Stacking is an approach to creating ensembles; it uses a meta-estimator to combine the predictions of base estimators. Sometimes called blending, stacking adds a second supervised learning problem: the meta-estimator must be trained to use the predictions of the base estimators to predict the value of the response variable. To train a stacked ensemble, first use the training set to train the base estimators. Unlike bagging and boosting, stacking can use different types of base estimators; a random forest could be combined with a logistic regression classifier, for example. The base estimators' predictions and the ground truth are then used as the training set for the meta-estimator. The meta-estimator can learn to combine the base estimators' predictions in more complex ways than voting or averaging. scikit-learn does not implement a stacking meta...
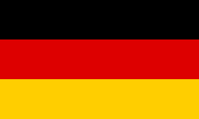
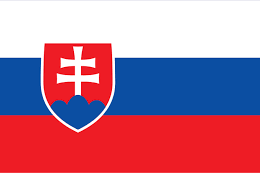
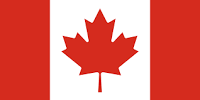
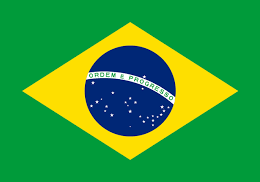

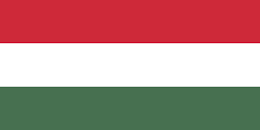
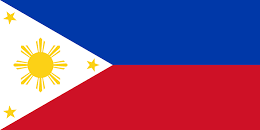
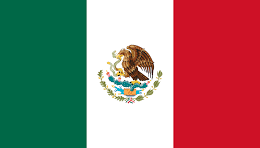
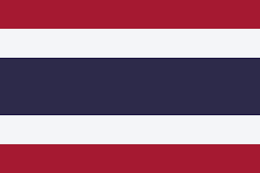
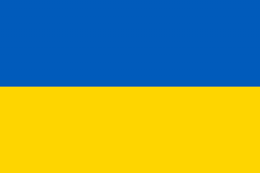
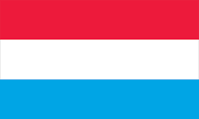
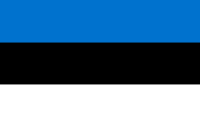
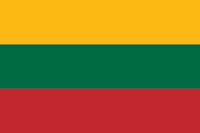
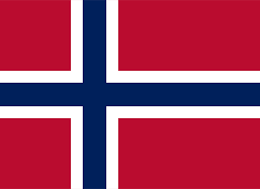
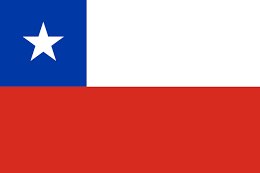
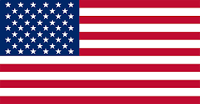
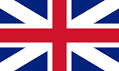
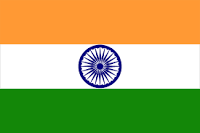
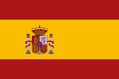
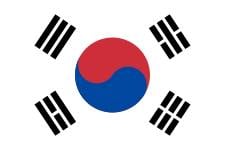
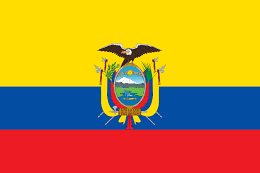
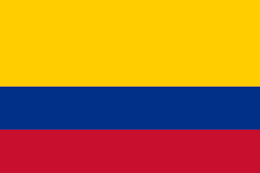
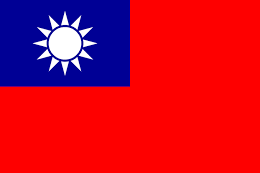
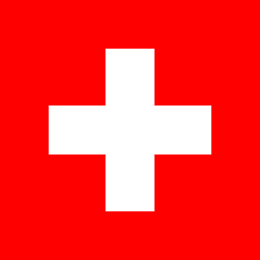
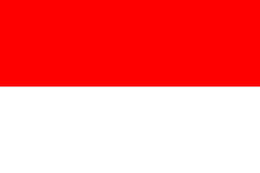
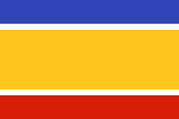
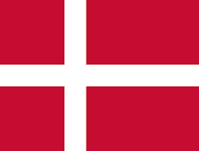
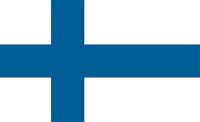
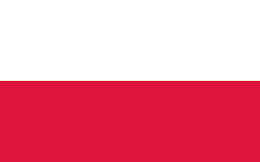
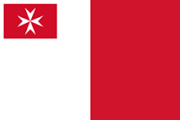
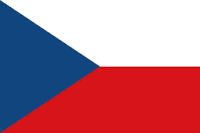
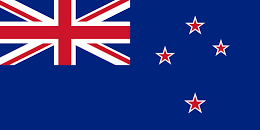
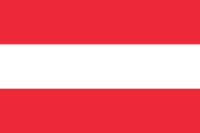
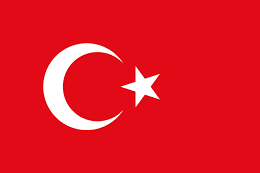
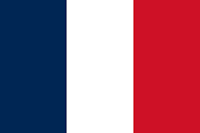
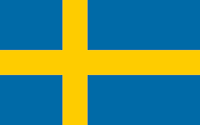
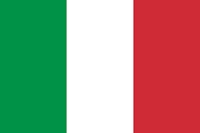
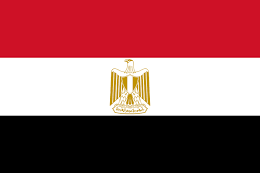
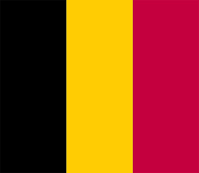
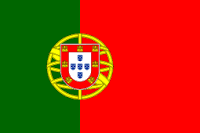
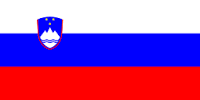
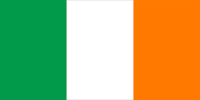
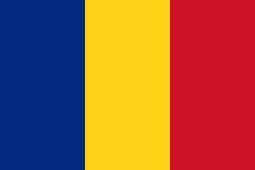
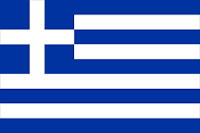
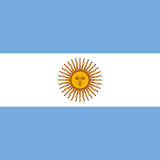
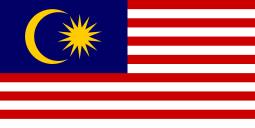
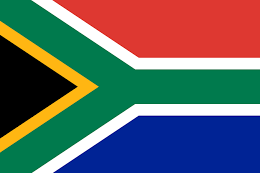
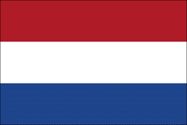
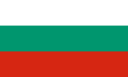
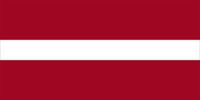
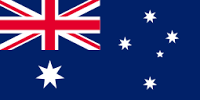
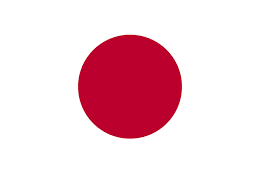
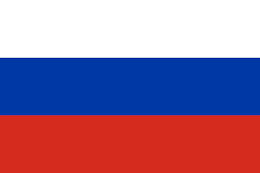