This book is a collection of newly evolved deep learning models, methodologies, and implementations based on the areas of their application. In the first section of the book, you will learn about the building blocks of deep learning and the math behind neural networks (NNs). In the second section, you'll focus on convolutional neural networks (CNNs) and their advanced applications in computer vision (CV). You'll learn to apply the most popular CNN architectures in object detection and image segmentation. Finally, you'll discuss variational autoencoders and generative adversarial networks.
In the third section, you'll focus on natural language and sequence processing. You'll use NNs to extract sophisticated vector representations of words. You'll discuss various types of recurrent networks, such as long short-term memory (LSTM) and gated recurrent unit (GRU). Finally, you'll cover the attention mechanism to process sequential data without the help of recurrent networks. In the final section, you'll learn how to use graph NNs to process structured data. You'll cover meta-learning, which allows you to train an NN with fewer training samples. And finally, you'll learn how to apply deep learning in autonomous vehicles.
By the end of this book, you'll have gained mastery of the key concepts associated with deep learning and evolutionary approaches to monitoring and managing deep learning models.
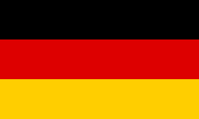
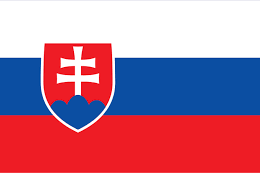
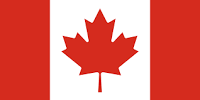
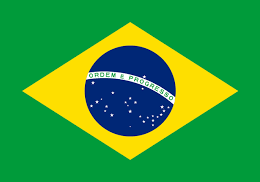

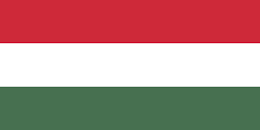
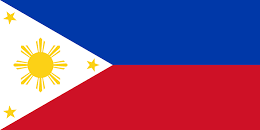
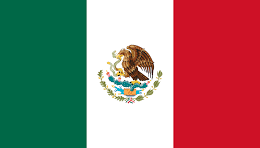
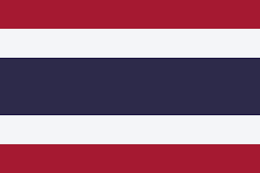
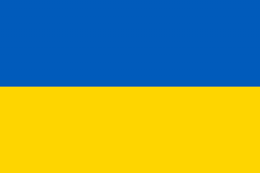
