Introduction to ML Engineering on AWS
Most of us started our machine learning (ML) journey by training our first ML model using a sample dataset on our laptops or home computers. Things are somewhat straightforward until we need to work with much larger datasets and run our ML experiments in the cloud. It also becomes more challenging once we need to deploy our trained models to production-level inference endpoints or web servers. There are a lot of things to consider when designing and building ML systems and these are just some of the challenges data scientists and ML engineers face when working on real-life requirements. That said, we must use the right platform, along with the right set of tools, when performing ML experiments and deployments in the cloud.
At this point, you might be wondering why we should even use a cloud platform when running our workloads. Can’t we build this platform ourselves? Perhaps you might be thinking that building and operating your own data center is a relatively easy task. In the past, different teams and companies have tried setting up infrastructure within their data centers and on-premise hardware. Over time, these companies started migrating their workloads to the cloud as they realized how hard and expensive it was to manage and operate data centers. A good example of this would be the Netflix team, which migrated their resources to the AWS cloud. Migrating to the cloud allowed them to scale better and allowed them to have a significant increase in service availability.
The Amazon Web Services (AWS) platform provides a lot of services and capabilities that can be used by professionals and companies around the world to manage different types of workloads in the cloud. These past couple of years, AWS has announced and released a significant number of services, capabilities, and features that can be used for production-level ML experiments and deployments as well. This is due to the increase in ML workloads being migrated to the cloud globally. As we go through each of the chapters in this book, we will have a better understanding of how different services are used to solve the challenges when productionizing ML models.
The following diagram shows the hands-on journey for this chapter:
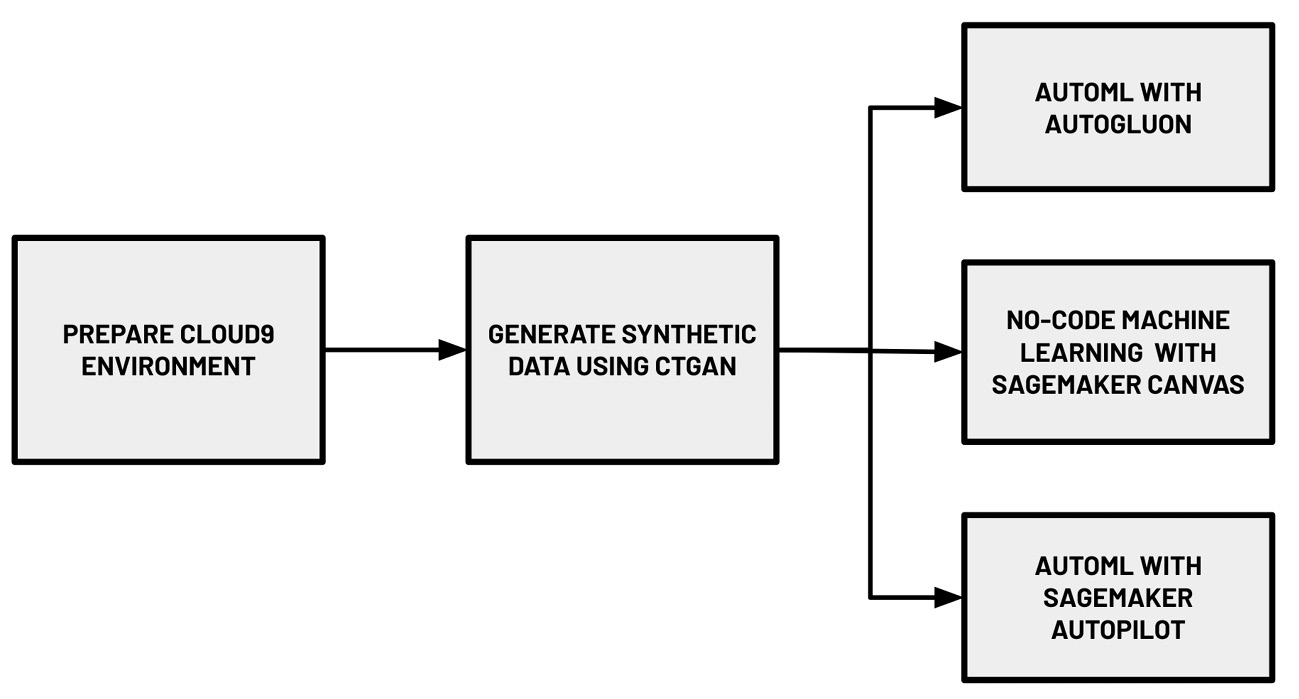
Figure 1.1 – Hands-on journey for this chapter
In this introductory chapter, we will focus on getting our feet wet by trying out different options when building an ML model on AWS. As shown in the preceding diagram, we will use a variety of AutoML services and solutions to build ML models that can help us predict if a hotel booking will be cancelled or not based on the information available. We will start by setting up a Cloud9 environment, which will help us run our code through an integrated development environment (IDE) in our browser. In this environment, we will generate a realistic synthetic dataset using a deep learning model called the Conditional Generative Adversarial Network. We will upload this dataset to Amazon S3 using the AWS CLI. Inside the Cloud9 environment, we will also install AutoGluon and run an AutoML experiment to train and generate multiple models using the synthetic dataset. Finally, we will use SageMaker Canvas and SageMaker Autopilot to run AutoML experiments using the uploaded dataset in S3. If you are wondering what these fancy terms are, keep reading as we demystify each of these in this chapter.
In this chapter, we will cover the following topics:
- What is expected from ML engineers?
- How ML engineers can get the most out of AWS
- Essential prerequisites
- Preparing the dataset
- AutoML with AutoGluon
- Getting started with SageMaker and SageMaker Canvas
- No-code machine learning with SageMaker Canvas
- AutoML with SageMaker Autopilot
In addition to getting our feet wet using key ML services, libraries, and tools to perform AutoML experiments, this introductory chapter will help us gain a better understanding of several ML and ML engineering concepts that will be relevant to the succeeding chapters of this book. With this in mind, let’s get started!