Data quality challenges
In this section, we will discuss the challenges of data quality, which are not unique to Web3 but relevant to all professionals who make decisions based on data. Data quality challenges range from access to incomplete, inaccurate, or inconsistent data to matters of data security, privacy, or governance. However, one of the most important challenges that a Web3 data analyst will face is the reliability of sources.
For instance, the market cap is the result of a simple multiplication of two data sources: the blockchain data that informs the total supply of tokens in circulation and the market price. However, the result of such multiplication varies depending on the source. Let’s take an example of the market cap for USDT. In one source, the following information appears:
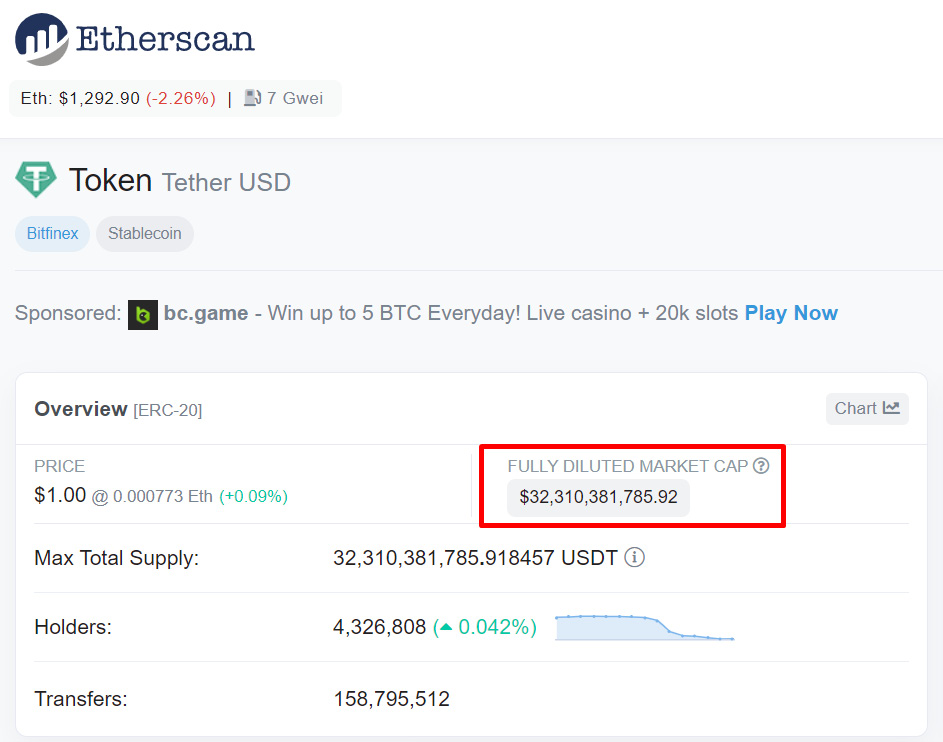
Figure 1.4 – USDT market cap information (source: https://etherscan.io/token/0xdac17f958d2ee523a2206206994597c13d831ec7)
On the CoinMarketCap website, for the same token, the fully diluted market cap is $70,158,658,274 (https://coinmarketcap.com/currencies/tether/).
As we see from the example, the same concept is shown differently depending on the source we review. So, how do we choose when we have multiple sources of information?
The most trustworthy and comprehensive source of truth regarding blockchain activity is the full copy of a node. Accessing a node ensures that we will always have access to the latest version of the blockchain. Some services index the blockchain to facilitate access and querying, such as Google BigQuery, Covalent, or Dune, continuously updating their copies. These copies are controlled and centralized.
When it comes to prices, there are numerous sources. A common approach to sourcing prices is connecting to an online marketplace for cryptocurrencies, commonly known as exchanges, such as Binance or Kraken, to extract their market prices. However, commercialization in these markets can be halted for various reasons. For example, during the well-known Terra USD (TUSD) de-peg incident, when the stablecoin lost its 1:1 peg to the US dollar, many exchanges ceased commercialization, citing consumer protection concerns. If our workflow relies on such data, it can be disrupted or show inaccurate old prices. To solve this issue, it is advisable to source prices from sources that average the prices from multiple exchanges, providing more robust information.
At this stage, it is crucial to understand what constitutes quality for our company. Do we prioritize fast and readily available information updated by the second, or do we value highly precise information with relatively slower access? While it may not be necessary to consider this for every project, deciding on certain sources and standardizing processes will save us time in the future.
Once we have determined the quality of the information we will consume, we need to agree on the concepts we want to analyze.