So far, we have explored models where we have different fixed levels for each effect. This makes a lot of sense when we have a set of possible levels for an effect that we control and are interested in measuring. It also makes sense when we have a blocking effect that has a finite (and small) set values (for example, the sex or occupation of a person). In some cases, we will have a huge amount of levels that will be generally unimportant, for example, if we want to measure whether a drug is effective, and we are dealing with multiple observations per person, we want to add a blocking effect for a person. In these cases, we are not interested in the effect per se, but we certainly want to use it as a control variable for our model. A model that uses proper blocks, will be more efficient: think of ANOVA as a method of attributing variability to factors. If we have...
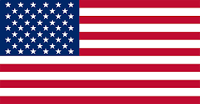
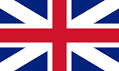
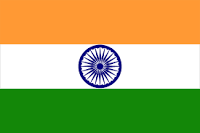
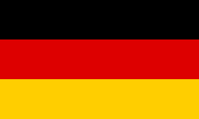
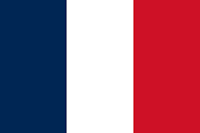
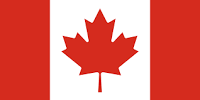
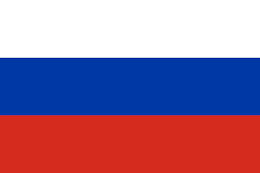
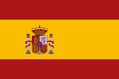
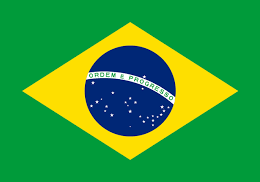
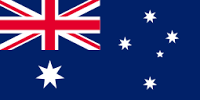

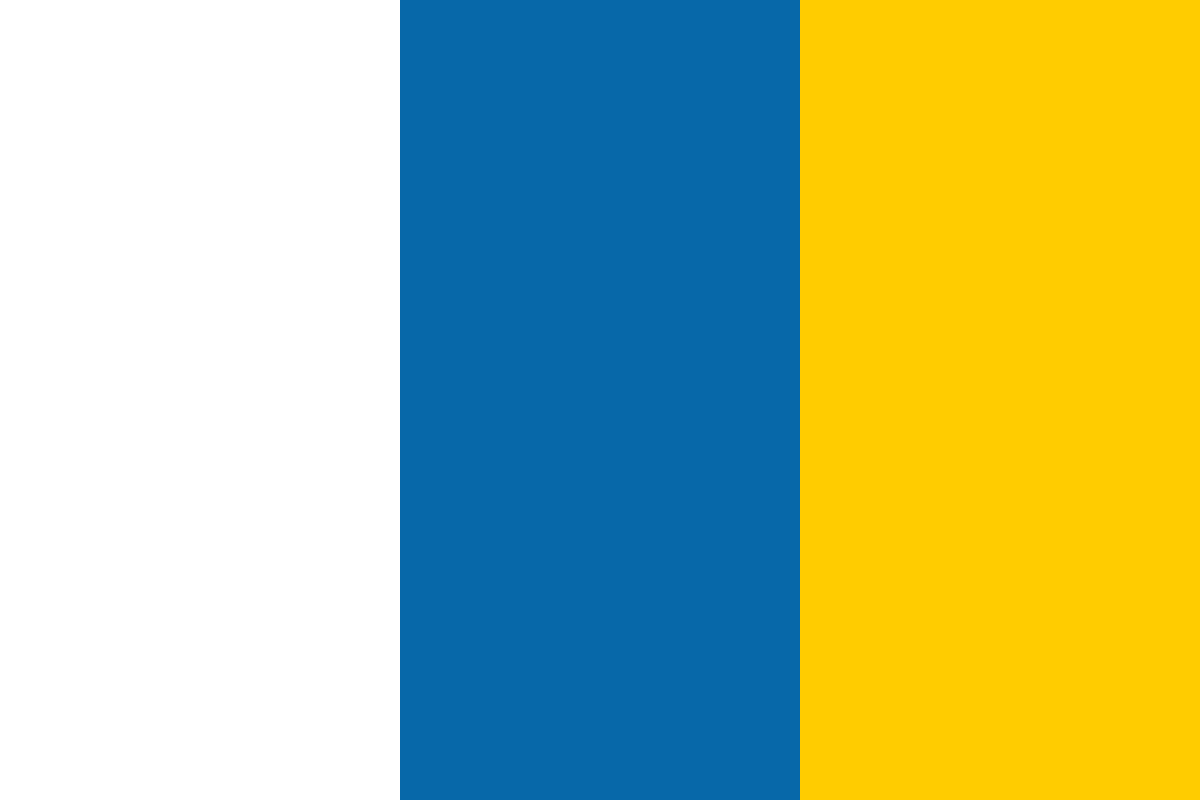
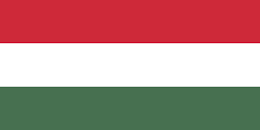
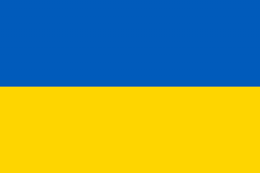
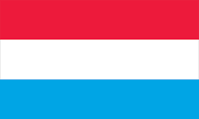
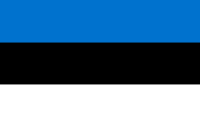
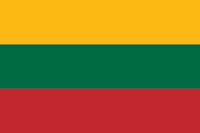
