Choosing an optimal number for K and cluster validation
A big part of K-means clustering is knowing the optimal number of clusters. If we knew this number ahead of time, then that might defeat the purpose of even using unsupervised learning. So we need a way to evaluate the output of our cluster analysis.
The problem here is that because we are not performing any kind of prediction, we cannot gauge how right the algorithm is at predictions. Metrics such as accuracy and RMSE go right out of the window.
The Silhouette Coefficient
The Silhouette Coefficient is a common metric for evaluating clustering performance in situations when the true cluster assignments are not known.
A Silhouette Coefficient is calculated for each observation as follows:
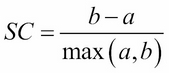
Let's look a little closer at the specific features of this formula:
- a: Mean distance to all other points in its cluster
- b: Mean distance to all other points in the next nearest cluster
It ranges from -1 (worst) to 1 (best). A global score is calculated...