This chapter covered topics that are critical to success in deep learning projects. These included the different types of evaluation metric that can be used to evaluate the model. We looked at some issues that can come up in data preparation, including if you only have a small amount of data to train on and how to create different splits in the data, that is, how to create proper train, test, and validation datasets. We looked at two important issues that can cause the model to perform poorly in production, different data distributions, and data leakage. We saw how data augmentation can be used to improve an existing model by creating artificial data and looked at tuning hyperparameters in order to improve the performance of a deep learning model. We closed the chapter by examining a use case where we simulated a problem with different data distributions/data leakage and...
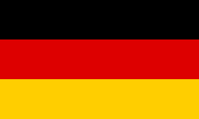
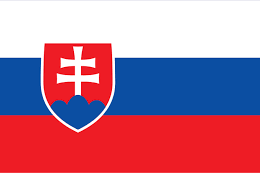
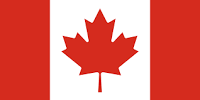
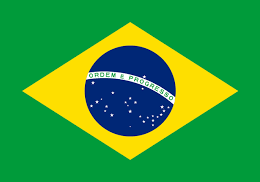

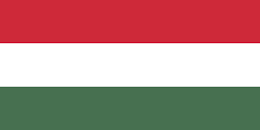
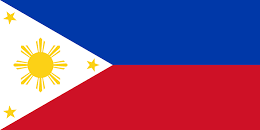
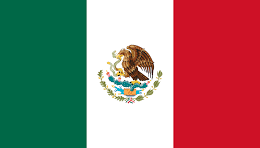
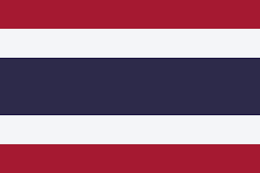
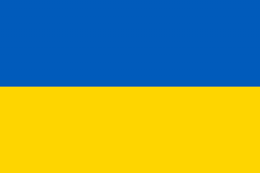
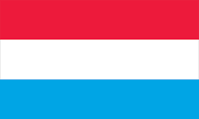
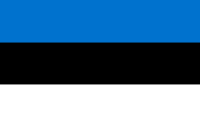
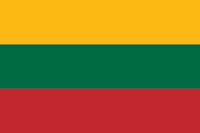
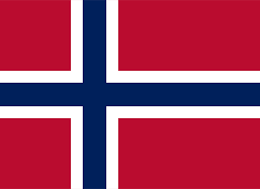
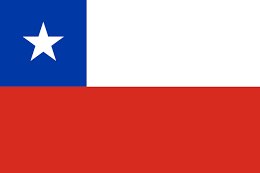
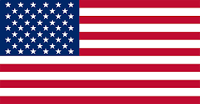
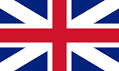
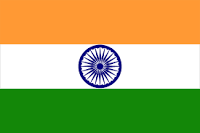
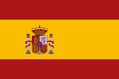
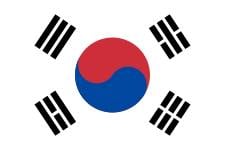
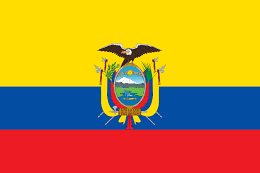
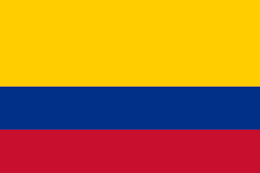
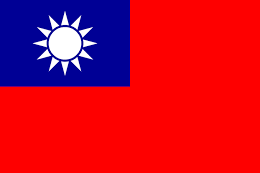
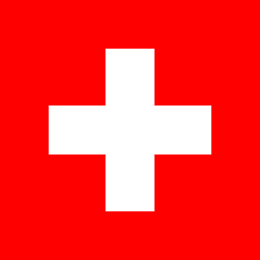
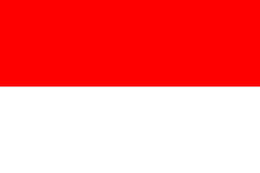
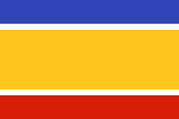
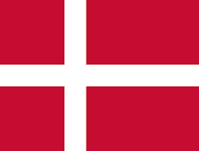
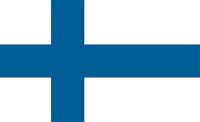
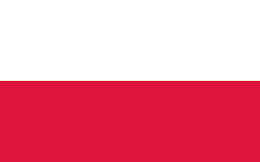
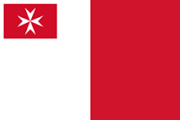
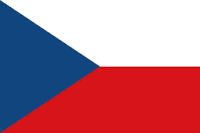
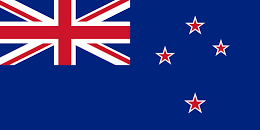
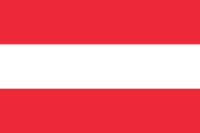
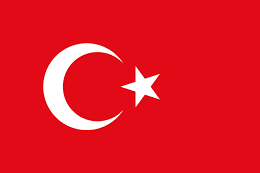
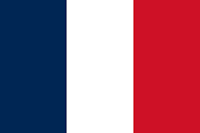
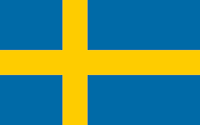
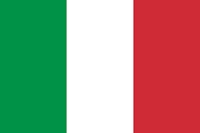
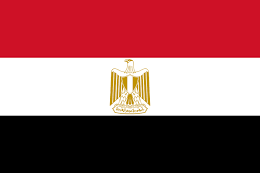
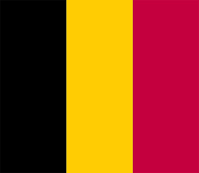
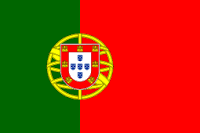
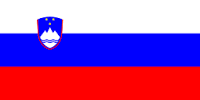
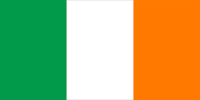
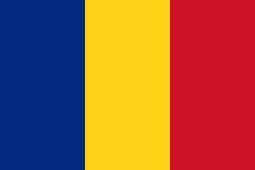
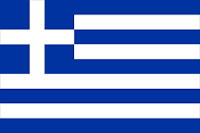
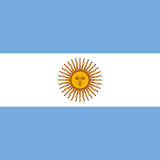
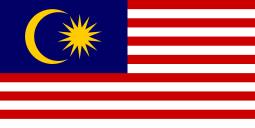
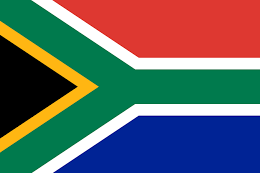
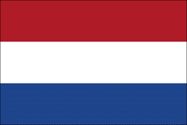
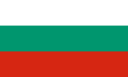
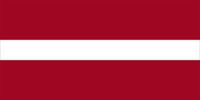
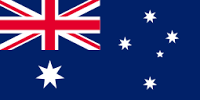
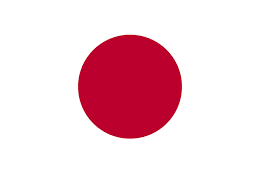
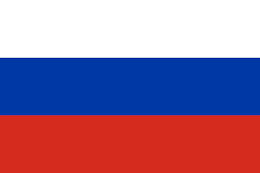