So far, we've learned how to build deep neural networks and the impact of tweaking their various hyperparameters. In this chapter, we will learn about where traditional deep neural networks do not work. We'll then learn about the inner workings of convolutional neural networks (CNNs) by using a toy example before understanding some of their major hyperparameters, including strides, pooling, and filters. Next, we will leverage CNNs, along with various data augmentation techniques, to solve the issue of traditional deep neural networks not having good accuracy. Following this, we will learn about what the outcome of a feature learning process in a CNN looks like. Finally, we'll put our learning together to solve a use case: we'll be classifying an image by stating whether the image contains a dog or a cat. By doing this...
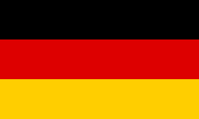
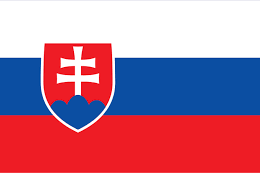
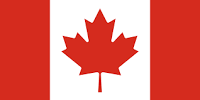
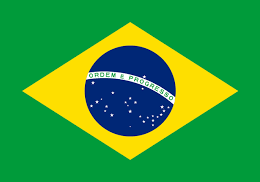

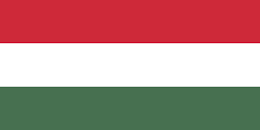
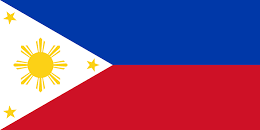
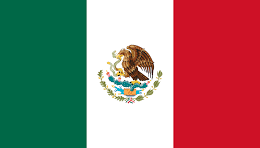
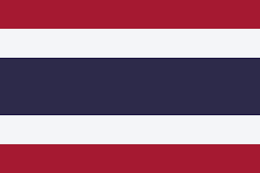
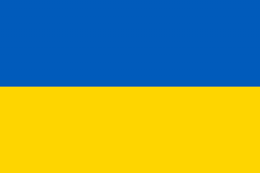
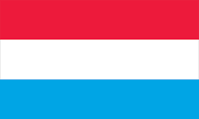
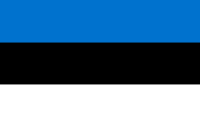
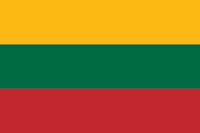
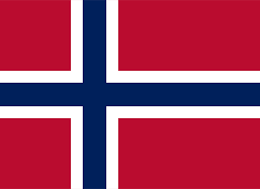
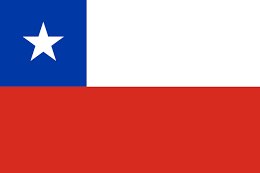
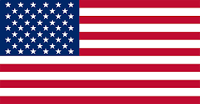
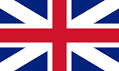
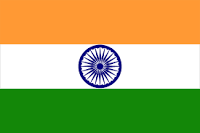
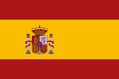
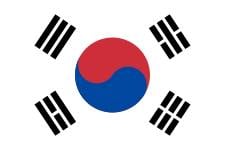
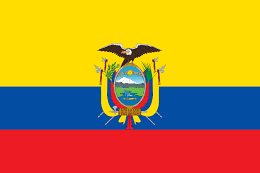
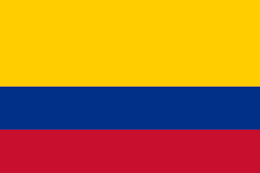
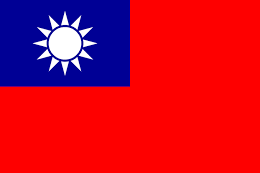
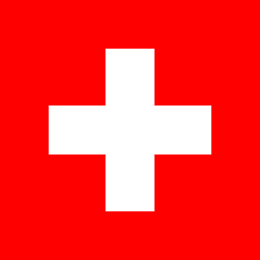
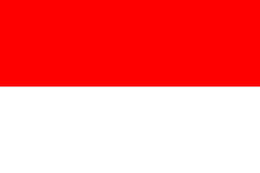
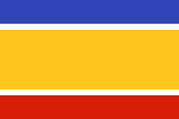
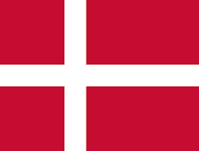
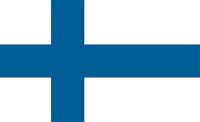
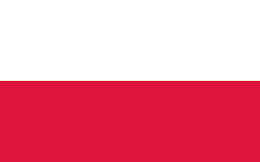
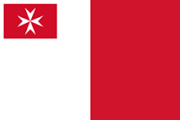
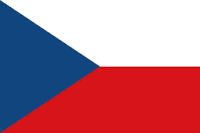
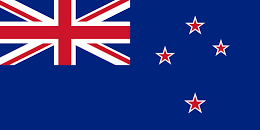
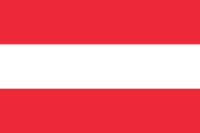
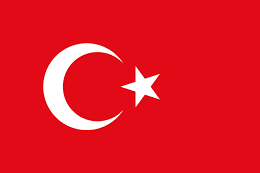
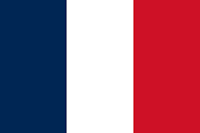
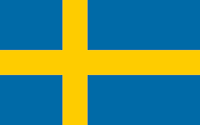
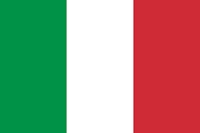
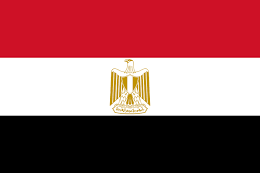
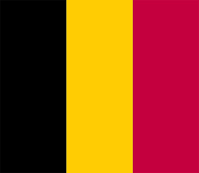
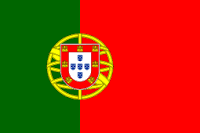
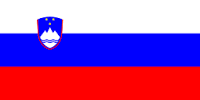
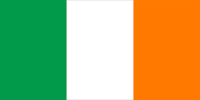
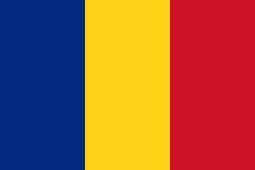
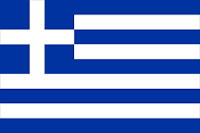
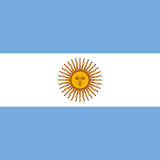
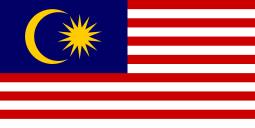
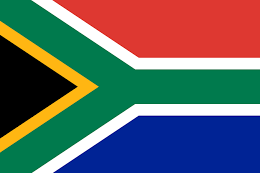
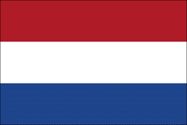
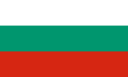
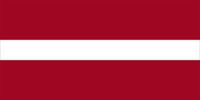
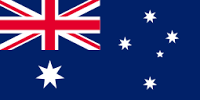
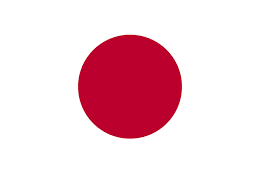
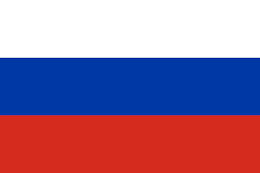