Deep learning models are known to be difficult to interpret. Some approaches to model interpretability, including LIME, allow us to gain some insights into how the model came to its conclusions. Before we demonstrate LIME, I will show how different data distributions and / or data leakage can cause problems when building deep learning models. We will reuse the deep learning churn model from Chapter 4, Training Deep Prediction Models, but we are going to make one change to the data. We are going to introduce a bad variable that is highly correlated to the y value. We will only include this variable in the data used to train and evaluate the model. A separate test set from the original data will be kept to represent the data the model will see in production, this will not have the bad variable in it. The creation of this bad variable...
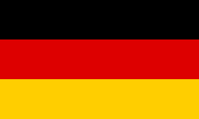
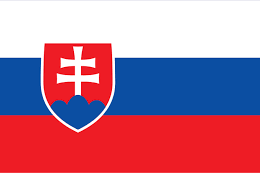
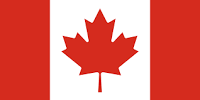
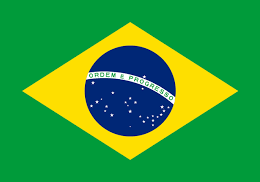

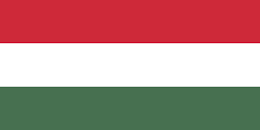
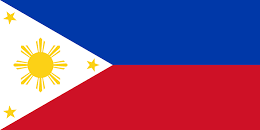
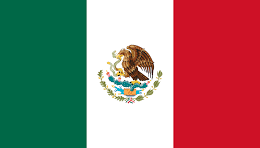
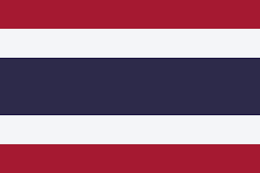
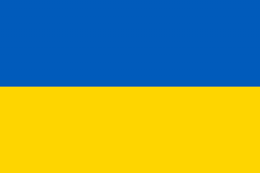
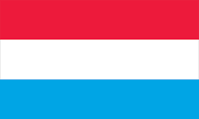
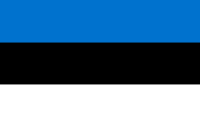
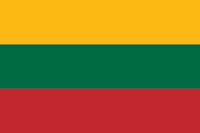
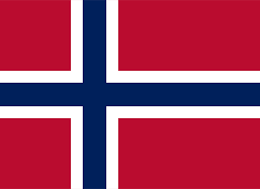
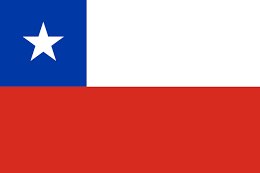
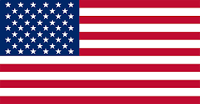
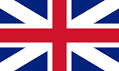
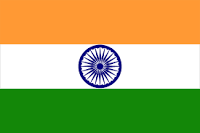
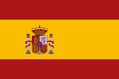
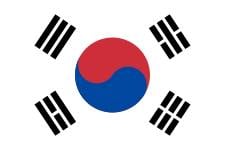
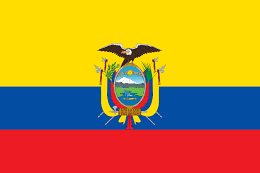
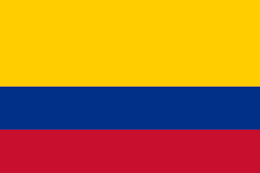
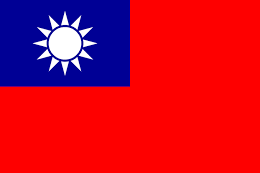
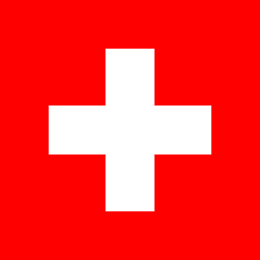
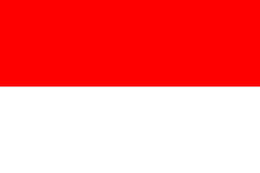
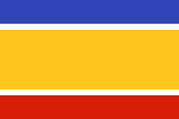
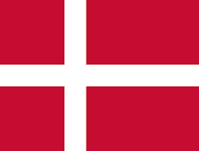
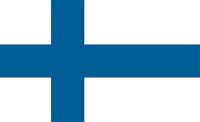
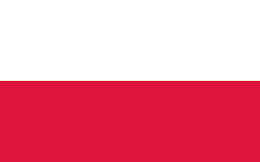
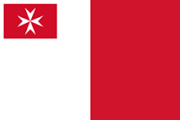
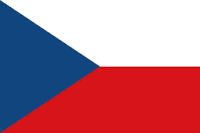
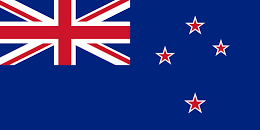
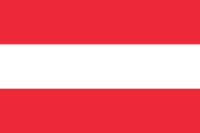
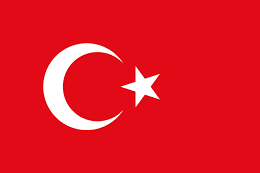
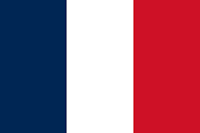
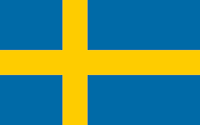
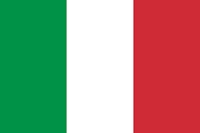
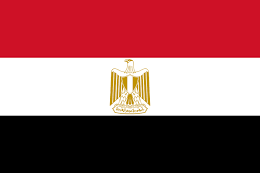
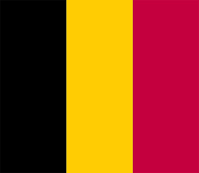
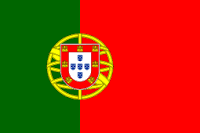
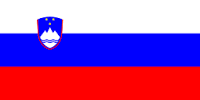
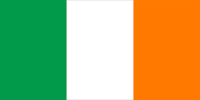
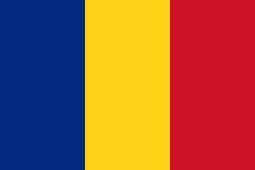
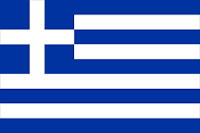
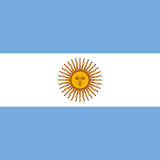
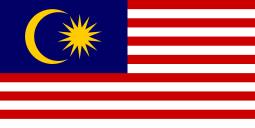
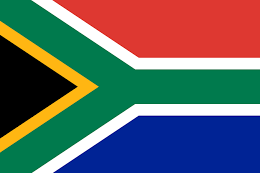
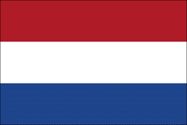
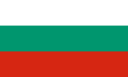
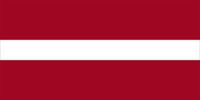
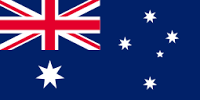
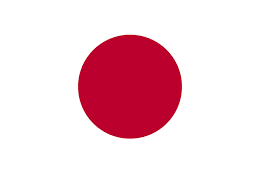
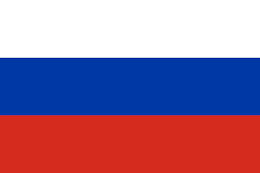