In the application examples that we've provided so far in this book, after we developed a classification or prediction deep network model, we carried out visualizations to view the overall performance of the models. These assessments are done using training and test data. The main idea behind such an assessment is to obtain an overall or global understanding of the model's performance. However, there are situations where we want to obtain a deeper understanding and also interpretations for a specific prediction. For example, we may be interested in understanding the main features or variables that have influenced a specific prediction in the test data. Such "local" interpretations are the focus of a package called Local Interpretable Model-Agnostic Explanations, or LIME. LIME can help provide deeper insights into each...
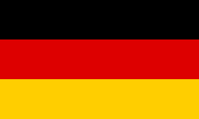
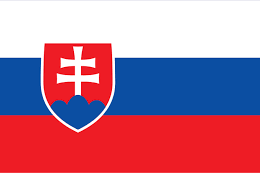
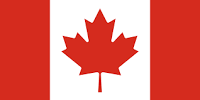
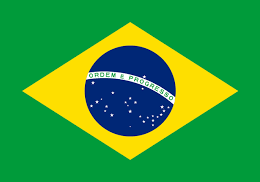

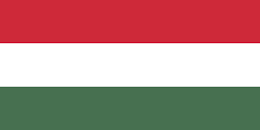
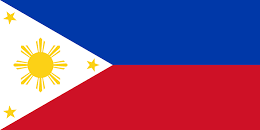
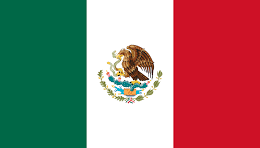
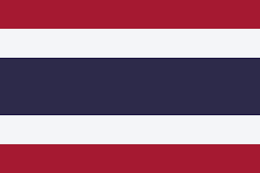
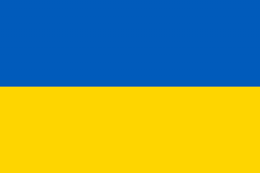
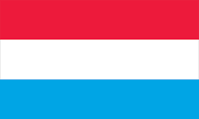
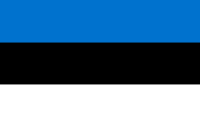
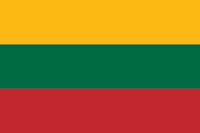
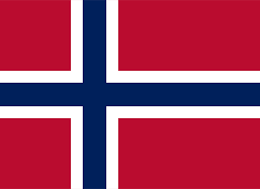
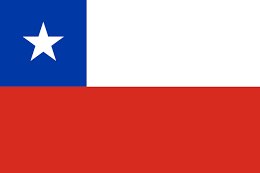
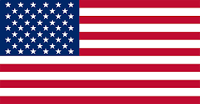
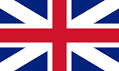
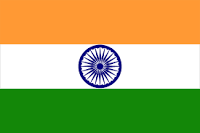
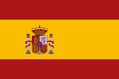
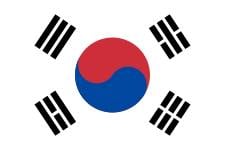
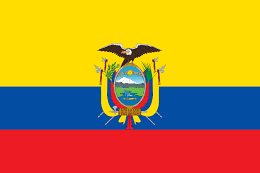
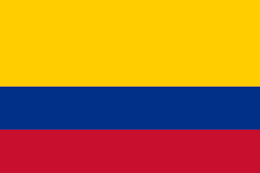
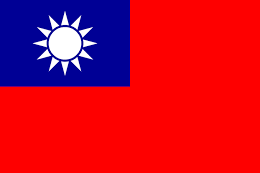
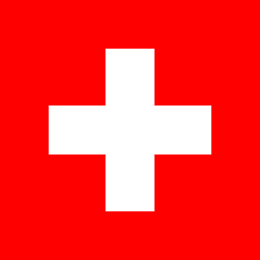
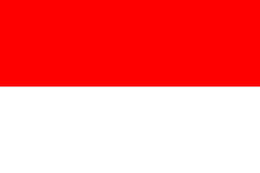
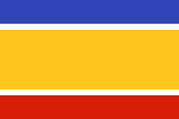
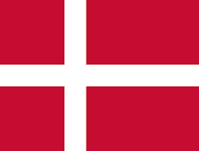
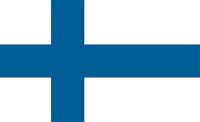
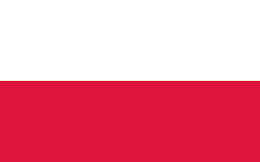
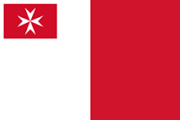
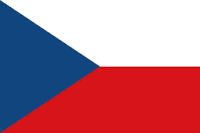
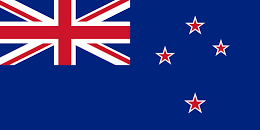
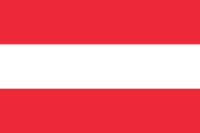
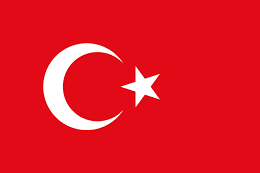
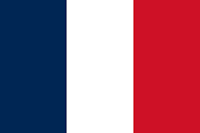
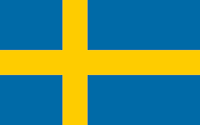
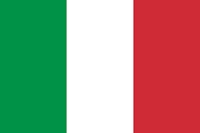
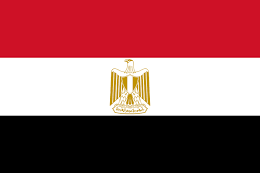
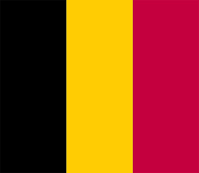
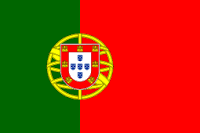
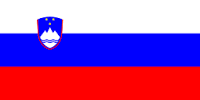
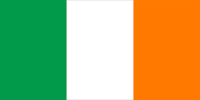
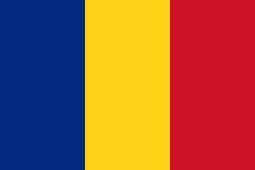
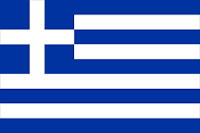
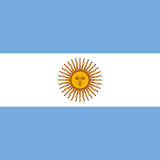
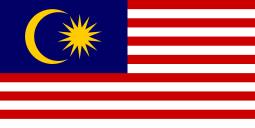
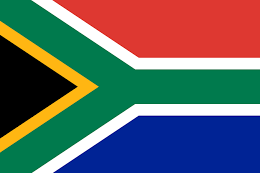
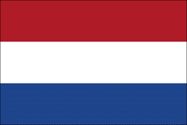
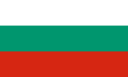
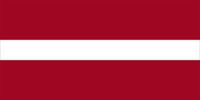
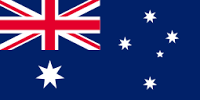
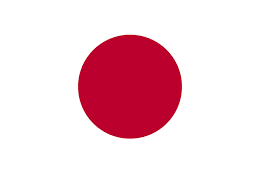
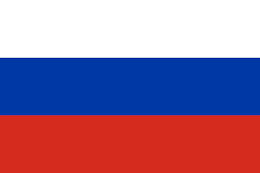