Approximating functions
There are many views on how best to think about neural networks, but perhaps the most useful is to see them as function approximators. Functions in math relate some input, x, to some output, y. We can write it as the following formula:
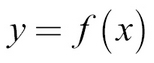
A simple function could be like this:
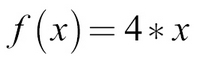
In this case, we can give the function an input, x, and it would quadruple it:
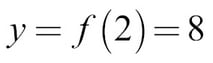
You might have seen functions like this in school, but functions can do more; as an example, they can map an element from a set (the collection of values the function accepts) to another element of a set. These sets can be something other than simple numbers.
A function could, for example, also map an image to an identification of what is in the image:

This function would map an image of a cat to the label "cat," as we can see in the following diagram:
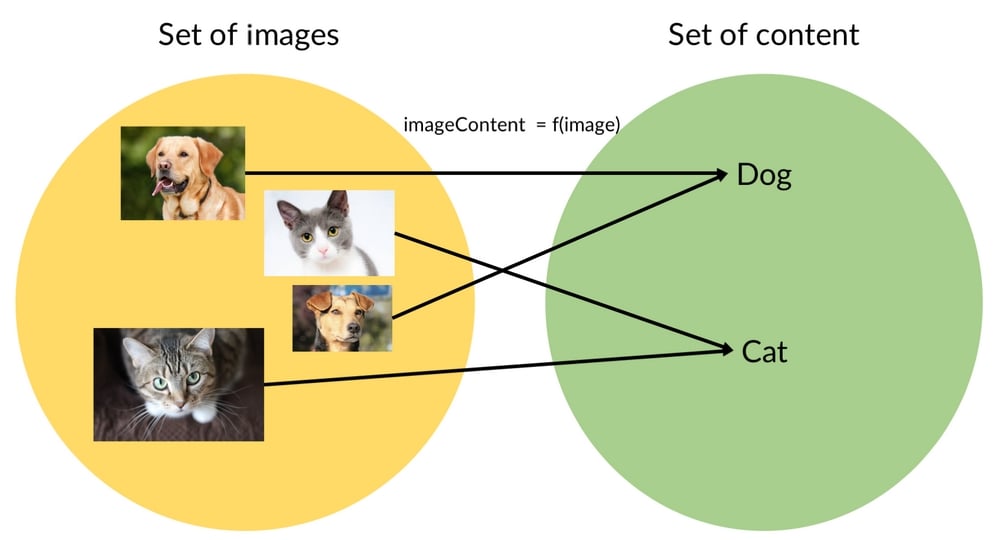
Mapping images to labels
We should note that for a computer, images are matrices full of numbers and any description of an image's content would also be stored as a matrix of numbers.
A neural network, if it is big enough, can approximate any function. It has been mathematically proven that an indefinitely large network could approximate every function. While we don't need to use an indefinitely large network, we are certainly using very large networks.
Modern deep learning architectures can have tens or even hundreds of layers and millions of parameters, so only storing the model already takes up a few gigabytes. This means that a neural network, if it's big enough, could also approximate our function, f, for mapping images to their content.
The condition that the neural network has to be "big enough" explains why deep (big) neural networks have taken off. The fact that "big enough" neural networks can approximate any function means that they are useful for a large number of tasks.