In this section, we will be using decision trees and student performance data to predict whether a child will do well in school. We will use the previous techniques with some scikit-learn code. Before starting with the prediction, let's just learn a bit about what decision trees are.
Decision trees are one of the simplest techniques for classification. They can be compared with a game of 20 questions, where each node in the tree is either a leaf node or a question node. Consider the case of Titanic survivability, which was built from a dataset that includes data on the survival outcome of each passenger of the Titanic.
Consider our first node as a question: Is the passenger a male? If not, then the passenger most likely survived. Otherwise, we would have another question to ask about the male passengers: Was the male over the age of 9.5? (where 9.5 was chosen by the decision tree learning procedure as an ideal split of the data). If the answer is Yes, then the passenger most likely did not survive. If the answer is No, then it will raise another question: Is the passenger a sibling? The following diagram will give you a brief explanation:
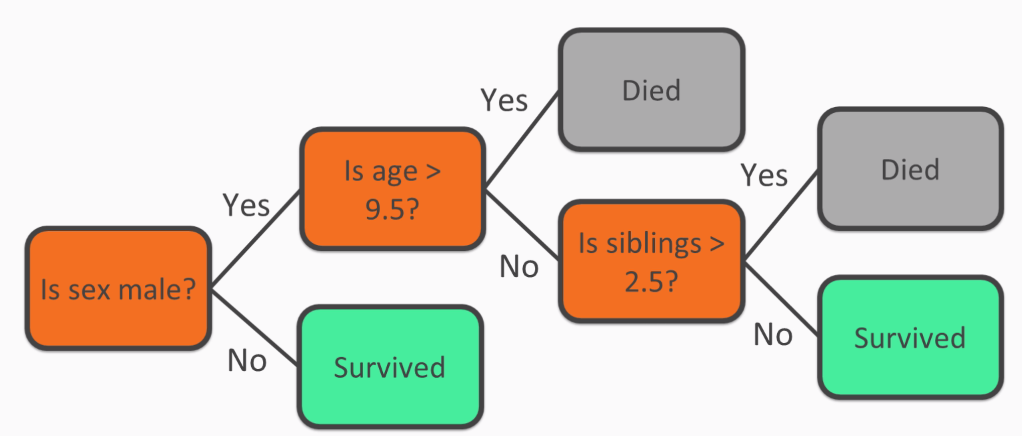
Understanding the decision trees does not require you to be an expert in the decision tree learning process. As seen in the previous diagram, the process makes understanding data very simple. Not all machine learning models are as easy to understand as decision trees.
Let us now dive deep into decision tree by knowing more about decision tree learning process. Considering the same titanic dataset we used earlier, we will find the best attribute to split on according to information gain, which is also known as entropy:
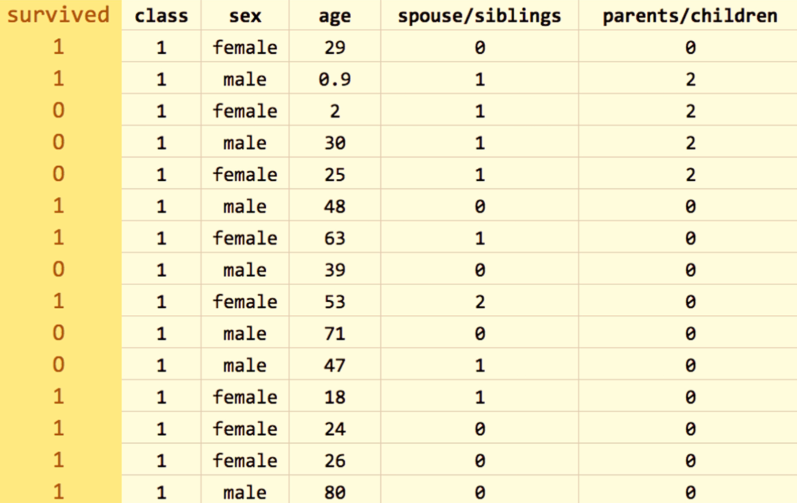
Information gain is highest only when the outcome is more predictable after knowing the value in a certain column. In other words, if we know whether the passenger is male or female, we will know whether he or she survived, hence the information gain is highest for the sex column. We do not consider age column best for our first split since we do not know much about the passengers ages, and is not the best first split because we will know less about the outcome if all we know is a passenger's age.