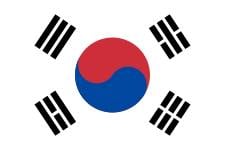
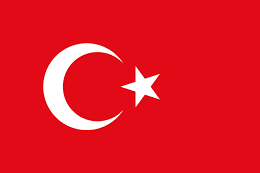
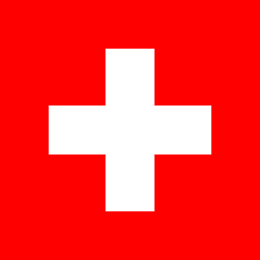
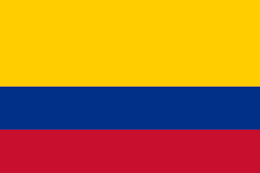
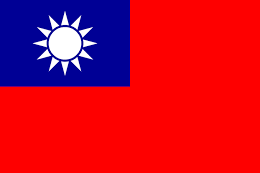
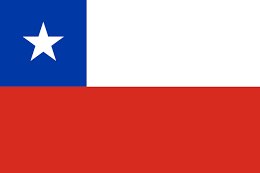
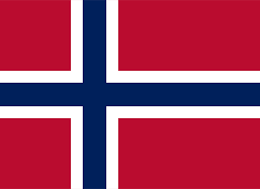
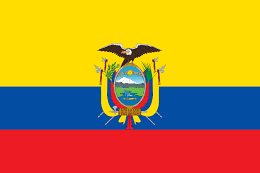
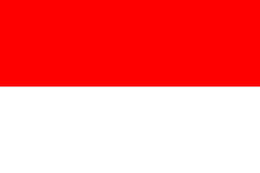
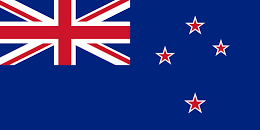
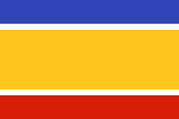
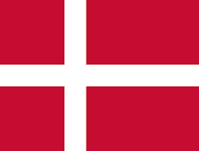
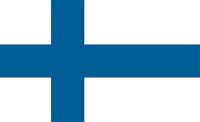
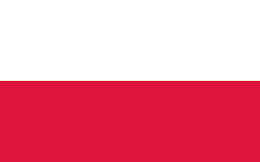
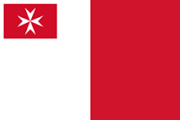
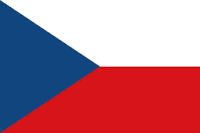
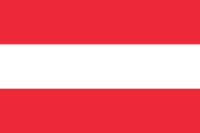
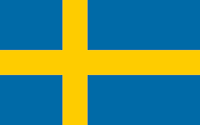
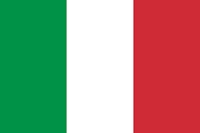
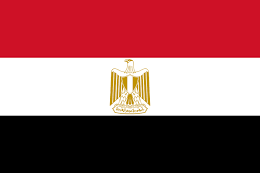
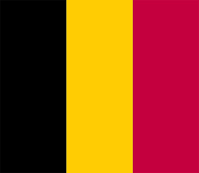
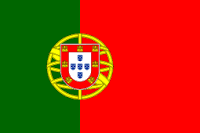
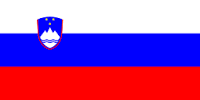
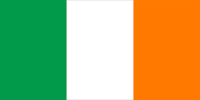
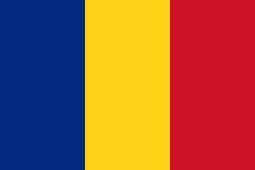
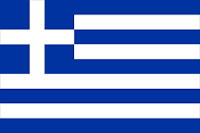
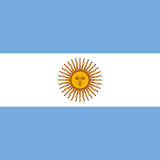
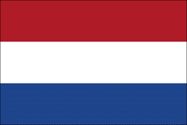
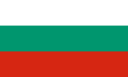
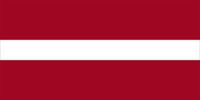
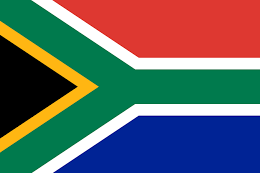
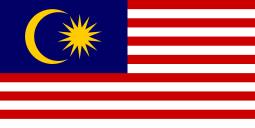
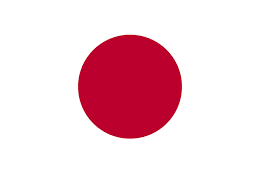
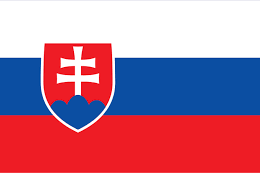
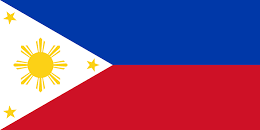
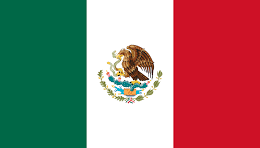
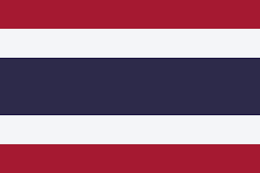