Digitization trends
Enterprises are seeking to get a deeper understanding of their data and provide differentiated, personalized experiences for their employees, customers, and partners. This requires modern applications to be created that are more responsive and can be used by clients across different types of devices. This also requires collecting a lot more data and applying artificial intelligence and machine learning to create personalized insights. This experience must be highly scalable, available, and made available for large set of users. This means it has to be built and managed on hybrid multi-cloud platforms leveraging an automated DevOps pipeline. We will discuss the impact of this digital transformation across architecture, application, data, integration, management, automation, development, and operations. Security and compliance are important cross-cutting concerns that needs to be addressed for each of these areas as part of this transformation.
Application modernization
The key opportunities and challenges with application modernization in the context of hybrid cloud are discussed in the following diagram:
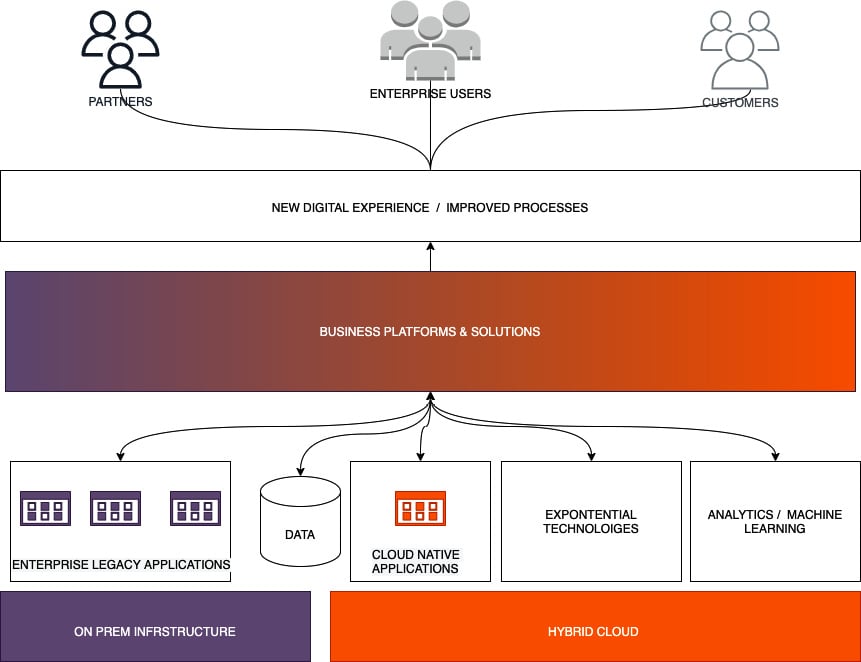
Figure 1.5 – Application modernization
The key trends in application modernization and migration to the cloud are listed as follows:
- Cloud-native applications: Companies are building new capabilities as cloud-native applications that are based on microservices. Taking a cloud-native approach provides high availability. Applications are able to launch to the market quickly and scale efficiently by taking a cloud-native approach. Microservices have emerged as the preferred architectural style for building cloud-native applications. This structures an application as a collection of services that are aligned to business requirements, loosely coupled, independently deployable, scalable, and highly available.
- Modernize and migrate existing applications to the cloud: Companies are looking at various options to modernize their legacy applications and make it ready for the digital era. They are considering the following:
- Lifting and shifting to the cloud, where the application and associated data is moved directly to cloud without any changes or redesigning.
- Re-platforming to a virtualized platform.
- Exposing application data through APIs.
- Containerizing the existing application.
- Refactoring or completely rewriting into new microservices.
- Strangling the monolith and moving to microservices over time, where a large legacy application is restrained from adding new features and incrementally transformed to new services based on cloud-native principles.
- Built-in intelligence: Modern apps include cognitive capabilities and participate in automated workflows. We are seeing this in all the domains across banking, telecom, insurance, retail, HR, and government. Bots or applications answer human queries and provide a better experience. This positive technology also is playing a key soldier role in the healthcare sector to help mankind fight the pandemic.
