We learned in the previous chapter that many learning algorithms perform better when they are trained on standardized data. Recall that standardized data has zero mean and unit variance. An explanatory variable with zero mean is centered about the origin; its average value is zero. A feature vector has unit variance when the variances of its features are all of the same order of magnitude. If one feature's variance is orders of magnitude greater than the variances of the other features, that feature may dominate the learning algorithm and prevent it from learning from the other variables. Some learning algorithms also converge to the optimal parameter values more slowly when data is not standardized. In addition to the StandardScaler transformer we used in the previous chapter, the scale function from the preprocessing module can be used to standardize...
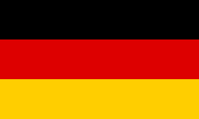
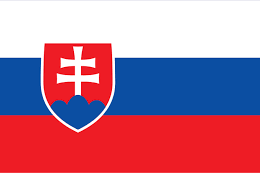
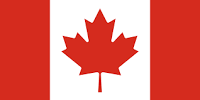
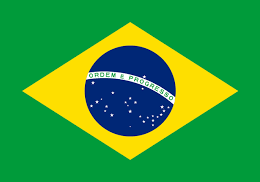

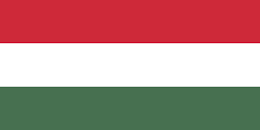
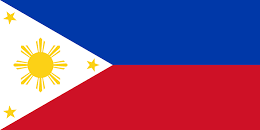
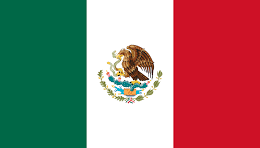
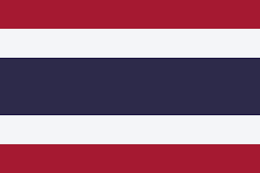
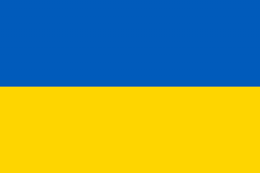
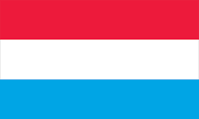
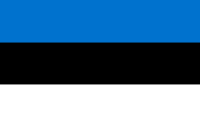
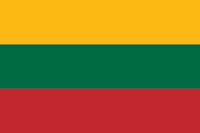
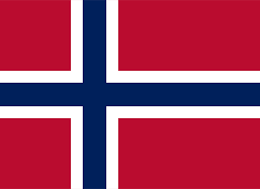
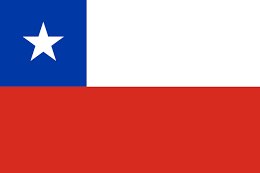
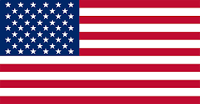
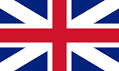
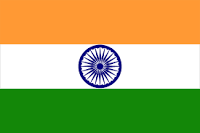
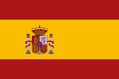
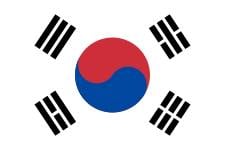
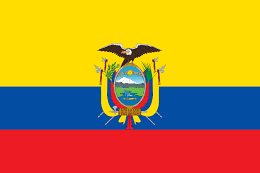
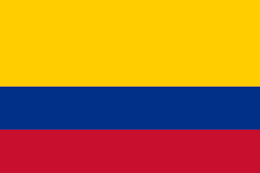
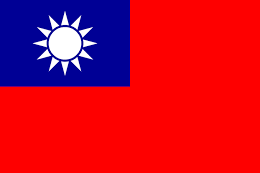
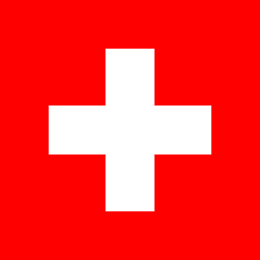
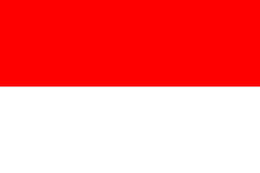
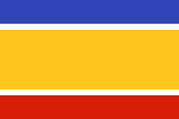
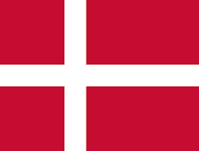
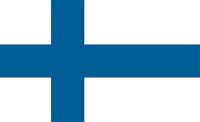
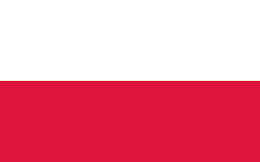
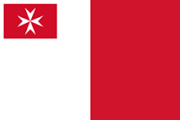
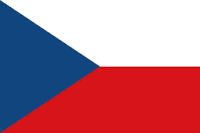
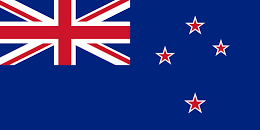
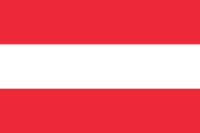
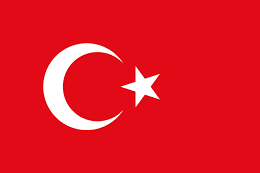
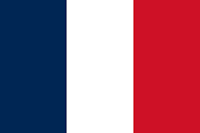
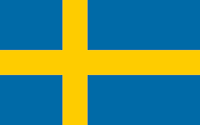
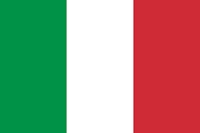
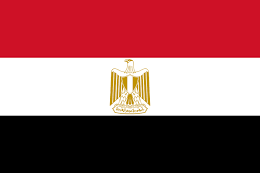
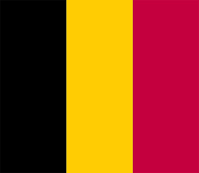
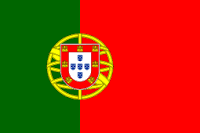
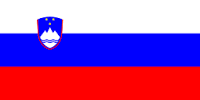
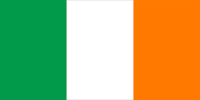
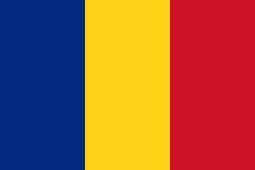
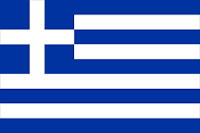
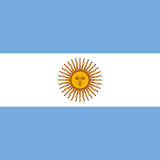
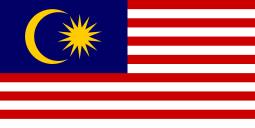
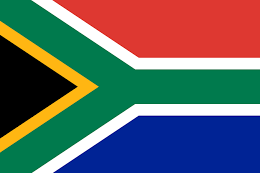
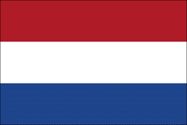
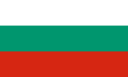
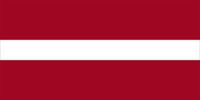
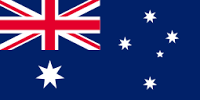
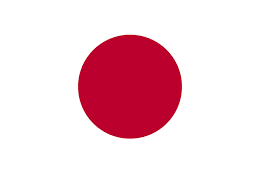
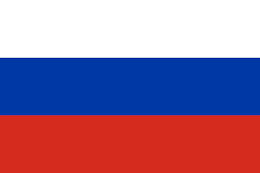