Efficient data storage with pandas
We'll be using many different datasets in this book, and it's worth comparing the main formats for efficiency and performance. In particular, we'll compare the following:
- CSV: Comma-separated, standard flat text file format.
- HDF5: Hierarchical data format, developed initially at the National Center for Supercomputing Applications. It is a fast and scalable storage format for numerical data, available in pandas using the PyTables library.
- Parquet: Part of the Apache Hadoop ecosystem, a binary, columnar storage format that provides efficient data compression and encoding and has been developed by Cloudera and Twitter. It is available for pandas through the pyarrow library, led by Wes McKinney, the original author of pandas.
The storage_benchmark.ipynb
notebook compares the performance of the preceding libraries using a test DataFrame that can be configured to contain numerical or text data, or both. For the HDF5 library, we test both the fixed and table formats. The table format allows for queries and can be appended to.
The following charts illustrate the read and write performance for 100,000 rows with either 1,000 columns of random floats and 1,000 columns of a random 10-character string, or just 2,000 float columns (on a log scale):
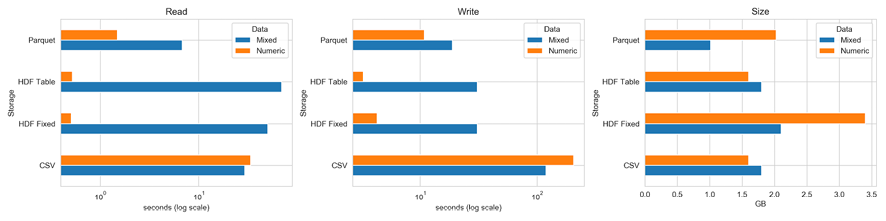
Figure 2.12: Storage benchmarks
The left panel shows that, for purely numerical data, the HDF5 format performs best by far, and the table format also shares with CSV the smallest memory footprint at 1.6 GB. The fixed
format uses twice as much space, while the parquet
format uses 2 GB.
For a mix of numerical and text data, Parquet is the best choice for read and write operations. HDF5 has an advantage with read in relation to CSV, but it is slower with write because it pickles text data.
The notebook illustrates how to configure, test, and collect the timing using the %%timeit
cell magic and, at the same time, demonstrates the usage of the related pandas commands that are required to use these storage formats.