- Modernizing batch functions in the cloud: This is another area that is growing rapidly. Some capabilities such as serverless computing and code engines help to build great applications in shorter time frames. These concepts will be discussed in detail in a later chapter. Essentially, these capabilities or application styles help offload otherwise long-running and resource-hungry tasks to asynchronous jobs. Thus, the cloud provides optimized scale and cost efficiency. Batch jobs that take lot of runtime and processing power or cost in costly legacy systems may be done at a much lower cost with these computing styles.
- Runtimes: As part of their modernization strategies, enterprises are evaluating how to minimize the cost of ownership and operations of the apps as well. To this end, some of the traditional applications are getting rearchitected to leverage cloud-native capabilities such as cloud functions or code engines. Another big set of applications are those that needs to be sunset or retired because the compilers or runtimes are going out of service. There is a demand for newer runtimes and faster virtual machines with lighter footprints in terms of resource usage.
- Tools: 80% of the core applications are still on legacy platforms. For businesses to move them to the cloud requires greater automation. Many intelligent tools for the discovery and extraction of logic and business rules that are more domain- or industry-focused exist.
Data modernization and the emergence of data fabric
We are at a tipping point in history where technology is transforming the way that business gets done. Businesses are intelligently leveraging analytics, artificial intelligence, and machine learning. How businesses collect, organize, and analyze data and infuse artificial intelligence (AI) will be the key to this journey being successful.
There are several data transformation use case scenarios for a hybrid multi-cloud architecture. Many companies have to modernize monoliths to cloud-native with distributed data management techniques to deliver digital personalized experiences. This involves transforming legacy architecture characterized by data monoliths, data silos, the tight coupling of data, high Total Cost of Ownership (TCO), and low speeds into new technologies identified by increased data velocity, variety, and veracity. Data governance is another key use case where enterprises need visibility and control over the data spread across hybrid multi-cloud solutions, data in motion, and data permeating enterprise boundaries (for example, the blockchain). Enterprises need to better efficiency and resiliency, with improved security for their data middleware itself and the workloads.
The greatest trend is related to delivering personal and empathetic customer service and experiences anchored by individual preferences. This is nothing new but how we enable this in a hybrid cloud is important. Customers have their System of Records (SoRs) locked in filesystems and traditional databases. There is no easy way to introduce new digital products that leverage these legacy data sources. What is required is to have a strategy to unlock the data in the legacy system to participate in these digital plays. To do this, enterprises take multiple approaches, which are listed as follows:
- One is to leave the SoRs or the Source of Truth (SoT), wherever it is, and add the ability to access the data through APIs in order to participate in digital transactions.
- A second option is to move the core itself to the cloud, getting rid of the constraints to introduce new products more quickly with data on the cloud in the process. In this model, a single legacy database could end up as multiple databases on the cloud. This will remove the dependency on data for the new development teams, thus speeding up the delivery of new digital capabilities.
Another key observation is that the line between transactional and analytical worlds is becoming thinner. Traditionally, enterprises handled their transactional data and analytics separately. While one side was looking into how to scale rapids to support transactions from multiple users and devices, on the other side, analytics systems were looking to provide insights that could drive personalization further. Insights and AI help optimize production processes and act to balance quality, cost, and throughput. This use case also involves using artificial intelligence services to transform the customer experience and guarantee satisfaction at every touchpoint. Within the industrial, manufacturing, travel and transportation, and process sectors, it is all about getting greater insight from the data that’s being produced. This data is used to drive immediate real-time actions. So, we see operation analytics (asset management), smarter processes (airport or port management), information from edge deployments, security monitoring driving new insights, and the insights, in turn, driving new types of business.
