- Reward refers to the current point value of taking an action, and value refers to the overall utility of an agent's future actions as a result of taking that action.
- A hyperparameter value is not determined by anything in the model itself and has to be set externally. Some kinds of hyperparameters might be the depth of or number of leaf nodes on a decision tree model.
- Because we don't want a learning agent to keep taking the same high-valued actions over and over if there are higher-valued actions available, an exploration strategy has it take a random action with the goal of discovering actions that might be higher-valued than the ones we've already seen. It could be taking a random action as a result of an exploration strategy.
- In a situation where we would value future rewards more heavily at the beginning...
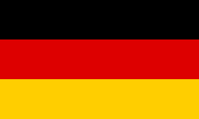
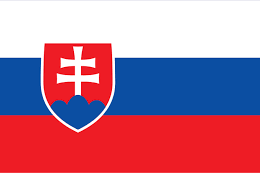
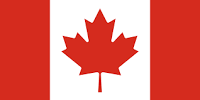
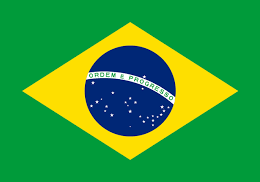

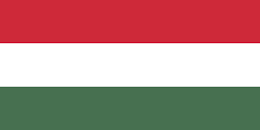
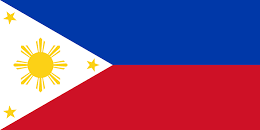
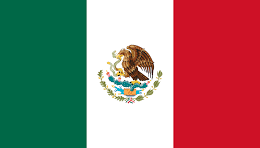
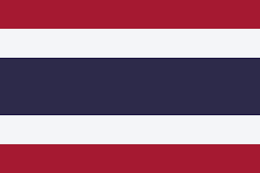
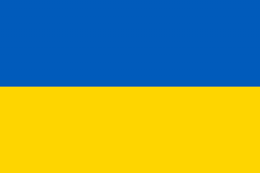
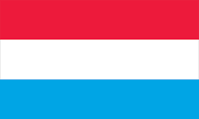
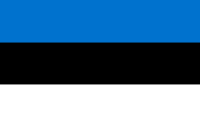
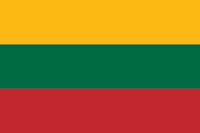
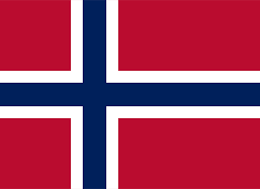
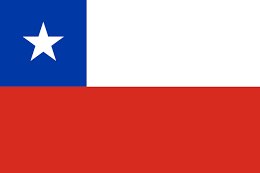
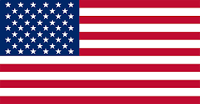
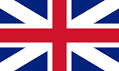
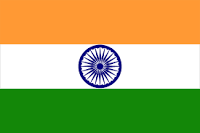
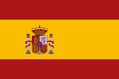
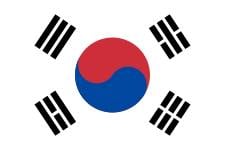
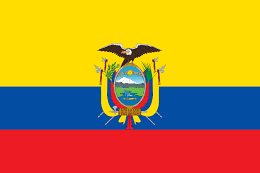
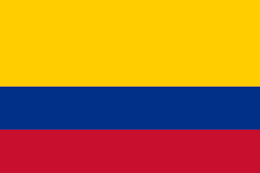
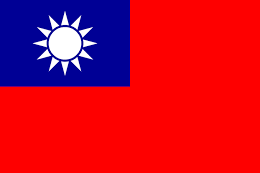
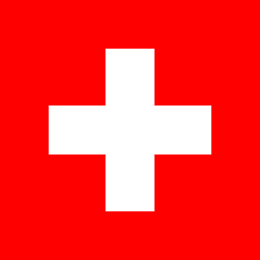
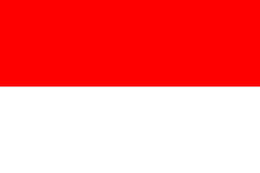
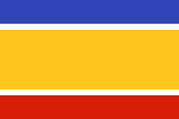
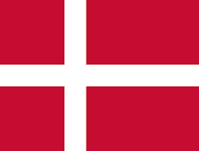
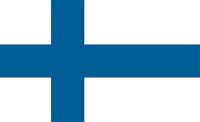
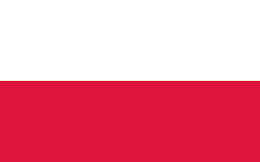
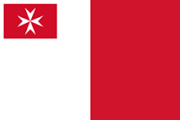
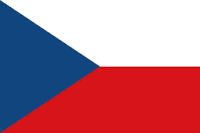
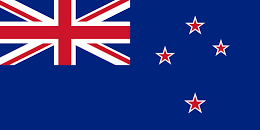
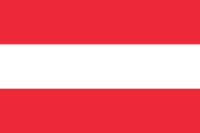
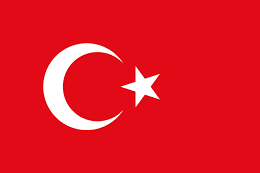
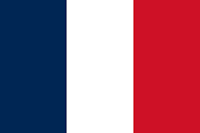
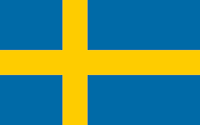
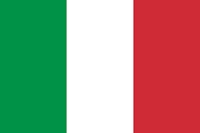
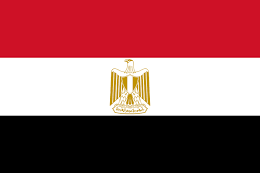
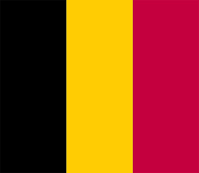
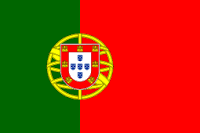
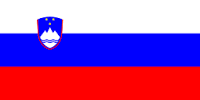
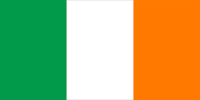
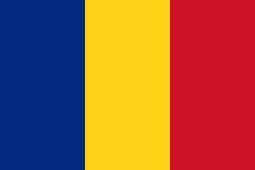
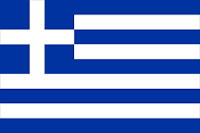
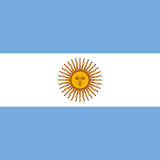
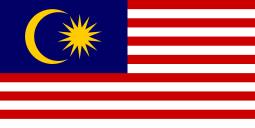
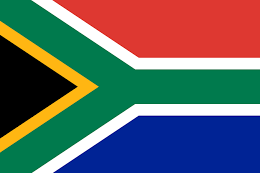
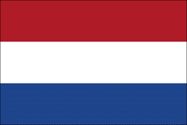
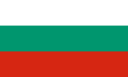
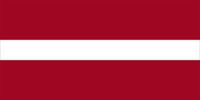
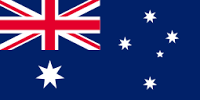
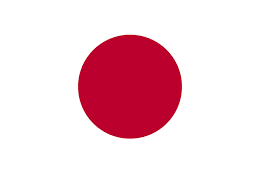
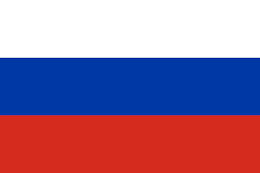