In today's world, distributed systems are everywhere. Ranging from the websites we browse to the apps that we use on our phones, hardly a day passes when we do not use distributed systems. Given this omnipresent nature, it is an obvious choice to adapt this paradigm for building machine learning systems. A typical pattern in building distributed systems is to perform resource-intensive (and data-sensitive) computations on backend servers whilst pushing lighter (and comparatively independent) compute tasks to the user's device. A large subset of machine learning applications falls into the resource-intensive category. Furthermore, machine learning models are built using data. In a significant fraction of real-world cases, the data used to do so is subject to privacy and security concerns. This further advances the case for implementing machine...
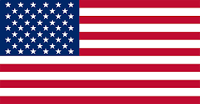
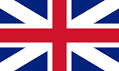
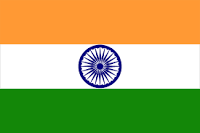
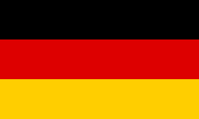
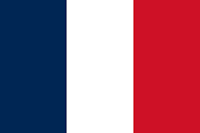
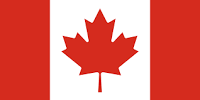
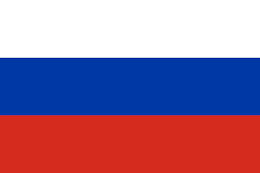
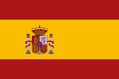
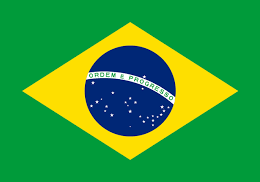
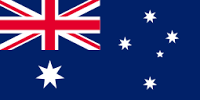

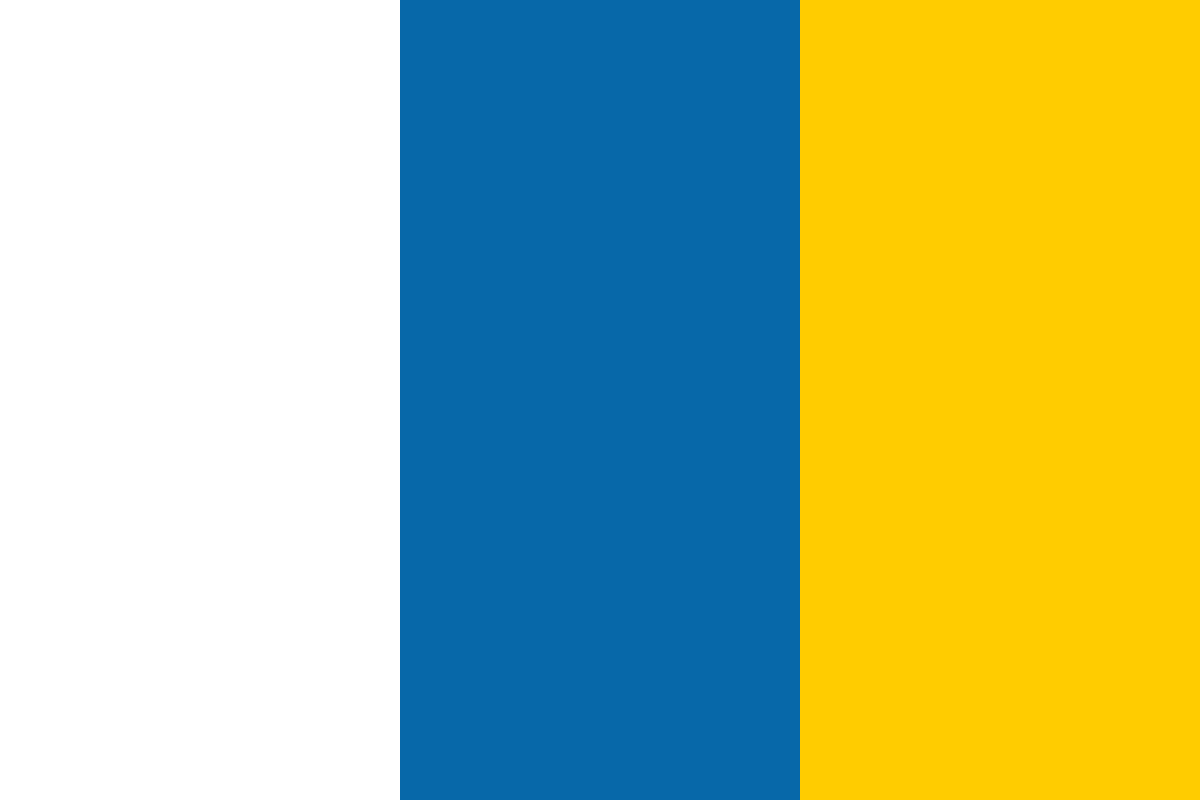
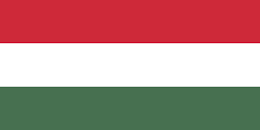
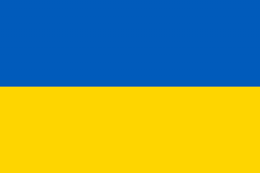
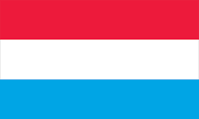
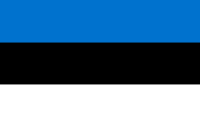
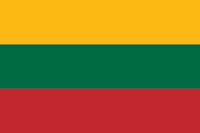
