In this chapter, you learned both the power of tree-based learning methods for classification problems. Single trees, while easy to build and interpret, may not have the necessary predictive power for many of the problems that we're trying to solve. To improve on the predictive ability, we have the tools of random forest and gradient-boosted trees at our disposal. With random forest, hundreds or even thousands of trees are built and the results aggregated for an overall prediction. Each tree of the random forest is built using a sample of the data called bootstrapping as well as a sample of the predictive variables. As for gradient boosting, an initial, and a relatively small, tree is produced. After this initial tree is built, subsequent trees are produced based on the residuals/misclassifications. The intended result of such a technique is to build a series of trees...
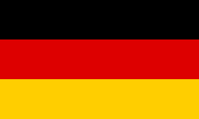
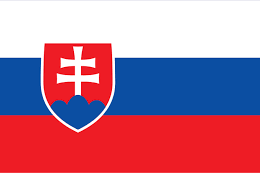
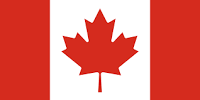
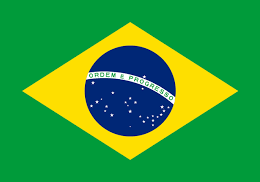

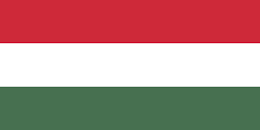
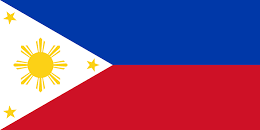
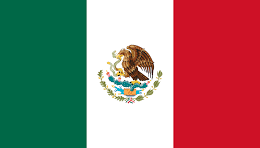
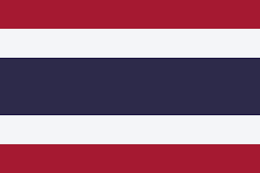
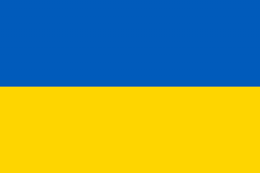
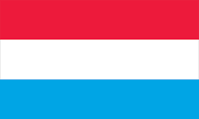
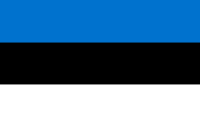
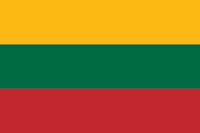
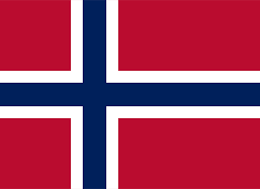
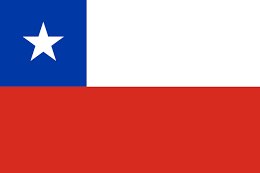
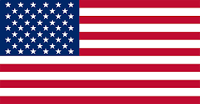
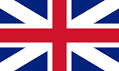
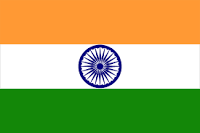
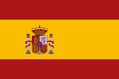
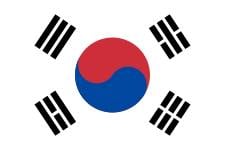
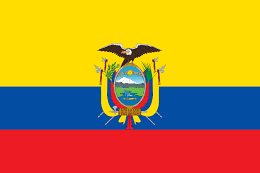
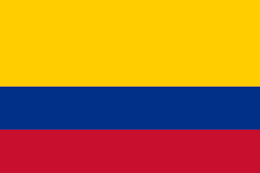
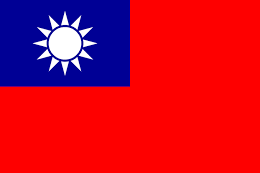
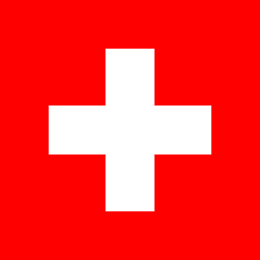
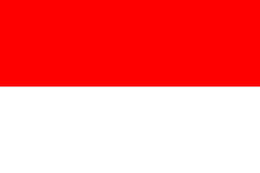
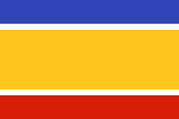
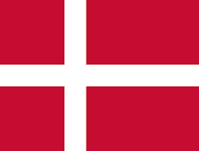
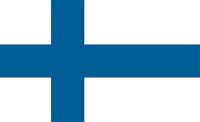
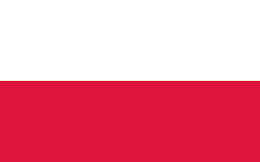
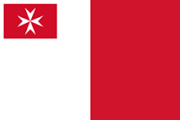
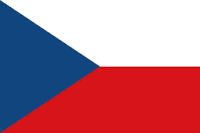
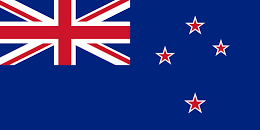
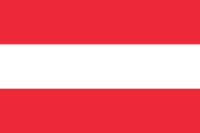
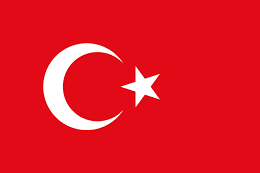
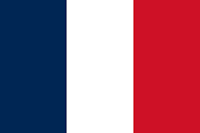
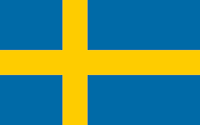
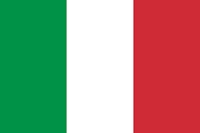
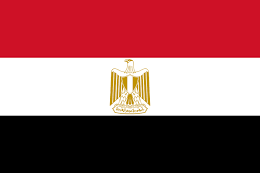
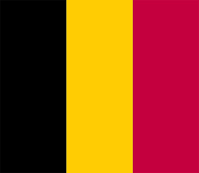
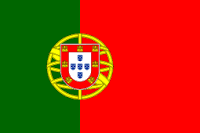
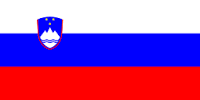
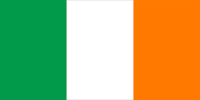
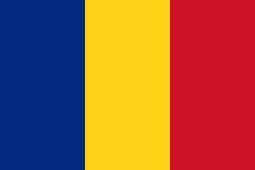
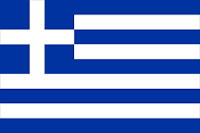
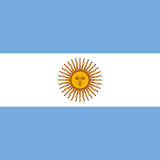
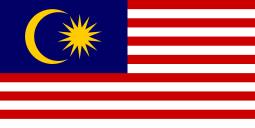
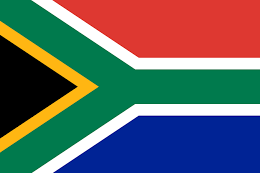
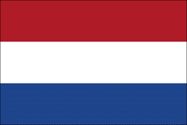
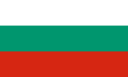
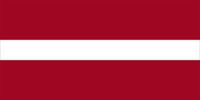
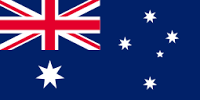
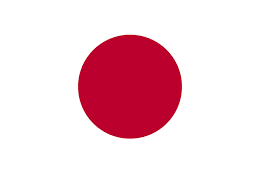
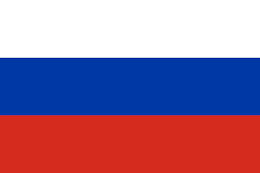