This book introduces data mining with popular free Python libraries. It is written in a conversational style, aiming to be approachable while imparting intuition on the reader. Data mining is a broad field of analytical methods designed to uncover insights from your data that are not obvious or discoverable by conventional analysis techniques. The field of data mining is vast, so the topics in this quick start guide were chosen by their relevance to not only their field of origin, but also the adjacent applications of machine learning and artificial intelligence. After a procedural first half, focused on getting the reader comfortable with data collection, loading, and munging, the book will move to a completely conceptual discussion. The concepts are introduced from first principles intuition and broadly grouped as transformation, clustering, and prediction. Popular methods such as principal component analysis, k-means clustering, support vector machines, and random forest are all covered in the conceptual second half of the book. The book ends with a discussion on pipe-lining and deploying your analytical models.
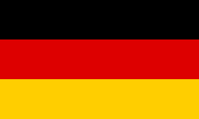
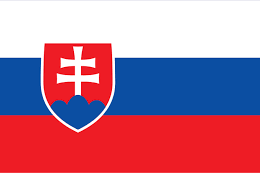
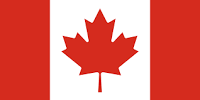
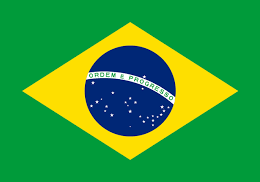

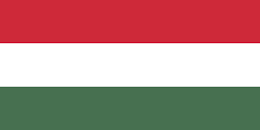
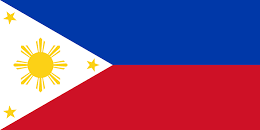
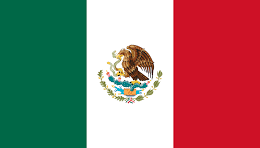
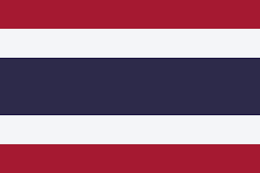
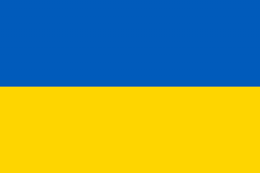
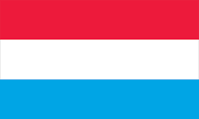
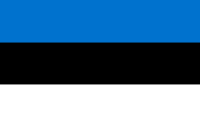
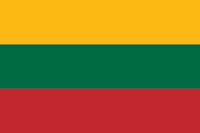
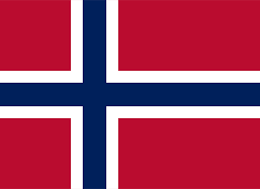
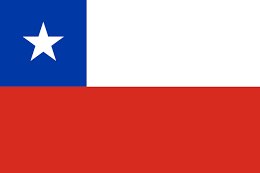
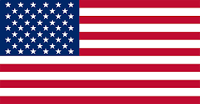
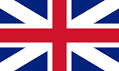
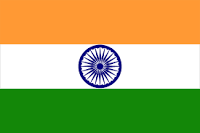
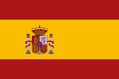
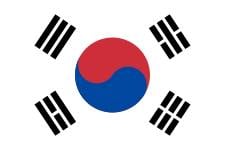
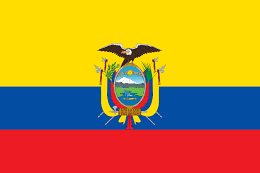
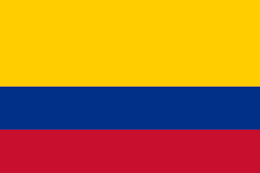
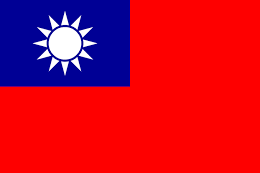
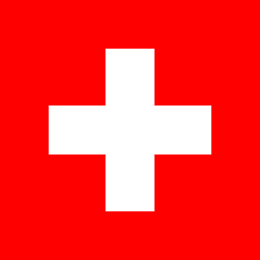
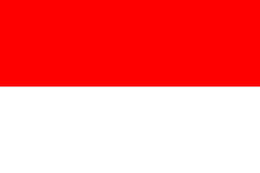
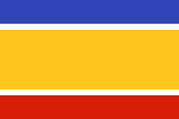
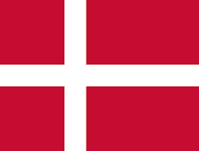
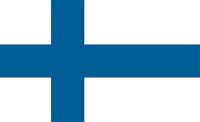
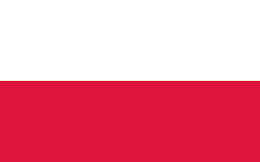
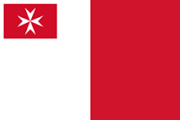
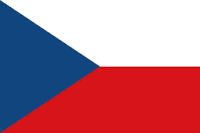
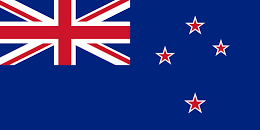
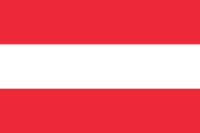
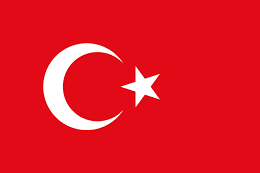
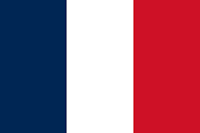
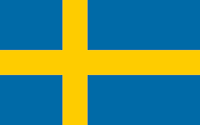
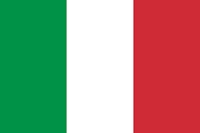
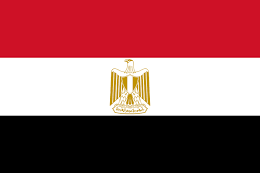
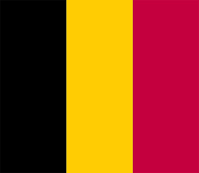
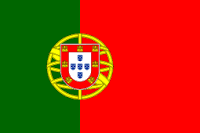
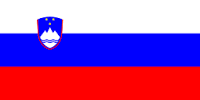
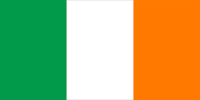
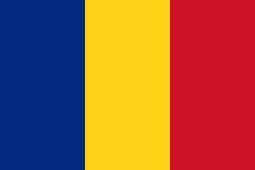
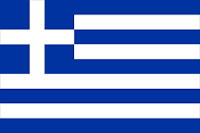
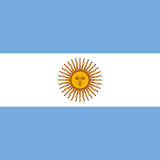
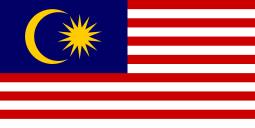
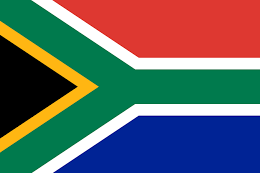
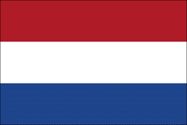
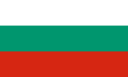
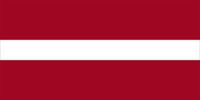
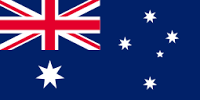
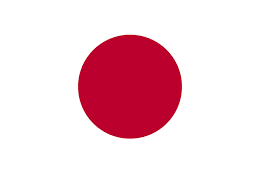
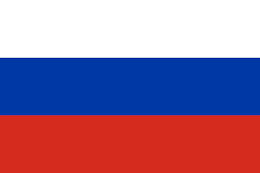