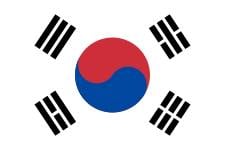
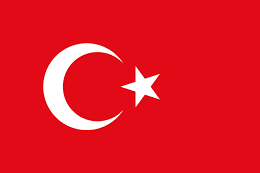
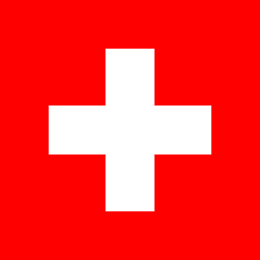
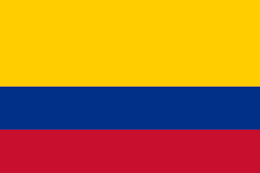
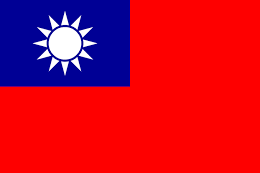
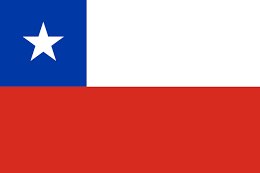
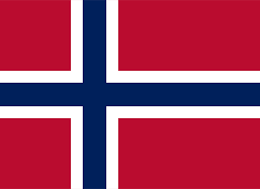
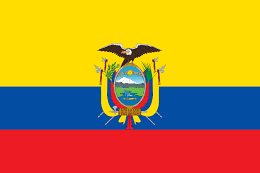
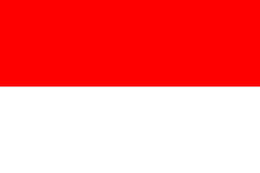
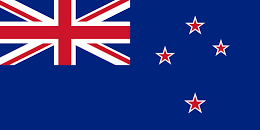
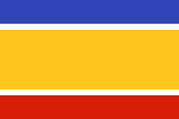
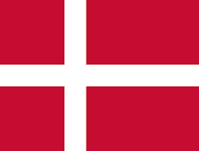
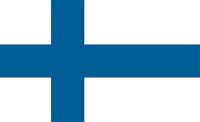
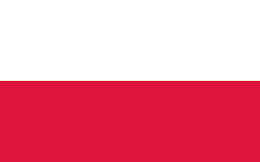
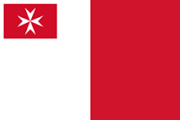
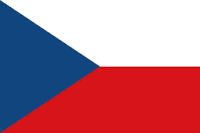
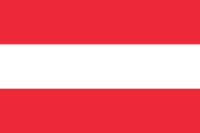
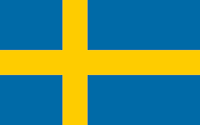
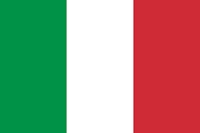
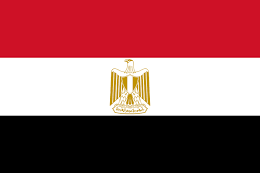
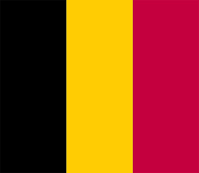
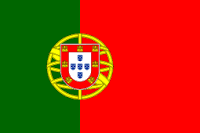
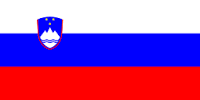
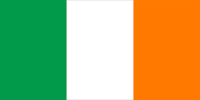
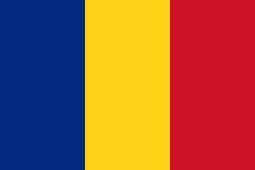
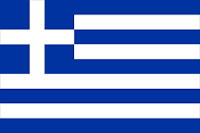
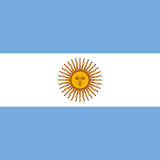
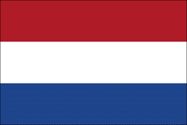
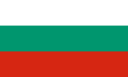
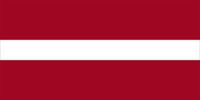
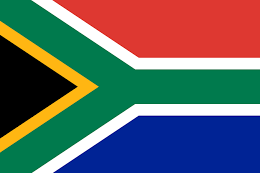
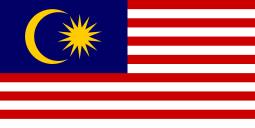
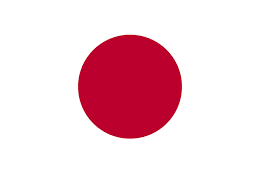
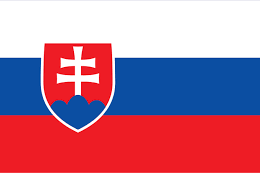
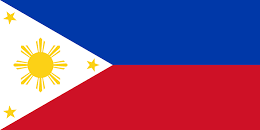
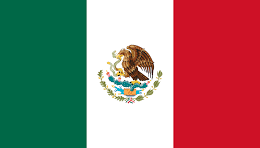
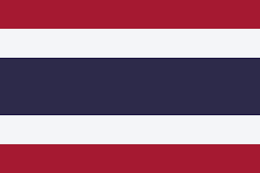