After splitting on the sex column according to the information gain, what we have now is female and male subsets, as seen in the following screenshot:
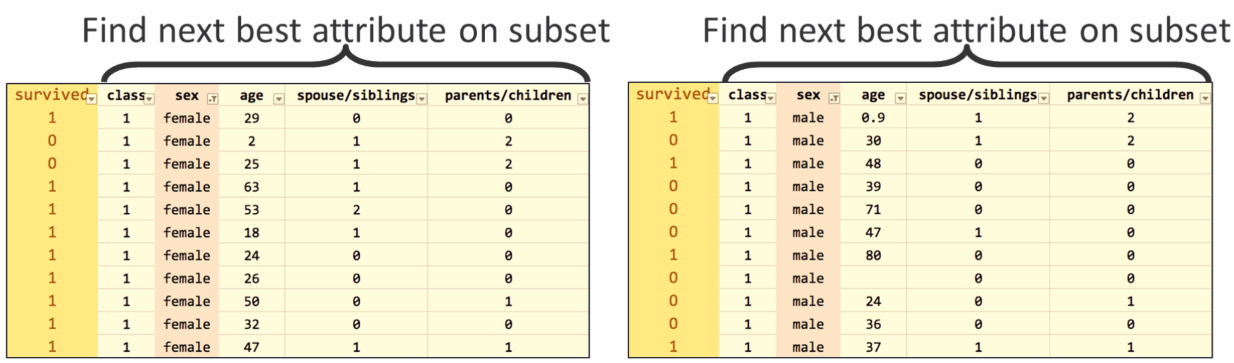
After the split, we have one internode and one question node, as seen in the previous screenshot, and two paths that can be taken depending on the answer to the question. Now we need to find the best attribute again in both of the subsets. The left subset, in which all passengers are female, does not have a good attribute to split on because many passengers survived. Hence, the left subset just turns into a leaf node that predicts survival. On the right-hand side, the age attribute is chosen as the best split, considering the value 9.5 years of age as the split. We gain two more subsets: age greater than 9.5 and age lower than 9.5:
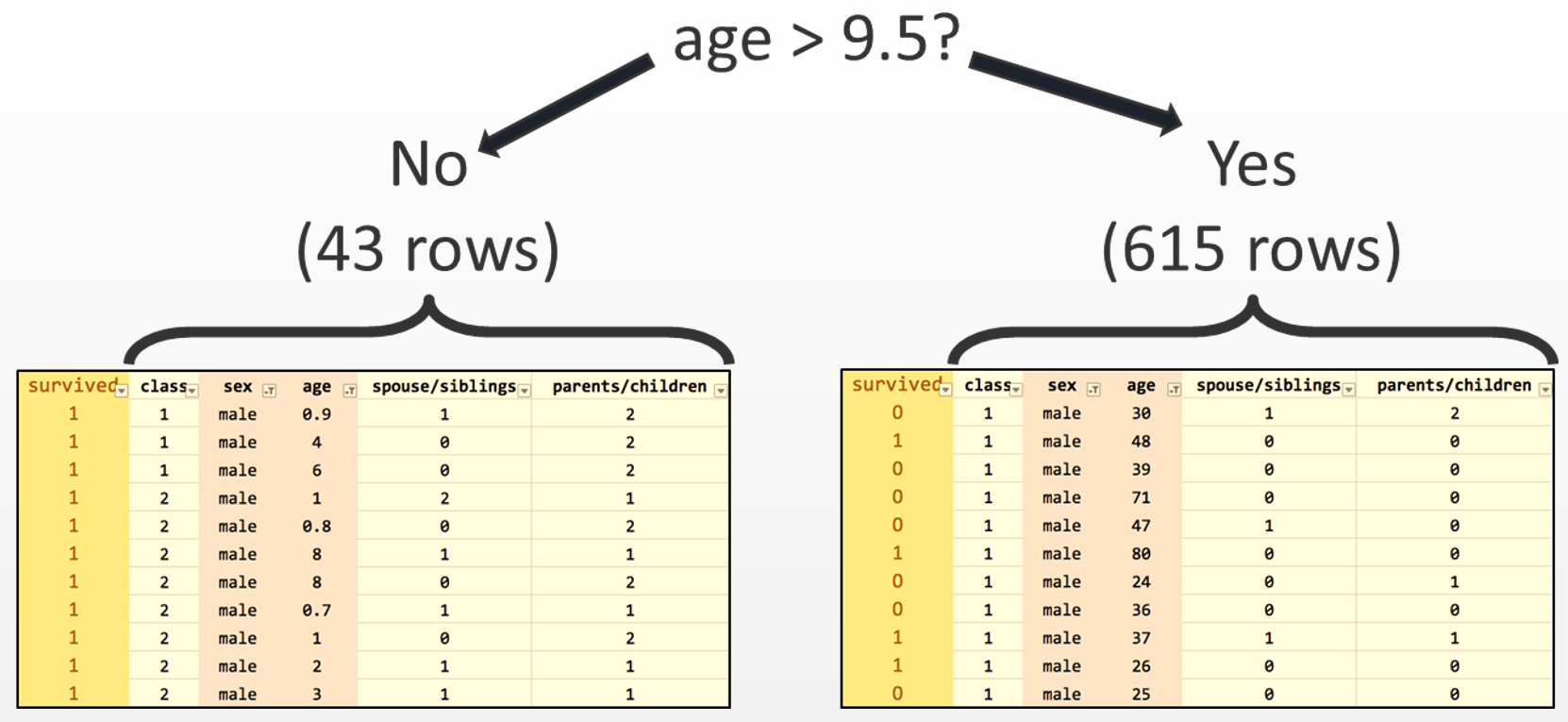
Repeat the process of splitting the data into two new subsets until there are no good splits, or no remaining attributes, and leaf nodes are formed instead of question nodes. Before we start with our prediction model, let us know a little more about the scikit-learn package.