Data Cleaning
Your next step is cleaning the data. You may well have made some entry errors and some of your data may not be useable. You need to find such instances and correct them. The alternative is that your data may not be fit for purpose and may mislead you in your pursuit of the answers to your questions.
Even after you've corrected the obvious entry errors, there may be other types of errors in your data that are harder to find.
Check That Your Data Is Sensible
Just because your dataset is clean, it doesn't mean that it is correct – real life follows rules, and your data must follow them, too. There are limits on the heights of participants in your study, so check that all data fits within reasonable limits. Calculate the minimum, maximum, and mean values of variables to see whether all values are sensible.
Sometimes, putting together two or more pieces of data can reveal errors that can otherwise be difficult to detect. Does the difference between date of birth and date of diagnosis give you a negative number? Is your patient over 300 years old?
Figure 1.2 gives you a list of the most useful measures that will help you discover errors in your data and find out whether real-life rules have been followed.
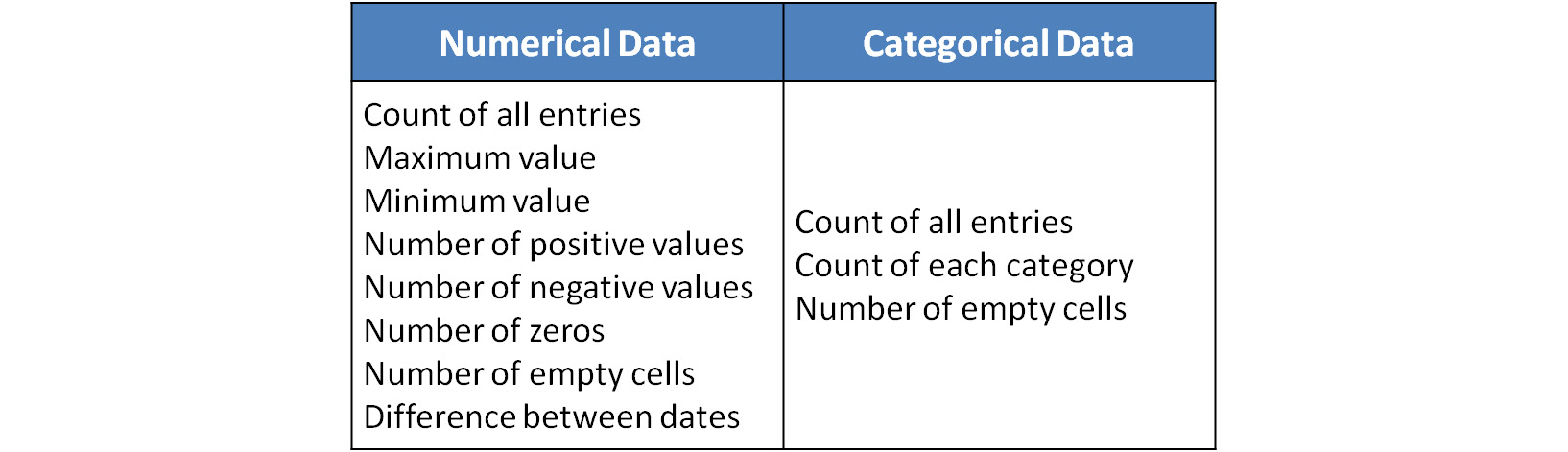
Figure 1.2: Essential descriptive statistics
Check That Your Variables Are Sensible
Once you have a perfectly clean dataset it is relatively easy to compare variables with each other to find out whether there is a relationship between them (the subject of this book). But just because you can, it doesn't mean that you should. If there is no good reason why there should be a relationship between sales of ice cream and haemorrhoid cream, then you should consider expelling one of or both of those variables from the dataset. If you've collected your own data from original sources, then you'll have considered beforehand what data is sensible to collect (you have, haven't you?), but if your dataset is a pastiche of two or more datasets, then you might find strange combinations of variables.
You should check your variables before doing any analyses and consider whether it is sensible to make these comparisons.
So, now you have collected your data, cleaned your data, and checked that your data is sensible and fit for purpose. In the next chapter, we'll go through the basics of data classification and introduce the four types of data.