As we mentioned in the introduction, the goal of meta learning is to allow an ML algorithm (in our case, NN) to learn from relatively fewer training samples compared to standard supervised training. Some meta learning algorithms try to achieve this goal by finding a mapping between their existing knowledge of the domain of a well-known task to the domain of a new task. Other algorithms are simply designed from scratch to learn from fewer training samples. Yet another category of algorithms introduce new optimization training techniques, designed specifically with meta learning in mind. But before we discuss these topics, let's introduce some basic meta learning paradigms. In a standard ML supervised learning task, we aim to minimize the cost function J(θ) across a training dataset D by updating the model parameters θ (network weights...
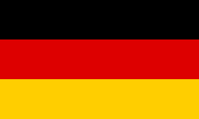
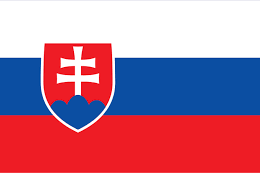
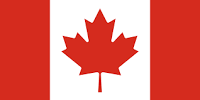
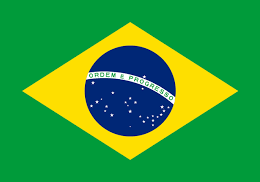

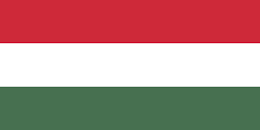
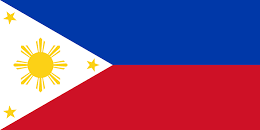
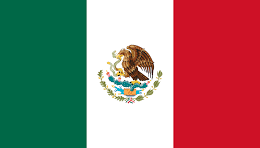
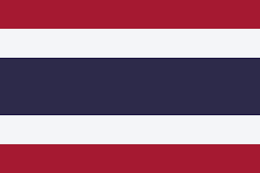
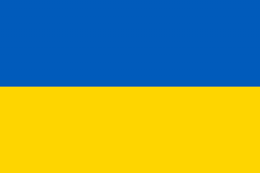
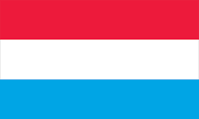
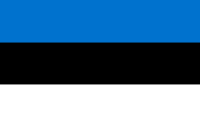
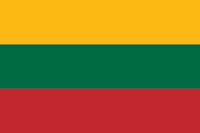
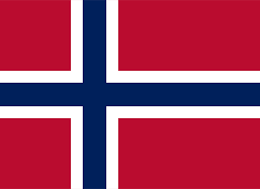
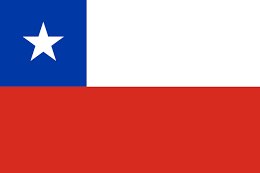
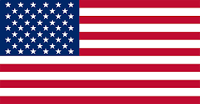
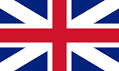
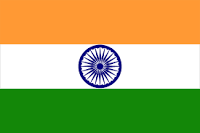
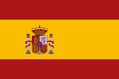
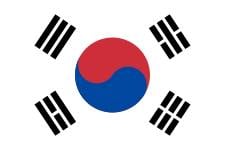
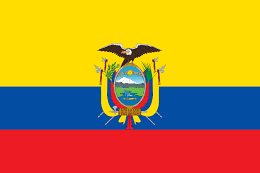
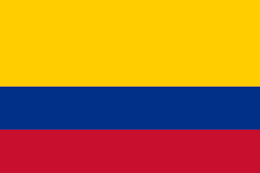
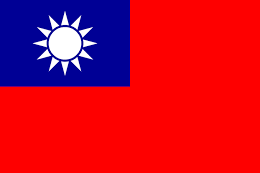
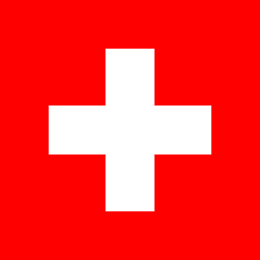
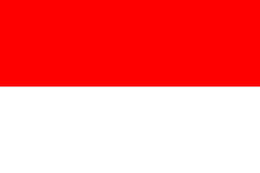
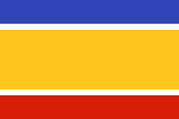
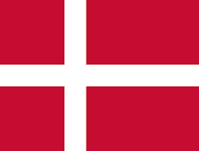
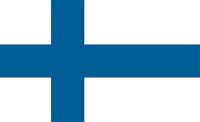
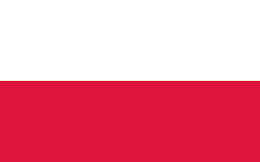
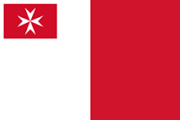
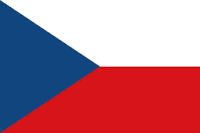
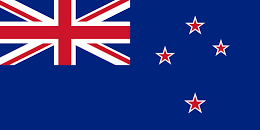
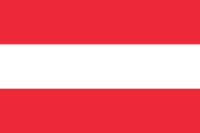
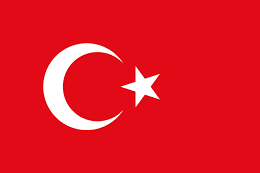
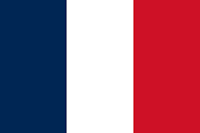
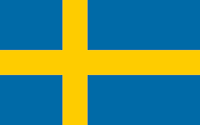
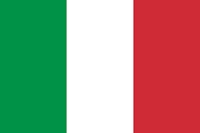
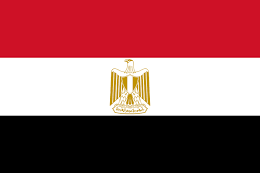
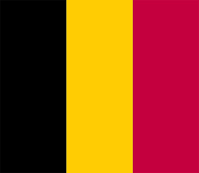
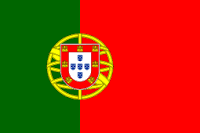
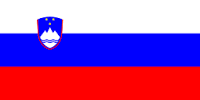
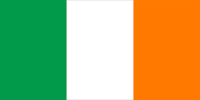
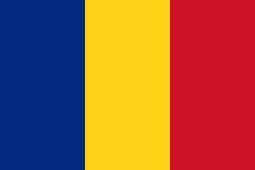
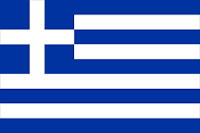
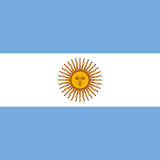
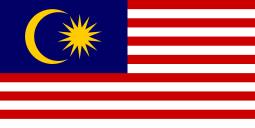
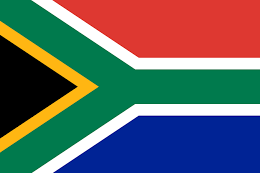
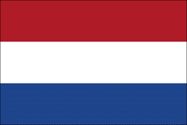
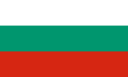
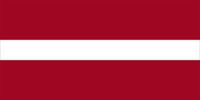
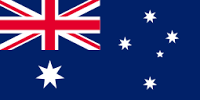
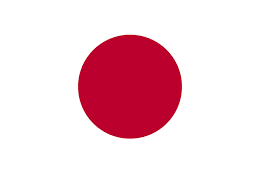
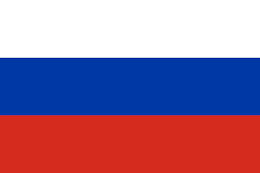