In every machine learning project, an algorithm learns rules and instructions from a training dataset, with a view to performing a task better. In reinforcement learning (RL), the algorithm is called the agent, and it learns from the data provided by an environment. Here, the environment is a continuous source of information that returns data according to the agent's actions. And, because the data returned by an environment could be potentially infinite, there are many conceptual and practical differences among the supervised settings that arise while training. For the purpose of this chapter, however, it is important to highlight the fact that different environments not only provide different tasks to accomplish, but can also have different types of input, output, and reward signals, while also requiring the adaptation of the algorithm...
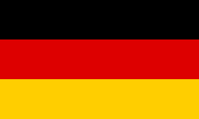
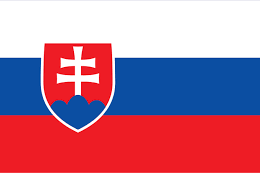
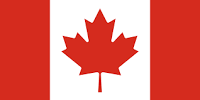
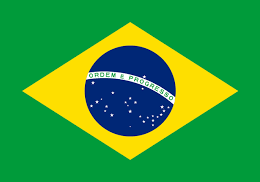

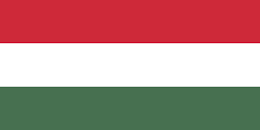
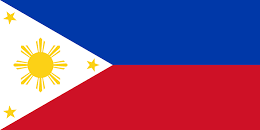
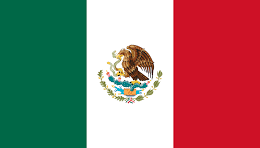
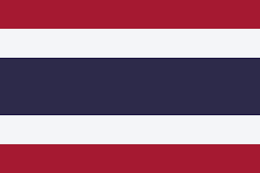
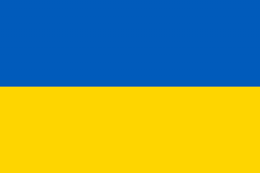
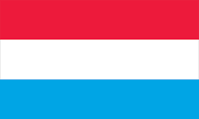
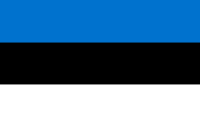
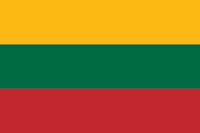
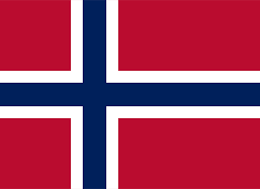
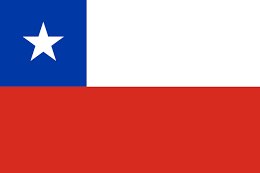
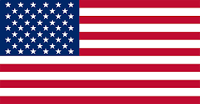
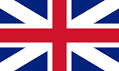
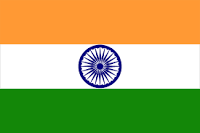
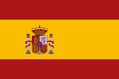
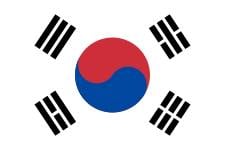
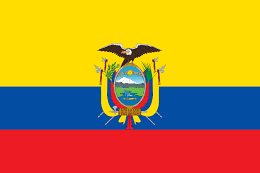
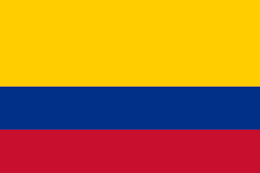
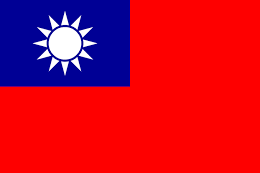
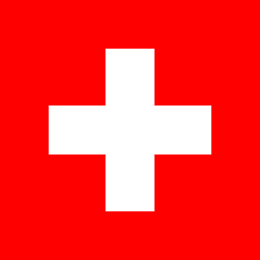
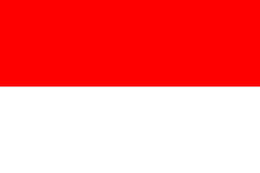
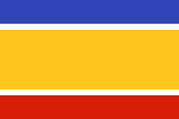
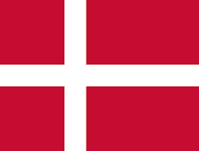
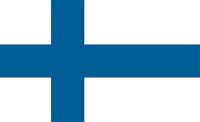
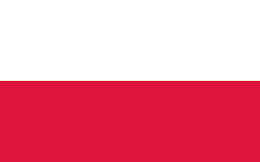
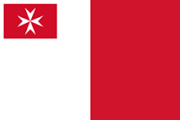
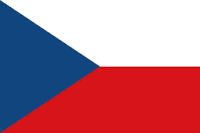
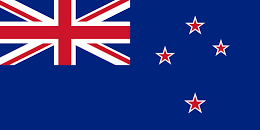
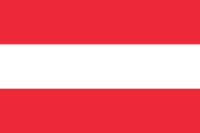
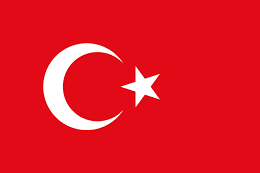
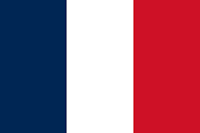
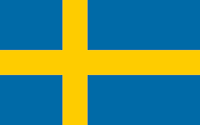
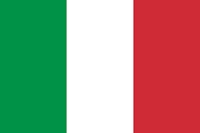
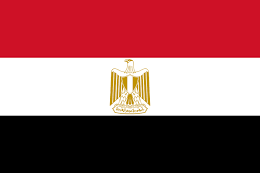
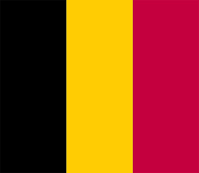
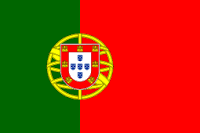
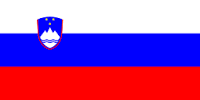
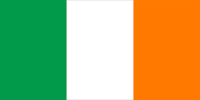
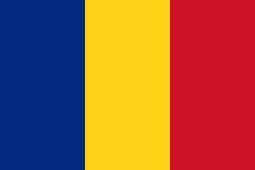
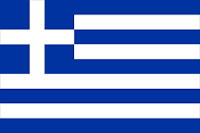
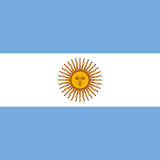
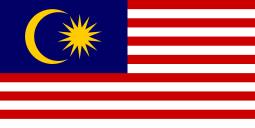
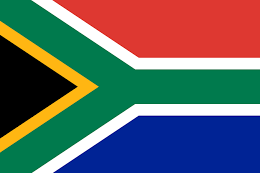
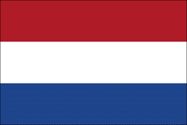
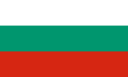
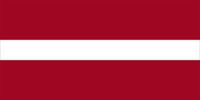
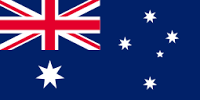
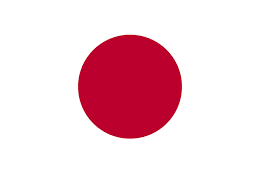
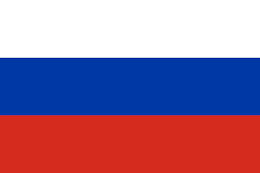