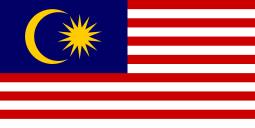
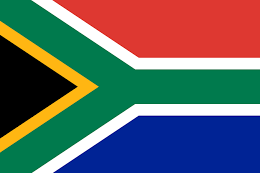
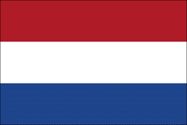
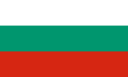
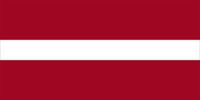
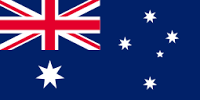
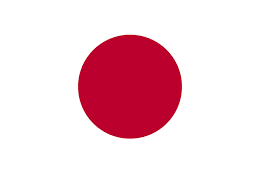
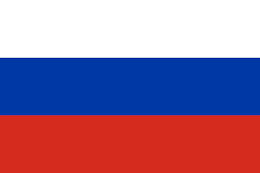