The emergence of data fabric
Businesses are looking to optimize their investment in analytics to drive real-time results to deliver personalized experiences with improved reliability and drive optimization in operations and response. This is their objective – to raise maturity on the journey to becoming a data-driven organization. Companies invest in industry-based analytics platforms that will drive this agenda related to cognitive capabilities to modernize the ecosystem and enable higher value business functions.
Given the intermingling of transactional data and analytics data, something called the data fabric emerges. It simply means companies don’t need to worry about where the data is, how it is stored, and what format it is available in. The data fabric does the hard work of making it available to the systems at the time they need it and in the form required.
The concept and value of a data fabric bridging the transactional and analytics world is detailed in the following diagram:
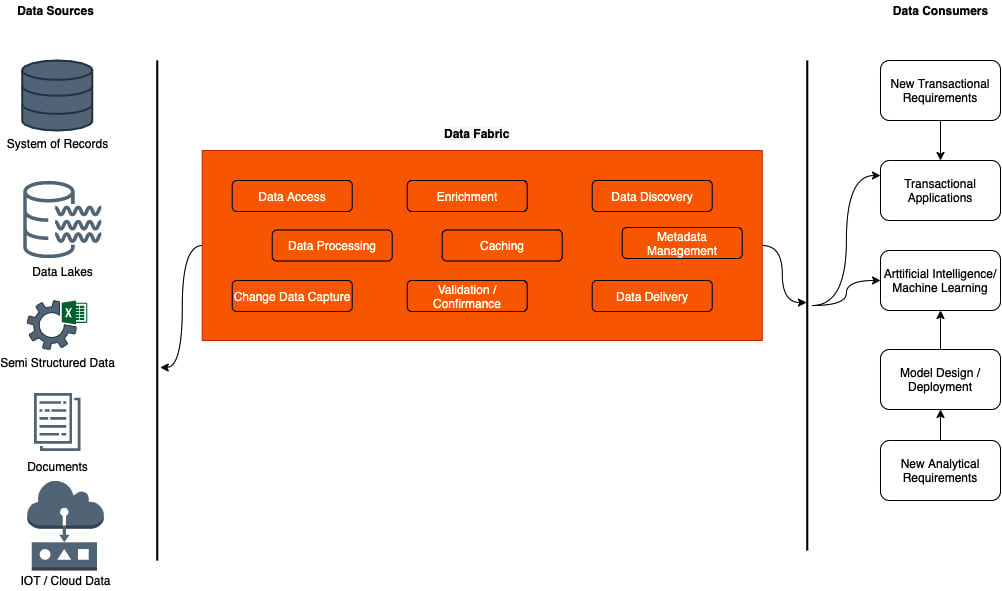
Figure 1.6 – The data fabric
Cloud, data hub, data fabric, and data quality initiatives will be intertwined as data and analytics leaders strive for greater operational efficiency across their data management landscape. Data fabrics remain an emerging key area of interest, enabled by active metadata.
Various architecture aspects of this fabric leverage hybrid multi-cloud containerized capabilities to accelerate the integration of AI infusion aspects with transaction systems.
Lowering the cost of data acquisition and management is another key area that enterprises are dealing with. They are required to manage heterogenous transactional and analytical data environments. So, they need help with decision data and analytics workload dispositions to the cloud. Cost optimization through adopting new cloud-based database technologies is accelerating the move from on-premises databases to cloud-based database technologies. A hybrid cloud strategy providing efficient solutions for how data is stored, managed, and accessed is a prerequisite to these activities.
Transactional and master data management within an organization changes from application to application in terms of its interpretation and granularity. This is another evolving theme of central versus distributed management of data. The fast-adopted model is to synchronize access to this data across the organization orchestrated through a data fabric or DataOps.
Integration, coexistence, and interoperability
The majority of enterprise clients rely on a huge integration landscape. The traditional enterprise integration landscape is still the core of many businesses powering their existing Business to Business (B2B), Business to Consumer (B2C), and Business to Employee (B2E) integrations. Enterprises need the agility of their integration layer to align with the parallel advances occurring in their application delivery.
