For the more mathematically oriented, you must be wondering how exactly we descend our gradient iteratively. Well, as you know, we start by initializing random weights to our model, feed in some data, compute dot products, and pass it through our activation function along with our bias to get a predicted output. We use this predicted output and the actual output to estimate the errors in our model's representations, using the loss function. Now here comes the calculus. What we can do now is differentiate our loss function, J(θ), with respect to the weights of our model (θ). This process essentially lets us compare how changes in our model's weights affect the changes in our model's loss. The result of this differentiation gives us the gradient of our J(θ) function at the current model weight (θ) along with the direction of...
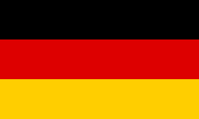
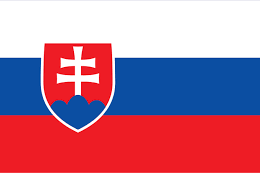
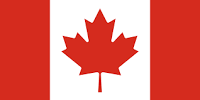
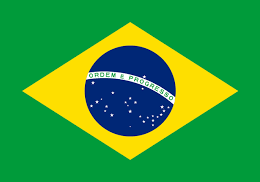

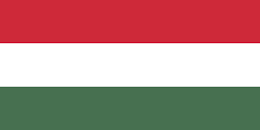
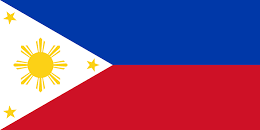
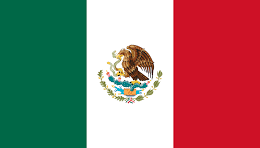
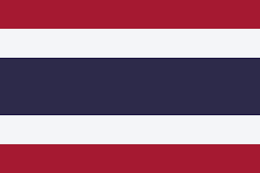
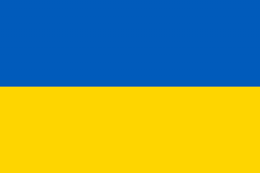
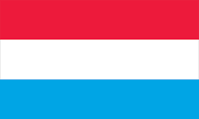
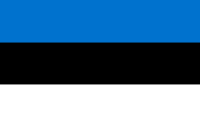
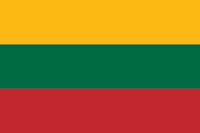
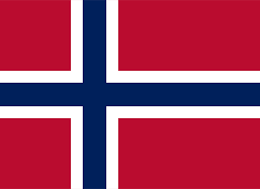
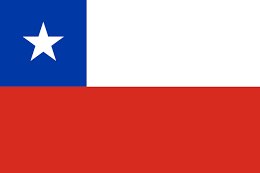
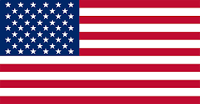
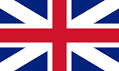
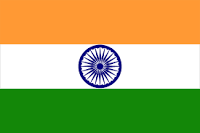
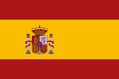
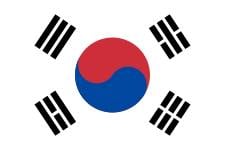
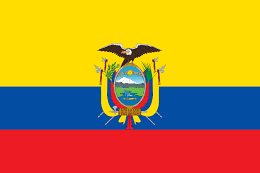
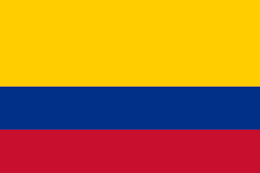
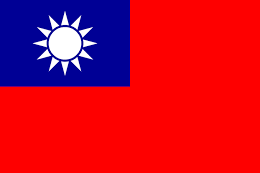
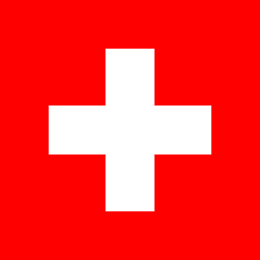
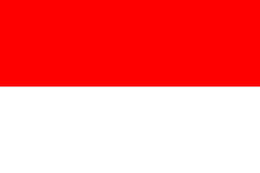
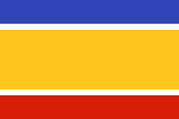
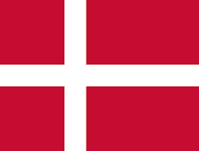
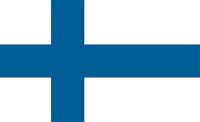
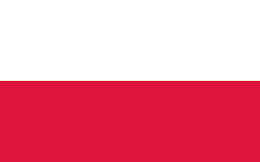
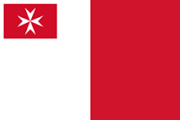
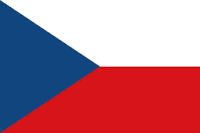
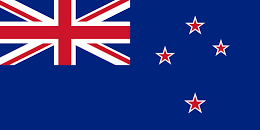
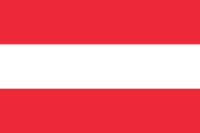
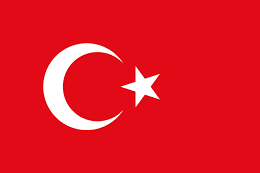
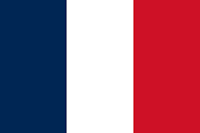
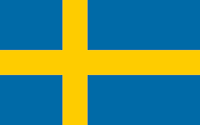
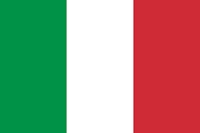
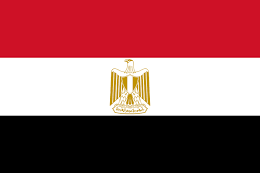
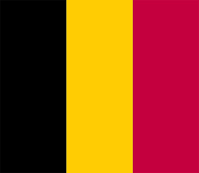
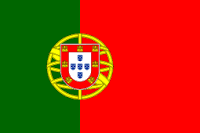
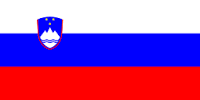
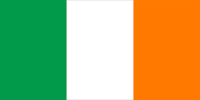
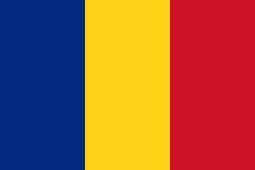
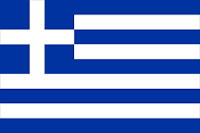
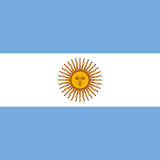
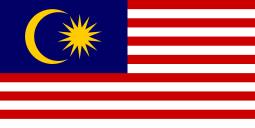
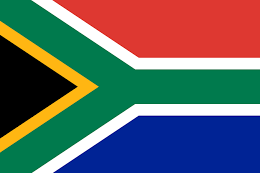
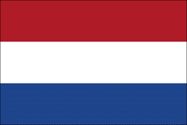
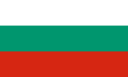
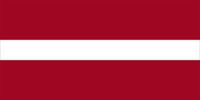
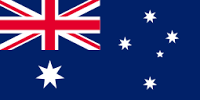
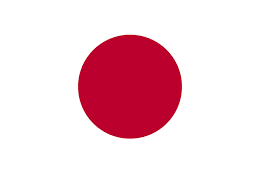
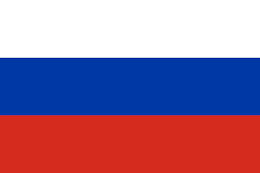