Let's begin modeling by using our dataset. We're going to examine the effect that the ZIP code and the number of bedrooms have on the rental price. We'll use two packages here: the first, statsmodels, we introduced in Chapter 1, The Python Machine Learning Ecosystem, but the second, patsy, https://patsy.readthedocs.org/en/latest/index.html, is a package that makes working with statsmodels easier. Patsy allows you to use R-style formulas when running a regression. Let's do that now:
import patsy import statsmodels.api as sm f = 'rent ~ zip + beds' y, X = patsy.dmatrices(f, zdf, return_type='dataframe') results = sm.OLS(y, X).fit() results.summary()
The preceding code generates the following output:
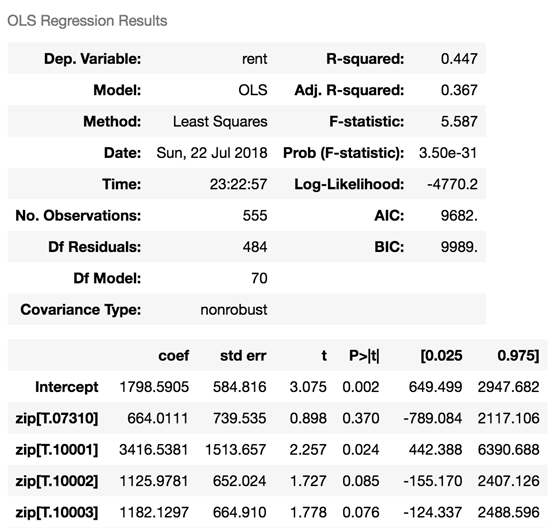
Note that the preceding output is truncated.
With those few lines of code, we have just run our first machine...