So far, our focus has been on discrete actions and episodic environments, where the agent often learns to solve a puzzle or accomplish some task. The best examples of such environments are GridWorld, and, of course, the Hallway/VisualHallway samples, where the agent discretely chosses actions such as up, left, down, or right, and, using those actions, has to navigate to some goal. While these are great environments to play with and learn the basic concepts of RL, they can be quite tedious environments to learn from, since results are not often automatic and require extensive exploration. However, in marathon RL environments, the agent is always learning by receiving rewards in the form of control feedback. In fact, this form of RL is analogus to control systems for robotics and simulations. Since these environments are rich with rewards in the form of feedback, they...
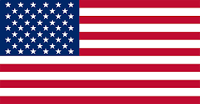
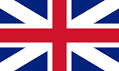
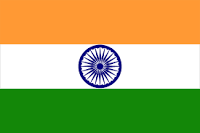
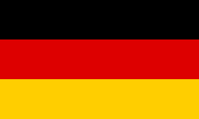
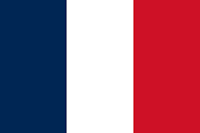
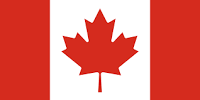
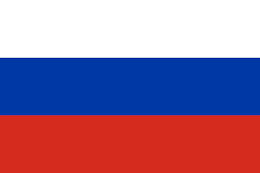
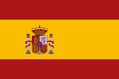
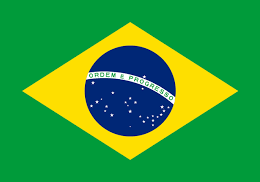
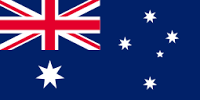

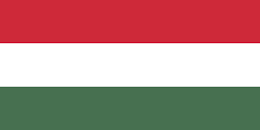
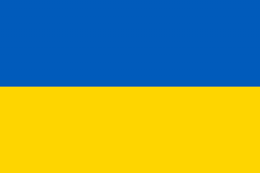
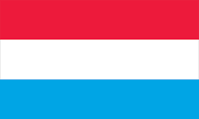
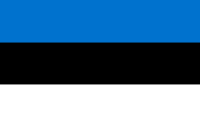
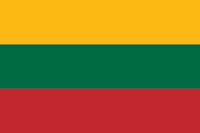
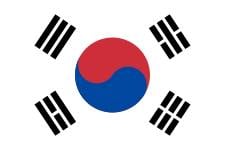
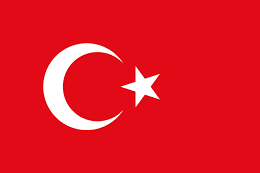
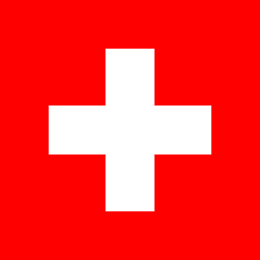
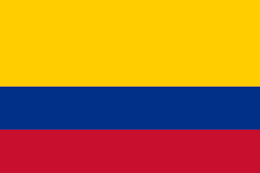
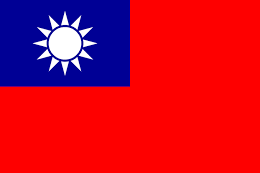
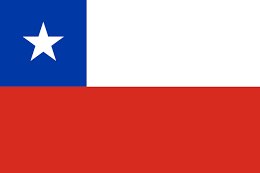
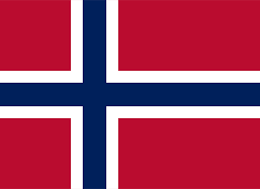
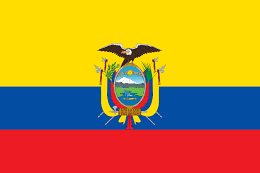
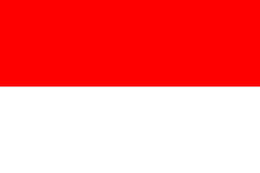
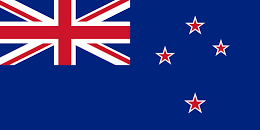
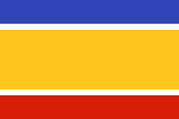
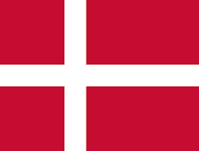
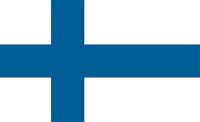
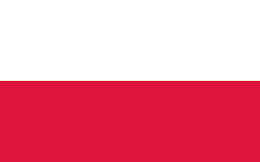
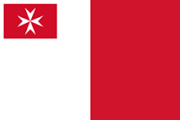
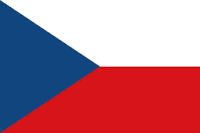
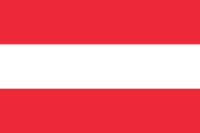
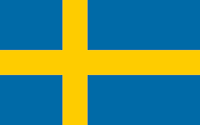
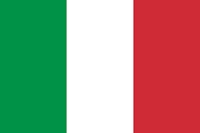
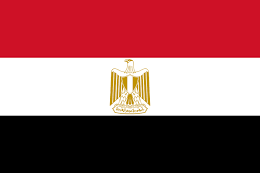
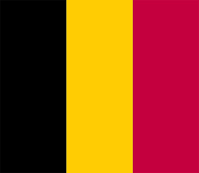
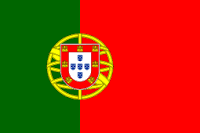
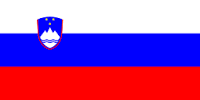
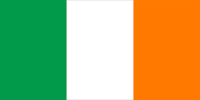
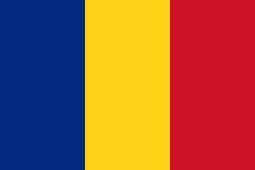
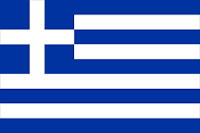
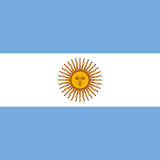
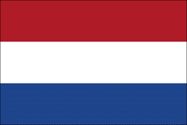
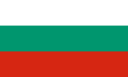
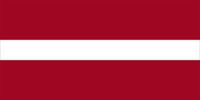
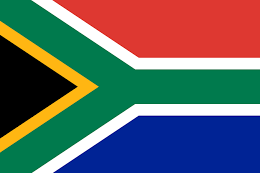
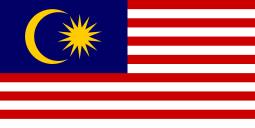
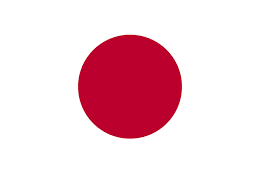
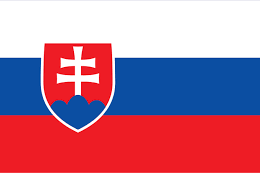
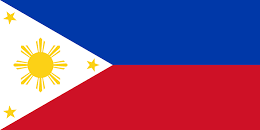
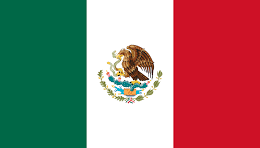
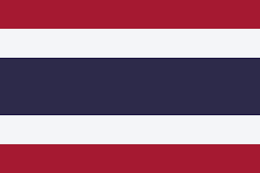