DQN on Keras
To illustrate DQN, the CartPole-v0
environment of the OpenAI Gym is used. CartPole-v0
is a pole balancing problem. The goal is to keep the pole from falling over. The environment is 2D. The action space is made of two discrete actions (left and right movements). However, the state space is continuous and is made of four variables:
- Linear position
- Linear velocity
- Angle of rotation
- Angular velocity
The CartPole-v0
is shown in Figure 9.6.1.
Initially, the pole is upright. A reward of +1 is provided for every timestep that the pole remains upright. The episode ends when the pole exceeds 15 degrees from the vertical or 2.4 units from the center. The CartPole-v0
problem is considered solved if the average reward is 195.0 in 100 consecutive trials:
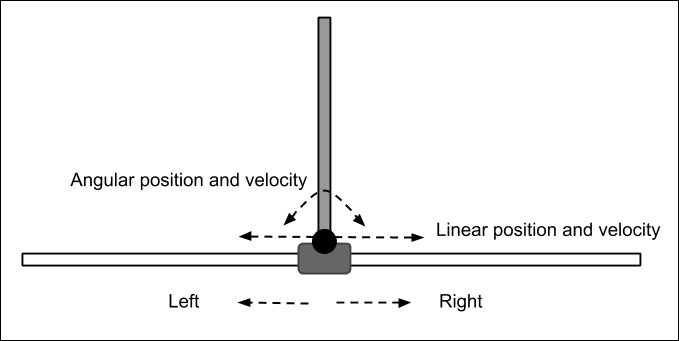
Figure 9.6.1: The CartPole-v0 environment
Listing 9.6.1 shows us the DQN implementation for CartPole-v0
. The DQNAgent
class represents the agent using DQN. Two Q-Networks are created:
- Q-Network or Q in Algorithm 9.6.1
- Target Q-Network or Qtarget...