What are models?
There are a lot of definitions of models from the perspective of various domains. When we define a model or use the term model in this book, we will consider it in the context of ML. A model in ML can be seen as a function that has been fine-tuned through training, using some well-engineered data so that the function can recognize and distinguish patterns in unseen data.
Depending on the problem and business goal, a different model can be used, for example, a linear regression model, a logistic regression model, a naive Bayes model, or a decision tree model. These models’ underlying logical representations are different from each other. However, we use the generic term model and the problem domain and name of the ML algorithm to give us a clear picture of what the model is, how it was trained, and how the model is represented. For example, if we are told that the model is for linear regression, then we know that it was trained by minimizing a cost function iteratively using the training data, and it can be saved by storing the regression parameters’ coefficients and intercepts. Similarly, other models will have different algorithms for training and storing. For a deep learning model, we might have to use forward propagation and backward propagation for training, and for storing we might have to store the weights and biases of all the layers.
The trained model can be stored in different formats to load later for serving and inference. Some popular formats in which to save a model are as follows:
- ONNX
- YAML
- Protobuf
- Pickle
- JSON
- H5
- TFJS
- Joblib
However, model-serving tools usually require the models to be saved in a particular format. So, they provide a function to save the model in its desired format. There are also tools and libraries to convert models from one format to another. For example, in Figure 1.2, we see that an AlexNet model that is pre-trained in PyTorch is loaded and exported to ONNX format in a file named alexnet.onnx
.
PyTorch files
It’s worth knowing that PyTorch saves the model using the Python pickle (https://docs.python.org/3/library/pickle.html) library. For further reading on PyTorch strategies for saving and loading models, please check out their official documentation: https://pytorch.org/tutorials/beginner/saving_loading_models.html.
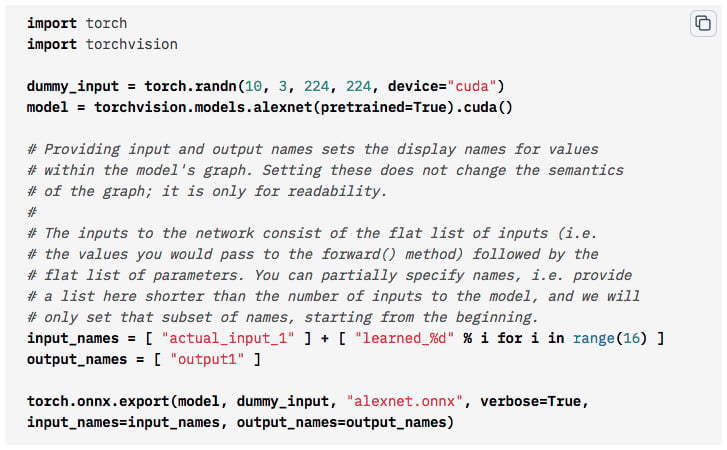
Figure 1.2 – Example code converting a PyTorch pre-trained AlexNet model to ONNX format
Note
Figure 1.2 is an example from the PyTorch official website: https://pytorch.org/docs/stable/onnx.html#example-alexnet-from-pytorch-to-onnx.
Now we should have a good idea about models and how each model is represented and stored. The following section will introduce us to model serving.