When creating new systems of engagement, cloud engineering teams are looking for new ways to integrate with systems of records. They want to rearchitect, refactor, or reconstruct this middle layer. The demands on this middle layer are increasing as end users demand more responsive apps. Squads building new applications in the form of APIs and microservices like to be in complete control end-to-end, including at the integration layer.
The following diagram shows how the integration layer is modernized to support the needs of a hybrid cloud environment covering coexistence and interoperability aspects as well:
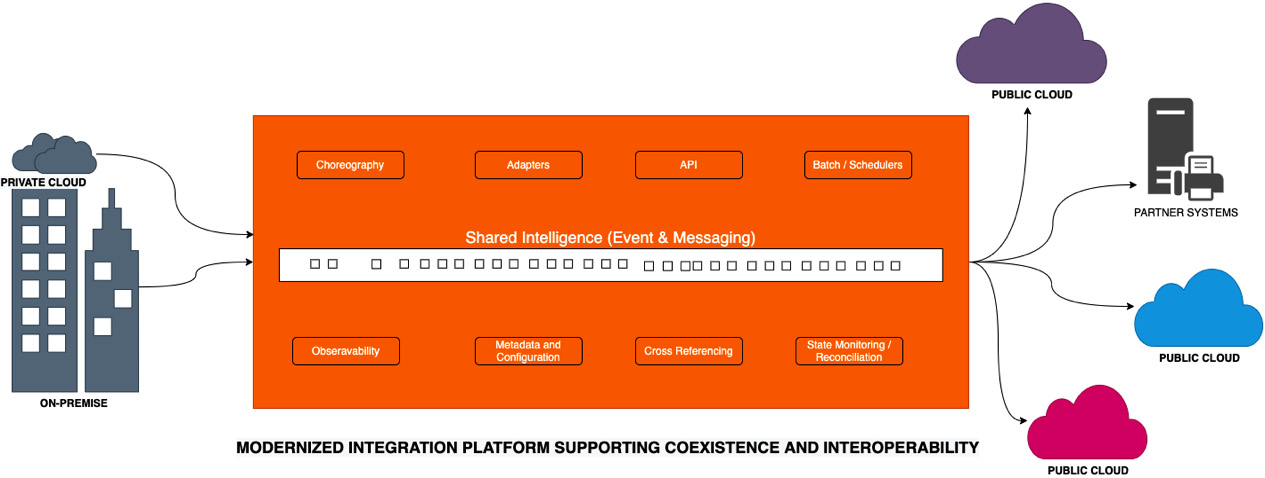
Figure 1.7 – Integration modernization, coexistence, and interoperability
Enterprises are adopting newer hybrid cloud architecture patterns to reach a higher level of maturity with optimized and agile integration. They are also looking for better ways to deploy and manage integrations. There is a need for an optimized CI/CD pipeline for integrations and the capability to roll out increments. Another requirement is controlling the roll-out of an application and integrating code for different target environments. Thus, we see tools for GitOps being extended to the integration layer as well.
Event hubs and intelligent workflows
Clients are looking to tap into interesting business events that occur within their enterprise and an efficient way to respond or react in real-time. The deployment of an event hub and event-based integrations are becoming the new norm. This also is the key enabler for building some of the intelligent workflows for a cognitive enterprise.
This integration model plays an important role in the hybrid cloud world, bringing together otherwise siloed and static processes. This also enables enterprises to go beyond their boundaries to create a platform for customers, partners, and vendors to collaborate and drive actions derived from real-time data. The applications (event consumers and producers) could be within or outside the enterprise. This space is set for exponential growth in the near future.
Coexistence and interoperability
Many enterprises have a clear understanding of their current landscape and the vision for their target multi-cloud architecture. However, they lack the knowledge of what their next logical step to reach the target is. The critical gap that needs to be addressed by their modernization strategy is related to technical and cultural coexistence and interoperability.
