Polars Series
Series is an important concept in a DataFrame library. A DataFrame is made up of one or more Series. A Series is like a list or array: it’s a one-dimensional structure that stores a list of values. A Series is different than a list or array in Python in that a Series is viewed as a column in a table, containing the list of data points or values of a certain data type. Just like the Polars DataFrame, the Polars Series also has many built-in methods you can utilize for your data transformations. In this recipe, we’ll cover the creation of Polars Series as well as how to inspect its attributes.
Getting ready
As usual, make that sure you import the Polars library at the beginning of your code if you haven’t already:
import polars as pl
How to do it...
We’ll first create a Series and explore its attributes.
- Create a Series from scratch:
s = pl.Series('col', [1,2,3,4,5]) s.head()
The preceding code will return the following output:
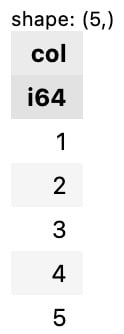
Figure 1.6 – Polars Series
- Create a Series from a DataFrame with the
.to_series()
and.
get_column()
methods:- First, let’s convert a DataFrame to a Series with
.to_series()
:data = {'a': [1,2,3], 'b': [4,5,6]} s_a = ( pl.DataFrame(data) .to_series() ) s_a.head()
The preceding code will return the following output:
- First, let’s convert a DataFrame to a Series with
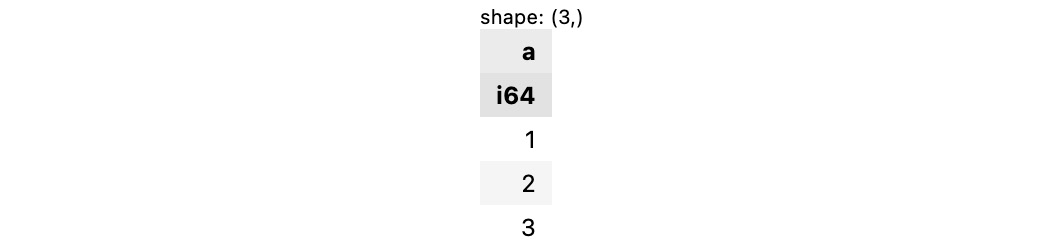
Figure 1.7 – A Series from a DataFrame
- By default,
.to_series()
returns the first column. You can specify the column by either index:s_b = ( pl.DataFrame(data) .to_series(1) ) s_b.head()
- When you want to retrieve a column for a Series, you can use
.
get_columns()
instead:s_b2 = ( pl.DataFrame(data) .get_column('b') ) s_b2.head()
The preceding code will return the following output:
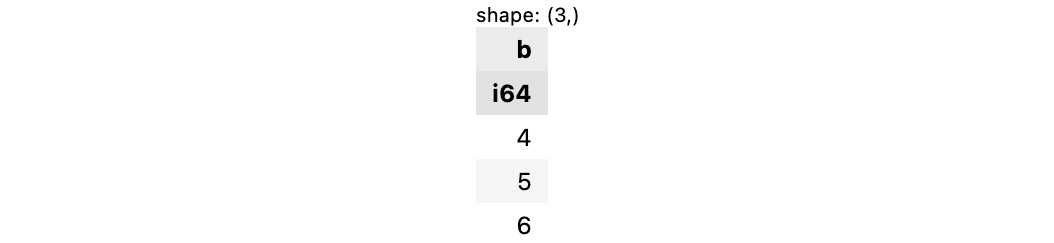
Figure 1.8 – Different ways to extract a Series from a DataFrame
- Display Series attributes:
- Get the length and width with
.shape
:s.shape
The preceding code will return the following output:
>> (5,)
- Use
.name
to get the column name:s.name
The preceding code will return the following output:
>> 'col'
.dtype
gives you the data type:s.dtype
The preceding code will return the following output:
>> Int64
- Get the length and width with
How it works...
The process of creating a Series and getting its attributes is similar to that of creating a DataFrame. There are many other methods that are common across DataFrame and Series. Knowing how to work with DataFrame means knowing how to work with Series and vice-versa.
There’s more...
Just like DataFrame, Series can be converted between other structures such as a NumPy array and pandas Series. We won’t get into details on that in this book, but we’ll go over this for DataFrame later in the book in Chapter 10, Interoperability with Other Python Libraries.
See also
If you’d like to learn more, please visit Polars’ documentation page: https://pola-rs.github.io/polars/py-polars/html/reference/series/index.html.