Key takeaways and lessons learned
A central goal of the book was to demonstrate the workflow of extracting signals from data using ML to inform a trading strategy. Figure 23.1 outlines this ML-for-trading workflow. The key takeaways summarized in this section relate to specific challenges we encounter when building sophisticated predictive models for large datasets in the context of financial markets:
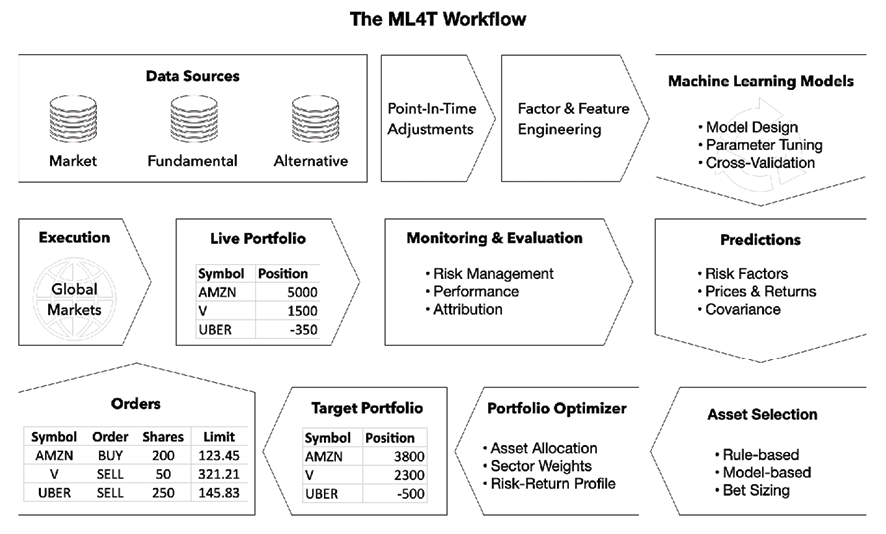
Figure 23.1: Key elements of using ML for trading
Important insights to keep in mind as you proceed to the practice of ML for trading include the following:
- Data is the single most important ingredient that requires careful sourcing and handling.
- Domain expertise is key to realizing the value contained in data and avoiding some of the pitfalls of using ML.
- ML offers tools that you can adapt and combine to create solutions for your use case.
- The choices of model objectives and performance diagnostics are key to productive iterations toward...