In this chapter, we presented different techniques that can be employed for both dimensionality reduction and dictionary learning. PCA is a very well-known method that involves finding the most import components of the dataset associated with the directions where the variance is larger. This method has the double effect of diagonalizing the covariance matrix and providing an immediate measure of the importance of each feature, so as to simplify the selection and maximize the residual explained variance (the amount of variance that it is possible to explain with a smaller number of components). As PCA is intrinsically a linear method, it cannot often be employed with non-linear datasets. For this reason, a kernel-based variant has been developed. In our example, you saw how an RBF kernel is able to project a non-linearly separable dataset onto a subspace, where PCA can...
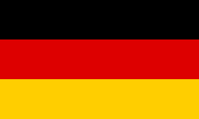
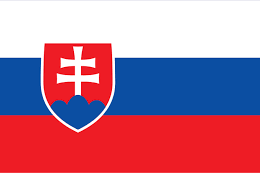
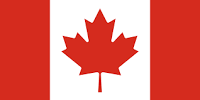
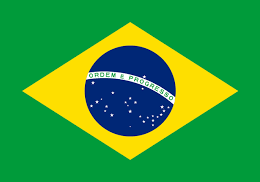

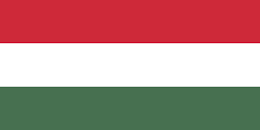
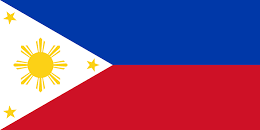
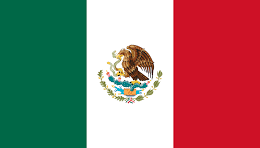
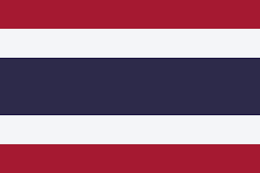
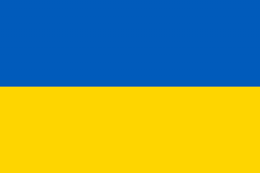
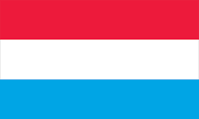
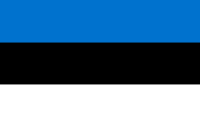
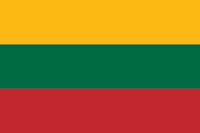
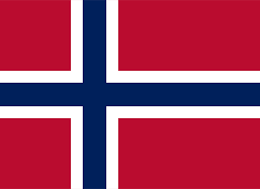
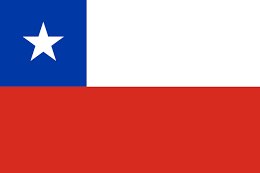
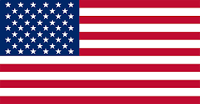
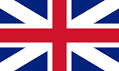
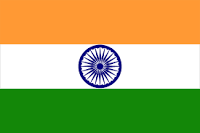
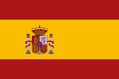
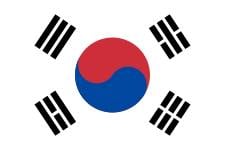
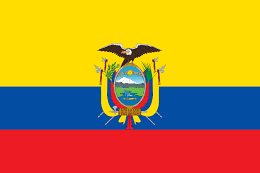
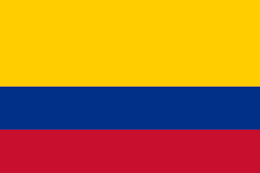
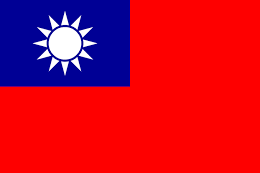
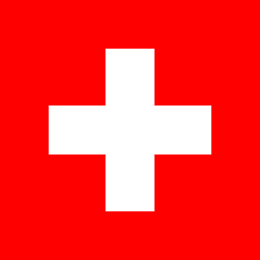
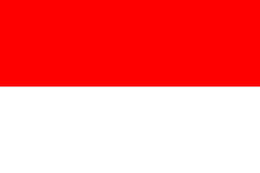
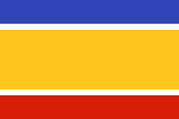
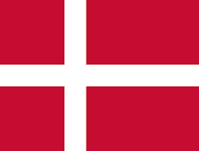
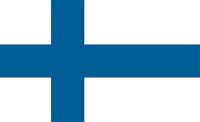
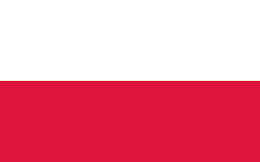
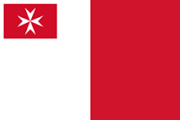
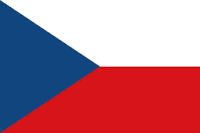
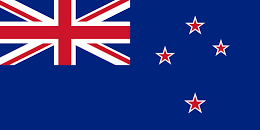
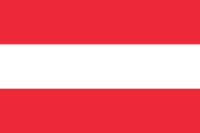
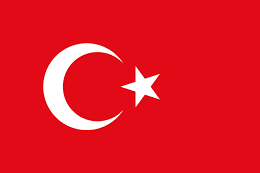
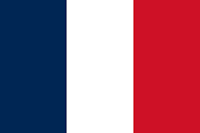
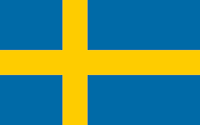
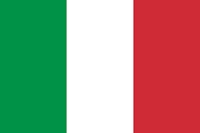
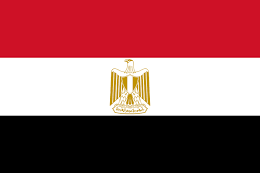
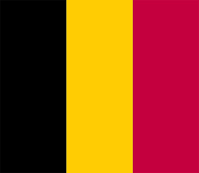
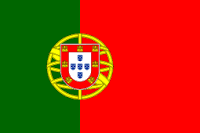
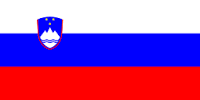
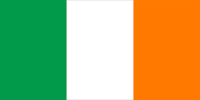
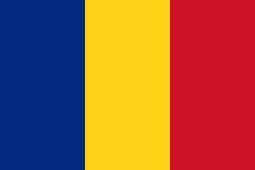
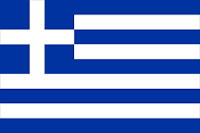
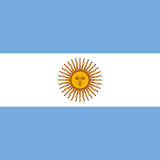
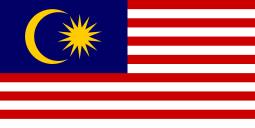
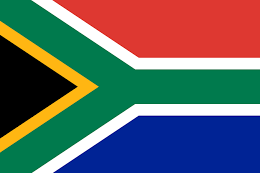
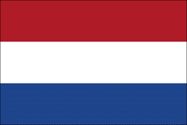
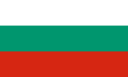
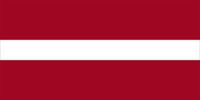
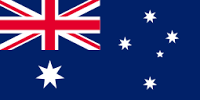
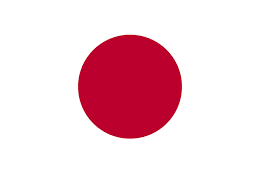
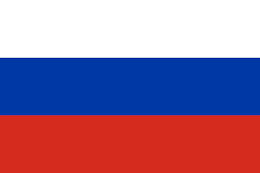