Augmenting LLMs with RAG
Coined for the first time in a 2020 paper, Lewis, Patrick et al. (2005). “Retrieval-Augmented Generation for Knowledge-Intensive NLP Tasks”. arXiv:2005.11401 [cs.CL] (https://arxiv.org/abs/2005.11401), published by several researchers from Meta, RAG is a technique that combines the powers of retrieval methods and generative models to answer user questions. The idea is to first retrieve relevant information from an indexed data source containing proprietary knowledge and then use that retrieved information to generate a more informed, context-rich response using a generative model (Figure 1.5):
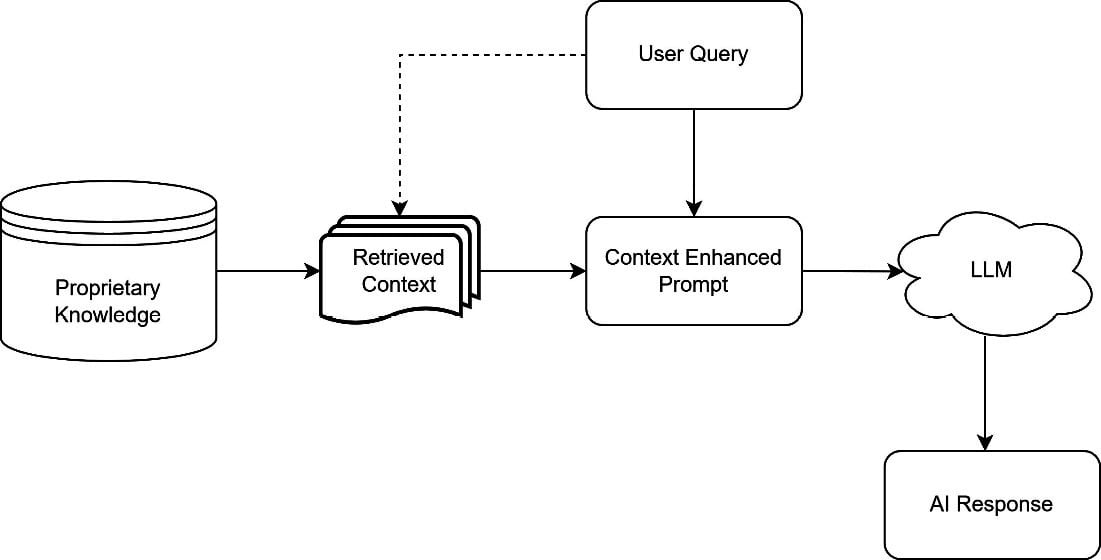
Figure 1.5 – A RAG model
Let’s have a look at what this means in practice:
- Much better fact retention: One of the advantages of using RAG is its ability to pull from specific data sources, which can improve fact retention. Instead of relying solely on the generative model’s own knowledge –...