ML workflows and Spark pipelines
In this section, we provide an introduction to machine learning workflows, and also Spark pipelines, and then discuss how Spark pipeline can serve as a good tool of computing ML workflows.
After this section, readers will master these two important concepts, and be ready to program and implement Spark pipelines for machine learning workflows.
ML as a step-by-step workflow
Almost all ML projects involve cleaning data, developing features, estimating models, evaluating models, and then interpreting results, which all can be organized into some step by step workflows. These workflows are sometimes called analytical processes.
Some people even define machine learning as workflows of turning data into actionable insights, for which some people will add business understanding or problem definition into the workflows as their starting points.
In the data mining field, Cross Industry Standard Process for Data Mining (CRISP-DM) is a widely accepted workflow standard, which is still widely adopted. And many standard ML workflows are just some form of revision to the CRISP-DM workflow.
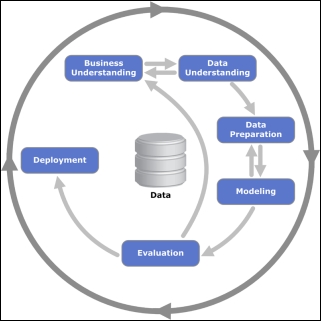
As illustrated in the above picture, for any standard CRISP-DM workflow, we need all the following 6 steps:
- Business understanding
- Data understanding
- Data preparation
- Modeling
- Evaluation
- Deployment
To which some people may add analytical approaches selection and results explanation, to make it more complete. For complicated machine learning projects, there will be some branches and feedback loops to make workflows very complex.
In other words, for some machine learning projects, after we complete model evaluation, we may go back to the step of modeling or even data preparation. After the data preparation step, we may branch out for more than two types of modeling.