Efficiently teaching neural networks so that they minimize the loss over training data is, however, not enough. We also want these networks to perform well once applied to new images. We do not want them to overfit the training set (as mentioned in Chapter 1, Computer Vision and Neural Networks). For our networks to generalize well, we mentioned that rich training sets (with enough variability to cover possible testing scenarios) and well-defined architectures (neither too shallow to avoid underfitting, nor too complex to prevent overfitting) are key. However, other methods have been developed over the years for regularization; for example, the process of refining the optimization phase to avoid overfitting.
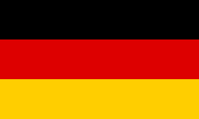
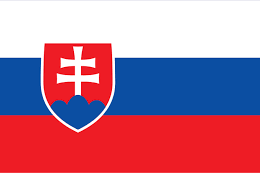
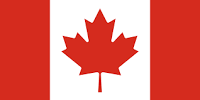
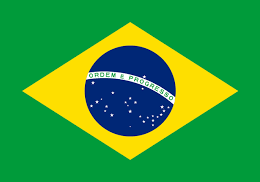

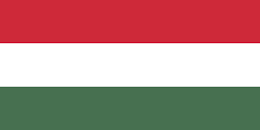
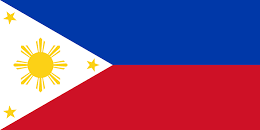
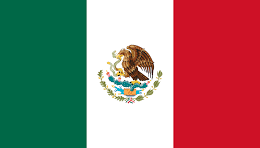
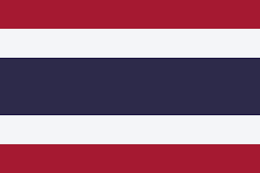
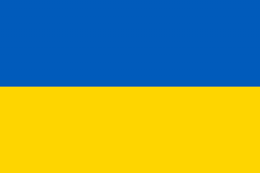
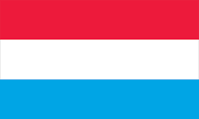
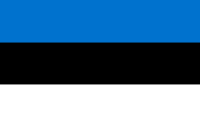
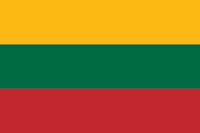
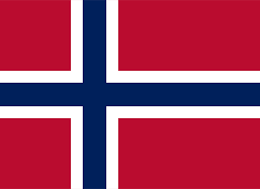
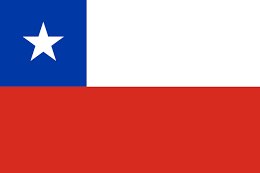
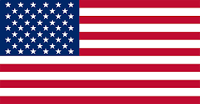
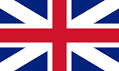
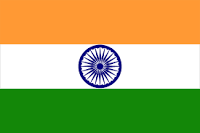
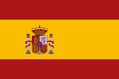
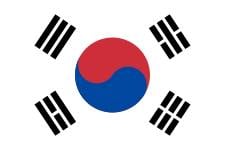
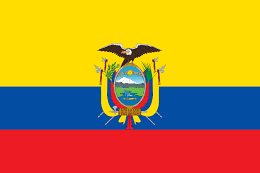
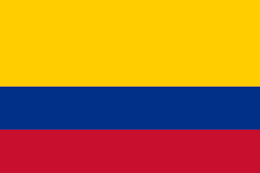
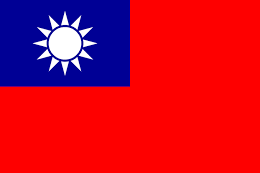
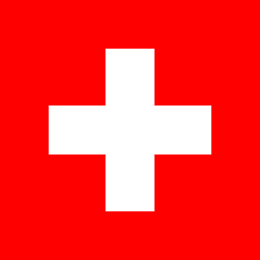
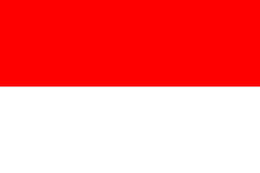
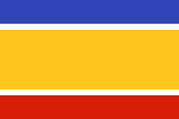
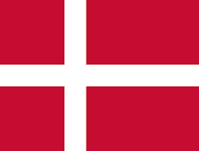
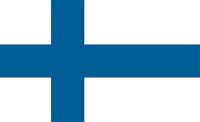
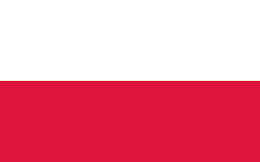
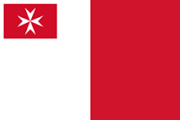
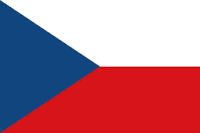
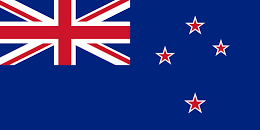
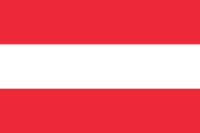
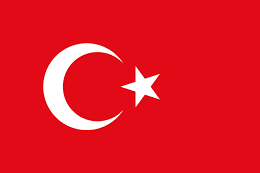
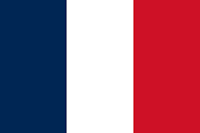
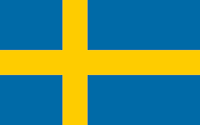
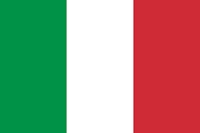
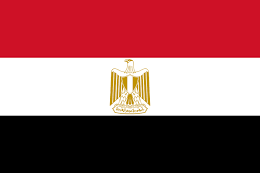
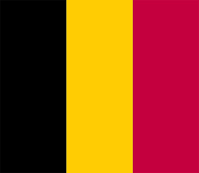
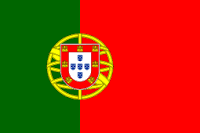
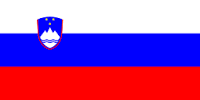
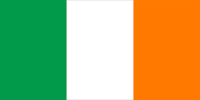
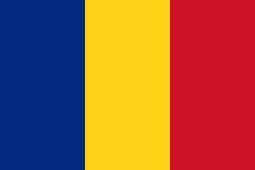
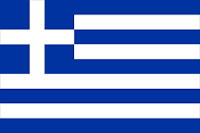
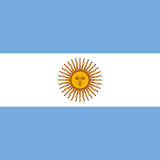
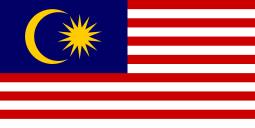
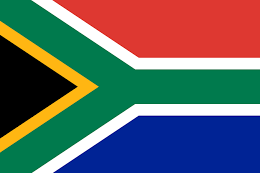
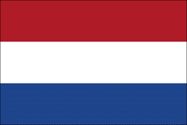
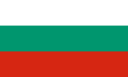
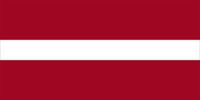
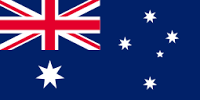
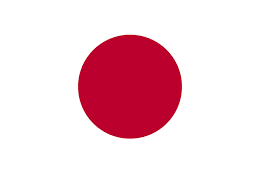
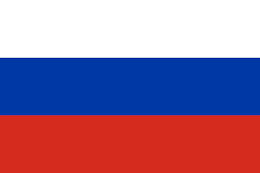