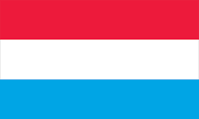
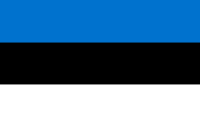
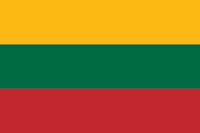
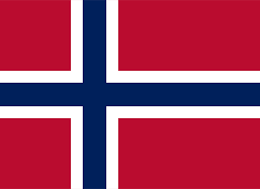
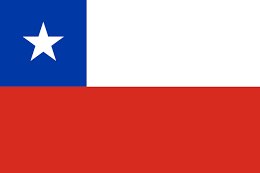
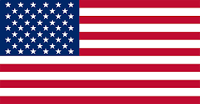
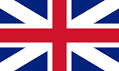
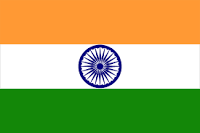
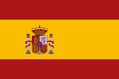
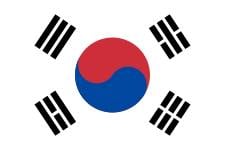
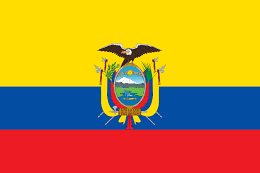
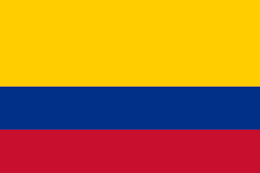
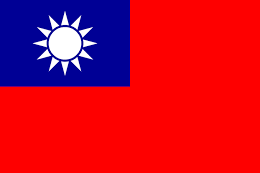
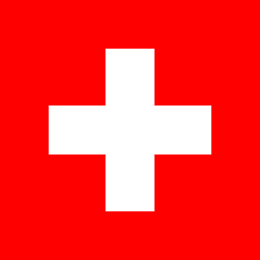
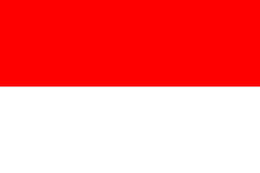
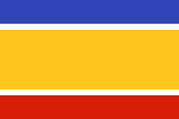
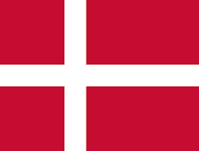
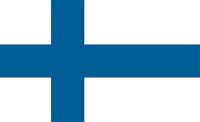
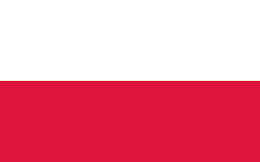
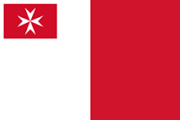
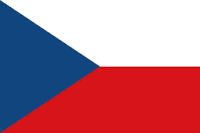
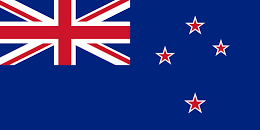
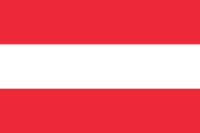
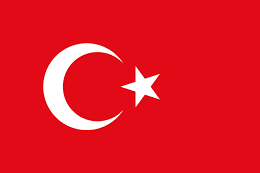
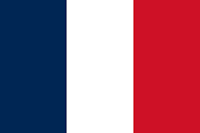
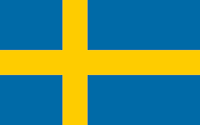
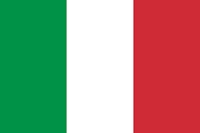
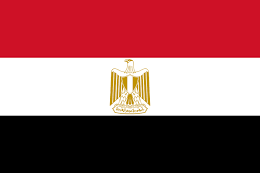
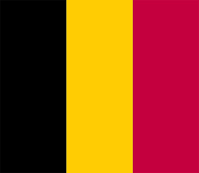
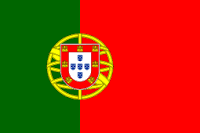
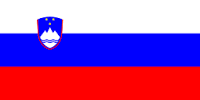
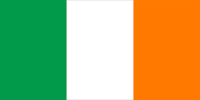
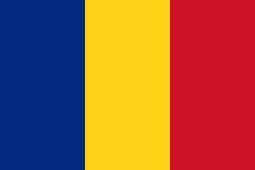
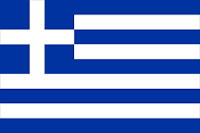
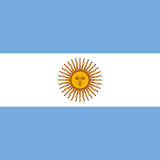
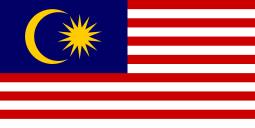
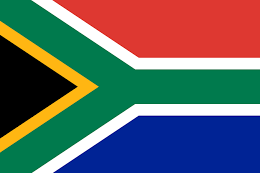
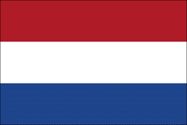
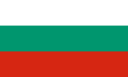
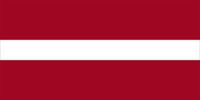
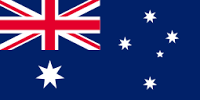
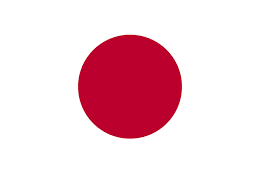
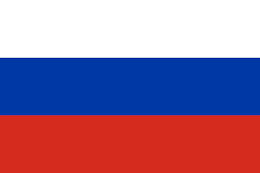