In an incremental modernization journey that is continuously iterative, coexistence and interoperability is a natural byproduct. Coexistence architecture isolates the modernized state from the current state. This layer also hides the complexities and gaps, as well as minimizes any changes to current state processing. Coexistence provides a simple, predictable experience and outcome for an organization to transition from old to new, while interoperability is the ability to execute the same functionality seamlessly in the legacy and the modernized side.
Businesses prioritize their investments and focus on acquiring new capabilities and transforming existing capabilities. Coexistence and interoperability help clients to focus on business transformation with logical steps towards realizing their target architecture. With many moving parts, as well as each of them being transformed and deployed on multicloud environments, this is an even more challenging problem to solve. This layer also needs to be carefully crafted and defined for successful multi-cloud adoption.
DevOps
DevOps is a core element of developing and deploying to the cloud. It combines the development and operation of both technological and cultural tools and practices with a perspective to drive the efficiency and speed of delivering cloud applications. It is important to understand the trends in the space and the impact of the adoption of the hybrid cloud.
Optimization of operations
Large enterprises want to ensure uninterrupted business operations with 99.99% application availability. There are traditional huge monolithic applications that can benefit from re-platforming and re-architecturing to the hybrid cloud. However, the goal is not about transformation in itself but about ensuring the cost of operations is minimized. For the FTEs who work on keeping the application alive, businesses need a better way to reduce the effort involved in releases and change management.
Over the years, these enterprises have created solid engineering teams with tons of scripts and automation to build, deploy, and maintain their existing applications. Some of these may be reused, but there is still more automation to be done to reduce manual work and the associated processes.
Leveraging observability for a better customer experience
Over the years, there has been a lot of software or application intelligence gathered by the build and deploy systems. Enterprises are looking to see how to leverage this information to provide a better experience to their clients. This is where observability comes in. Business needs to tap into this telemetry data to determine the aspects of the application that are heavily accessed following client consumption patterns. This also provides problem areas in the application that need to be addressed. Telemetry data-driven integrated operations to monitor across application environments are a requirement in this space. When observability is combined with the efforts of SREs, we have a beautiful way to create highly available solution components on the cloud that can provide a better user experience.
Several enterprises already use AI in this space to manage and understand immense volumes of data better. This includes understanding how the IT events are related and learn system behaviors. Predictive insights into this observability data with AIOps, where artificial intelligence is leveraged for operations and addressing end user engagement with ChatOps or bot-assisted self-service, is a major trend.
Automation, automation, automation
From the provisioning of new sandbox environments through infrastructure as code, development, and testing, automation is the key to do more with fewer developers. As companies build their CI/CD pipeline, automation drives the consistent process to build, run, test, merge, and deploy to multiple target environments. Automation brings QA and developer teams together to move from build, integration, and delivery to deployment. However much automation is done, there will still be a lot of areas in which to improve for enterprises and automation will always be a requirement for this.
