Preparing, building, training and tuning, deploying, and managing ML models
First, let's review the ML life cycle. By the end of this section, you should understand how SageMaker's capabilities map to the key phases of the ML life cycle. The following diagram shows you what the ML life cycle looks like:
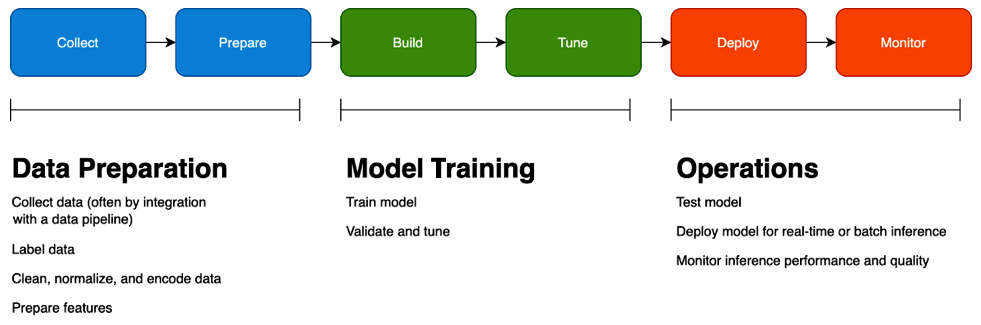
Figure 1.1 – Machine learning life cycle
As you can see, there are three phases of the ML life cycle at a high level:
- In the Data Preparation phase, you collect and explore data, label a ground truth dataset, and prepare your features. Feature engineering, in turn, has several steps, including data normalization, encoding, and calculating embeddings, depending on the ML algorithm you choose.
- In the Model Training phase, you build your model and tune it until you achieve a reasonable validation score that aligns with your business objective.
- In the Operations phase, you test how well your model performs against real-world data, deploy it, and monitor how well it performs. We will cover model monitoring in more detail in Chapter 11, Monitoring Production Models with Amazon SageMaker Model Monitor and Clarify.
This diagram is purposely simplified; in reality, each phase may have multiple smaller steps, and the whole life cycle is iterative. You're never really done with ML; as you gather data on how your model performs in production, you'll likely try to improve it by collecting more data, changing your features, or tuning the model.
So how do SageMaker capabilities map to the ML life cycle? Before we answer that question, let's take a look at the SageMaker console (Figure 1.2):
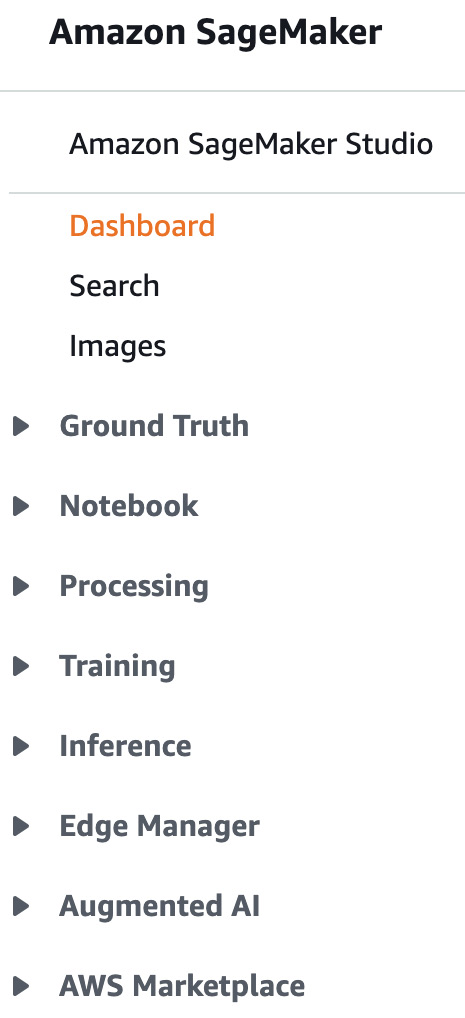
Figure 1.2 – Navigation pane in the SageMaker console
The appearance of the console changes frequently and the preceding screenshot shows the current appearance of the console at the time of writing.
These capability groups align to the ML life cycle, shown as follows:
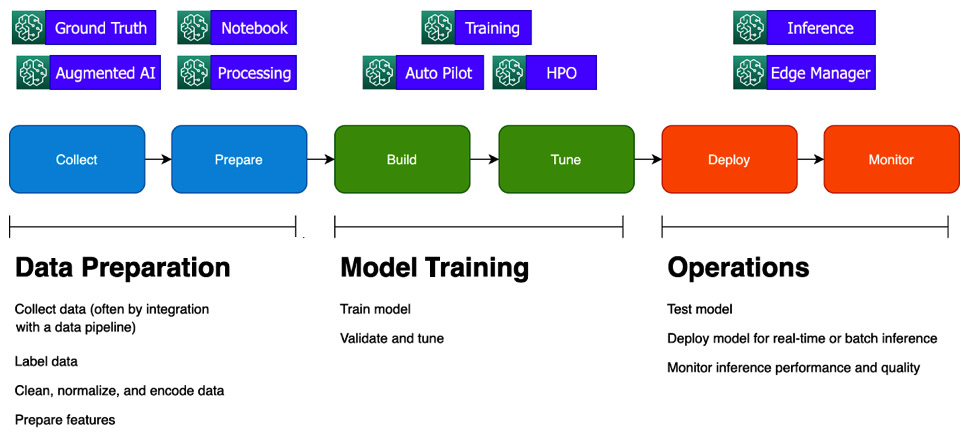
Figure 1.3 – Mapping of SageMaker capabilities to the ML life cycle
SageMaker Studio is not shown here, as it is an integrated workbench that provides a user interface for many SageMaker capabilities. The marketplace provides both data and algorithms that can be used across the life cycle.
Now that we have had a look at the console, let's dive deeper into the individual capabilities of SageMaker in each life cycle phase.