In this chapter, we'll go through a number of dimension reduction tasks. We'll look at the conditions in which dimension reduction is required and learn how to use dimension reduction algorithms efficiently in C++ with various libraries. Dimensionality reduction is where you transfer data that has a higher dimension into a new data representation with a lower dimension, all while preserving the most crucial information from the original data. Such a transformation can help us visualize multidimensional space, which can be useful in the data exploration stage or when identifying the most relevant features in dataset samples. Some machine learning (ML) techniques can perform better or faster if our data has a smaller number of features since it can consume fewer computational resources. The main purpose of this kind of transformation is to save...
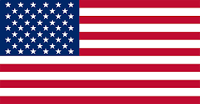
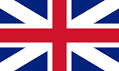
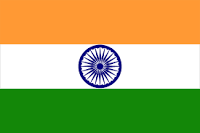
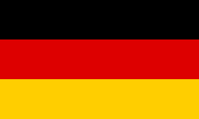
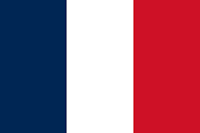
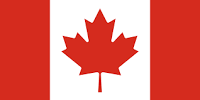
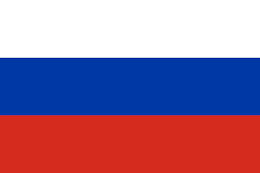
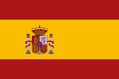
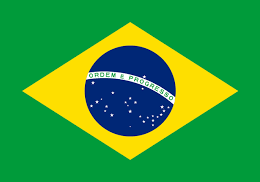
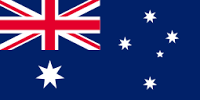

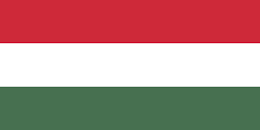
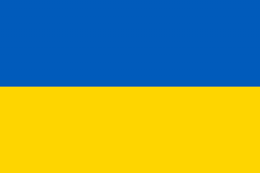
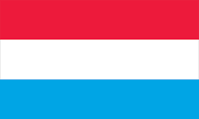
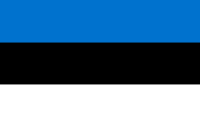
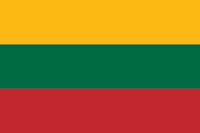
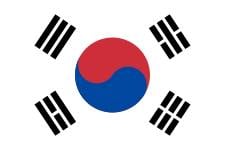
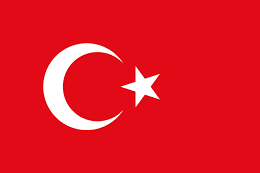
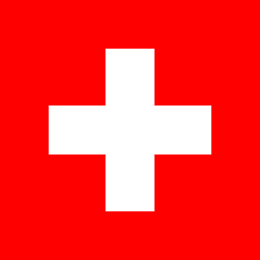
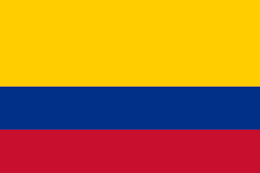
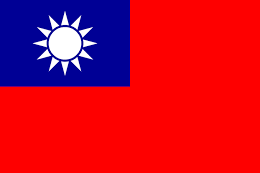
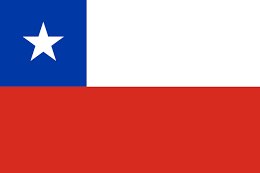
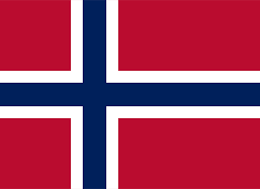
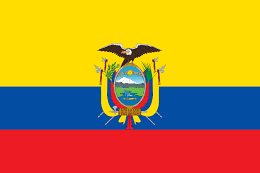
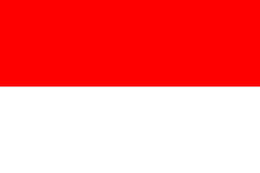
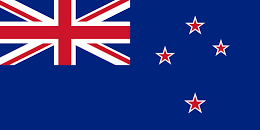
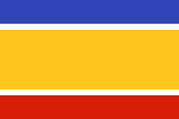
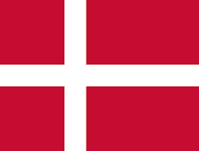
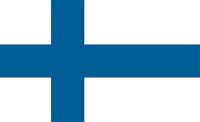
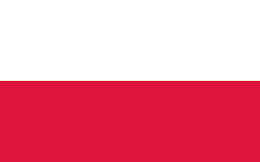
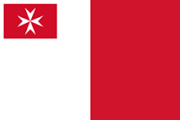
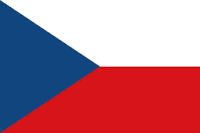
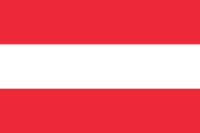
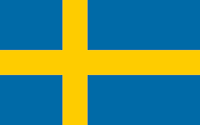
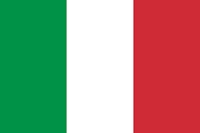
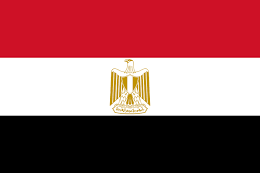
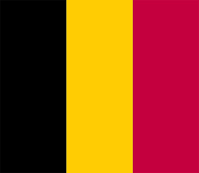
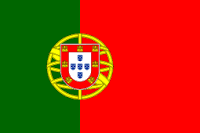
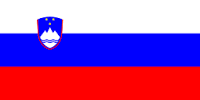
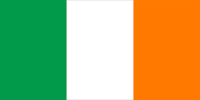
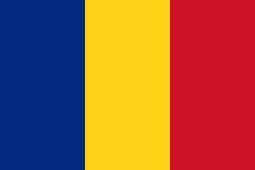
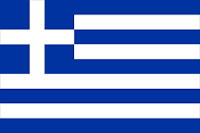
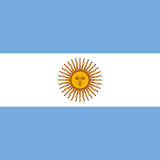
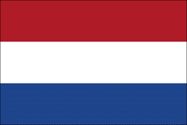
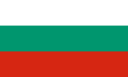
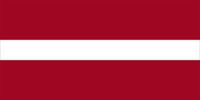
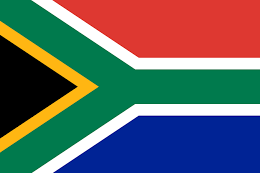
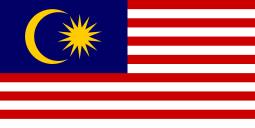
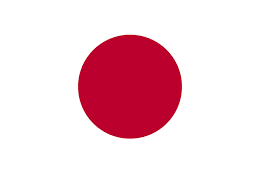
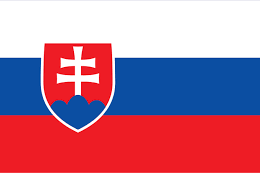
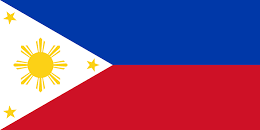
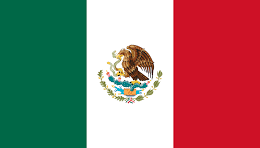
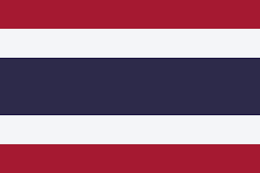