Building pipeline of pipelines for hybrid multi-cloud
Continuous planning, continuous integration (CI), and continuous delivery (CD) pipeline, as shown in the following diagram, are critical components required to operate software delivery in a hybrid cloud:
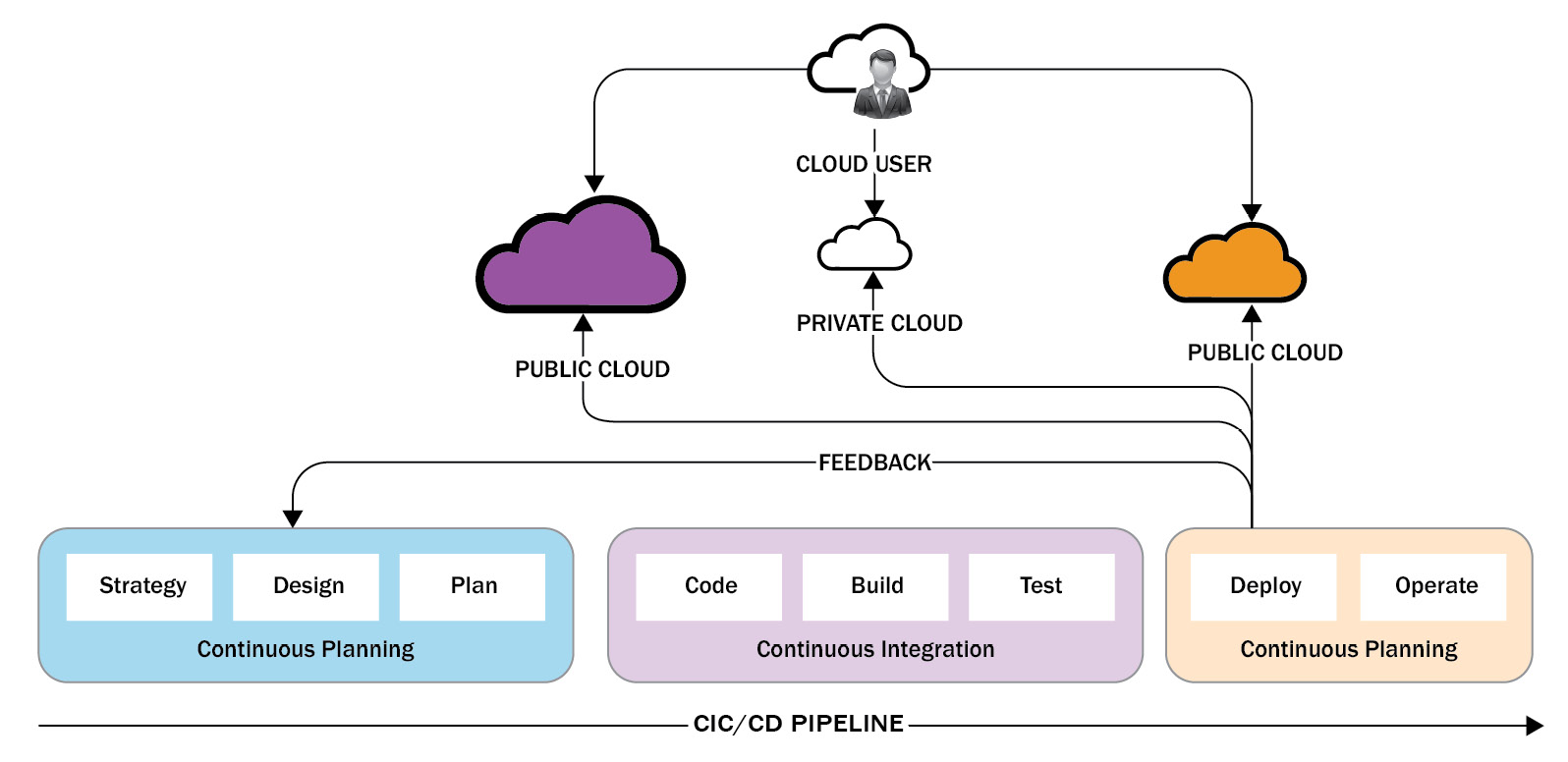
Figure 1.8 – A pipeline of pipelines
Companies need more than one CI/CD pipeline to build and deploy to multiple environments. These DevOps pipelines need to cater to building and deploying to existing and multi-cloud environments. Few are thinking of combining pipelines that can achieve a composite model for hybrid multi-cloud deployment. This is true for applications that have huge middleware and messaging components, which are also going through transformation. Bringing legacy systems into the existing pipeline with common tools and other modern tools is key to enabling application modernization. A robust coexistence and interoperability design sets the direction for an enterprise-wide pipeline. The integration of the pipeline with the service management toolset is another important consideration. Enterprises have to automize with the right set of tools that can also be customized for the target clouds based on the requirements.