Data science project methodologies
When working on a large data science project, it's good to organize it into a process of steps. This especially helps when working as a team. We'll discuss a few data science project management strategies here. If you're working on a project by yourself, you don't necessarily need to exactly follow every detail of these processes. However, seeing the general process will help you think about what steps you need to take when undertaking any data science task.
Using data science in other fields
Instead of focusing primarily on data science and specializing there, one can also use these skills for their current career path. One example is using machine learning to search for new materials with exceptional properties, such as superhard materials (https://par.nsf.gov/servlets/purl/10094086) or using machine learning for materials science in general (https://escholarship.org/uc/item/0r27j85x). Again, anywhere we have data, we can use data science and related methods.
CRISP-DM
CRISP-DM stands for Cross-Industry Standard Process for Data Mining and has been around since the late 1990s. It's a six-step process, illustrated in the diagram below.
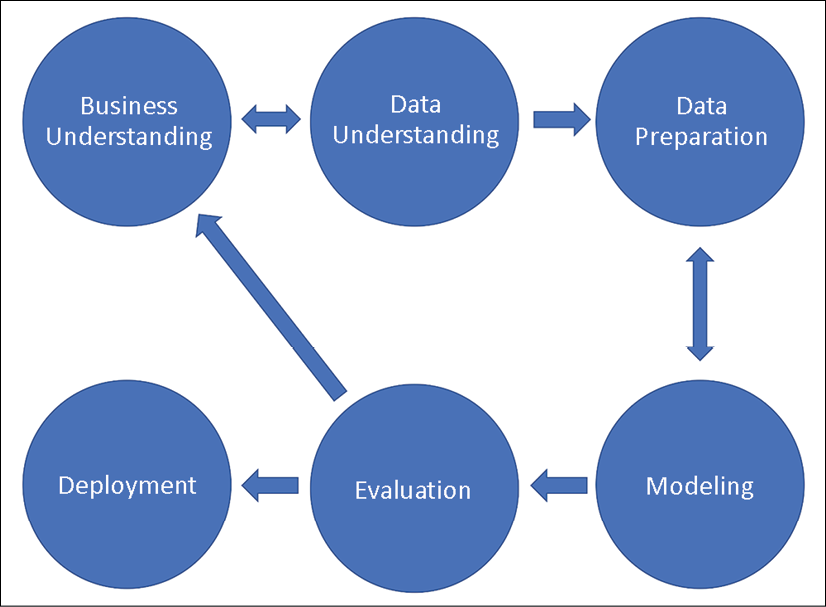
Figure 1.4: A reproduction of the CRISP-DM process flow diagram
This was created before data science existed as its own field, although it's still used for data science projects. It's easy to roughly implement, although the official implementation requires lots of documentation. The official publication outlining the method is also 60 pages of reading. However, it's at least worth knowing about and considering if you are undertaking a data science project.
TDSP
TDSP, or the Team Data Science Process, was developed by Microsoft and launched in 2016. It's obviously much more modern than CRISP-DM, and so is almost certainly a better choice for running a data science project today.
The five steps of the process are similar to CRISP-DM, as shown in the figure below.
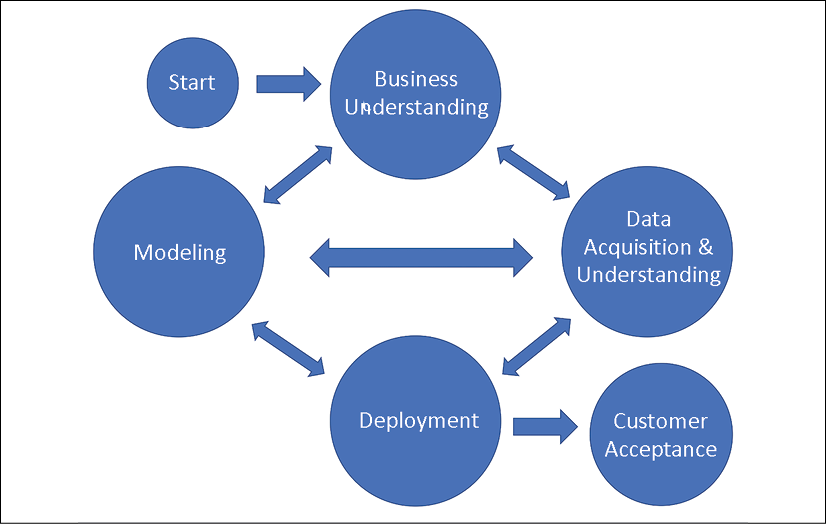
Figure 1.5: A reproduction of the TDSP process flow diagram
TDSP improves upon CRISP-DM in several ways, including defining roles for people within the process. It also has modern amenities, such as a GitHub repository with a project template and more interactive web-based documentation. Additionally, it allows more iteration between steps with incremental deliverables and uses modern software approaches to project management.
Further reading on data science project management strategies
There are other data science project management strategies out there as well. You can read about them at https://www.datascience-pm.com/.
You can find the official guide for CRISP-DM here:
https://www.the-modeling-agency.com/crisp-dm.pdf
And the guide for TDSP is here:
https://docs.microsoft.com/en-us/azure/machine-learning/team-data-science-process/overview
Other tools
Other tools used by data scientists include Kanban boards, Scrum, and the Agile software development framework. Since data scientists often work with software engineers to implement data science products, many of the organizational processes from software engineering have been adopted by data scientists.