Deep learning taxonomy
The feature learning taxonomy for deep learning cases is depicted here:
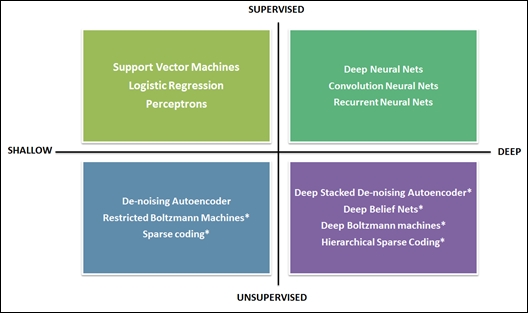
Some of the frameworks that are used to implement neural network applications are listed here:
- Theano is a Python library
- Torch a Lua programming language
- Deeplearning4J is an open, source Java-based framework that works with Spark and Hadoop
- Caffe is a C++ based framework
Convolutional neural networks (CNN/ConvNets)
CNN, also known as convolution nets (ConvNets), are a variation of the regular neural networks.
Let us recap the function of the regular neural network. Regular neural networks have a single vector-based input that is transformed through a series of hidden layers where the neurons in each layer are connected with the neurons in its neighboring layers. The last layer in this series provides the output. This layer is called the output layer.
When the input to the neural network is an image and does not just fit into a single vector structure, the complexity grows. CNN have this slight variation...