In this chapter, we will discuss a technique for reducing the dimensions of data called principal component analysis (PCA). Dimensionality reduction is motivated by several problems. Firstly, it can be used to mitigate problems caused by the curse of dimensionality. Secondly, dimensionality reduction can be used to compress data while minimizing the amount of information that is lost. Thirdly, understanding the structure of data with hundreds of dimensions can be difficult; data with only two or three dimensions can be visualized easily. We will use PCA to visualize a high-dimensional dataset in two dimensions and to build a face recognition system.
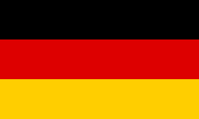
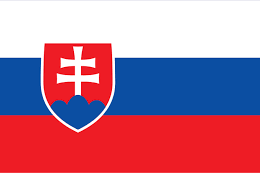
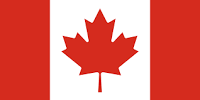
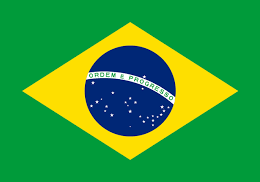

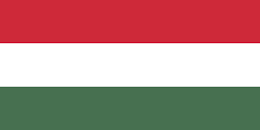
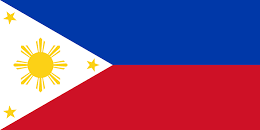
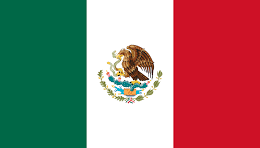
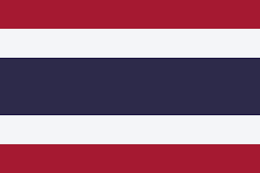
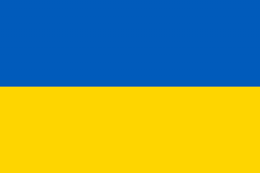
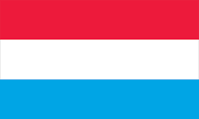
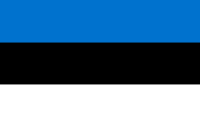
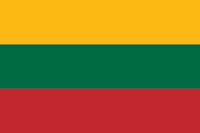
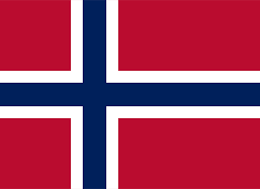
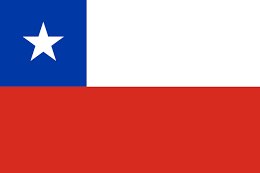
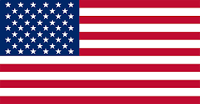
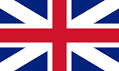
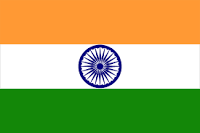
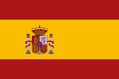
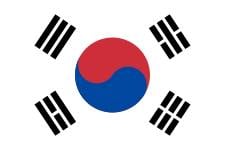
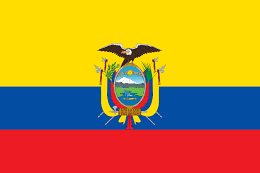
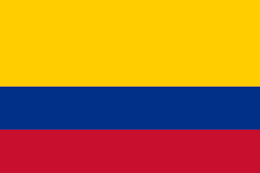
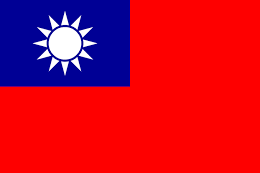
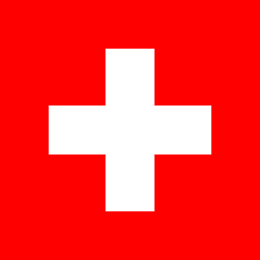
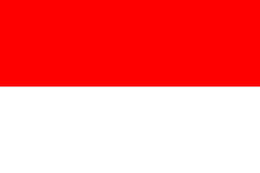
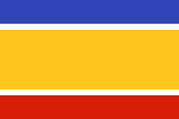
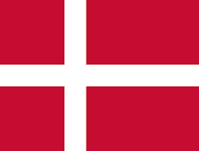
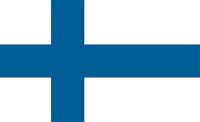
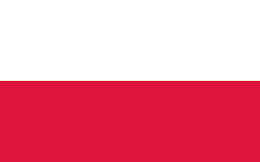
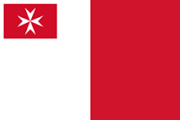
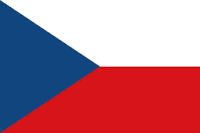
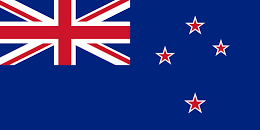
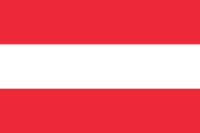
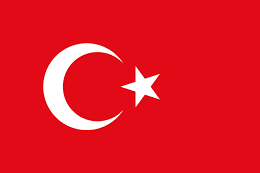
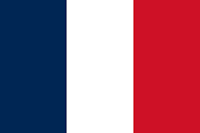
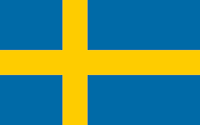
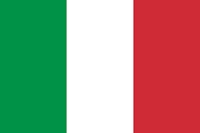
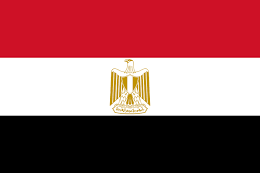
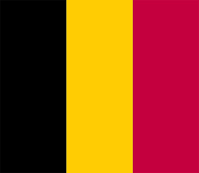
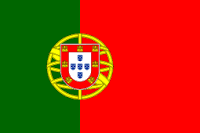
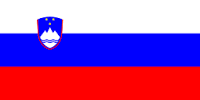
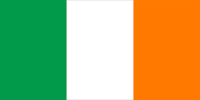
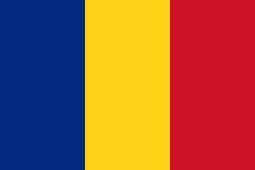
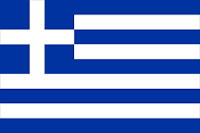
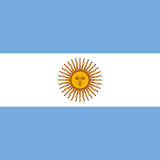
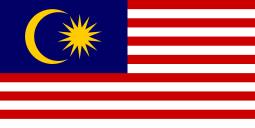
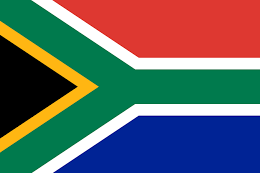
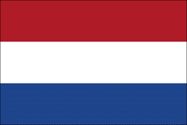
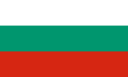
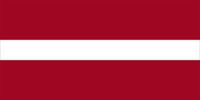
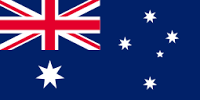
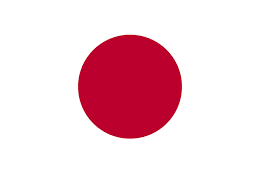
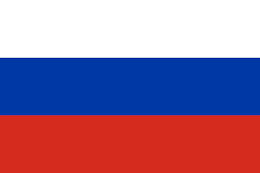