There is a lot of interest in using deep learning at the edge. This is the application of deep learning to compute solutions on or near the devices that capture data using sensors and cameras. An alternative solution to deep learning at the edge is to capture edge data and send it to in the cloud for processing. But, deep learning at the edge has the advantage of lower latency and higher security. Devices at the edge are typically cheap, have a small form factor and use less power, and their processors or accelerators have less compute capability. One of the key advantages of Caffe2 is that it has been designed and developed from the beginning to scale: from multi-GPU, multi-CPU servers, down to tiny edge devices. In this section, we will use the Raspberry Pi as an example of an edge device and learn how to use Caffe2 on it.
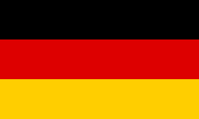
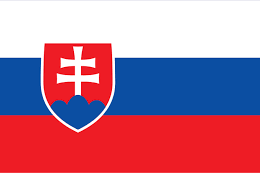
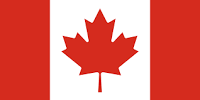
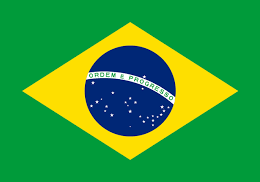

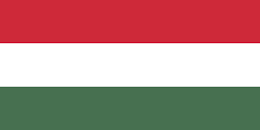
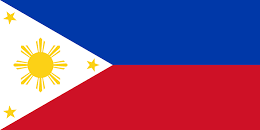
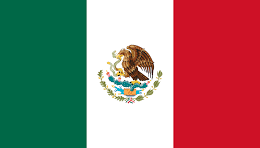
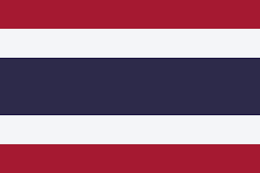
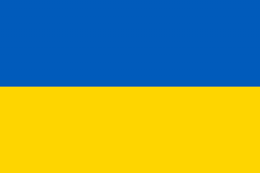
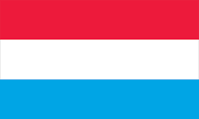
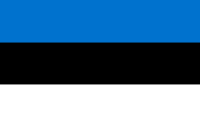
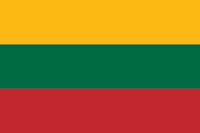
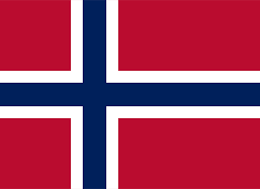
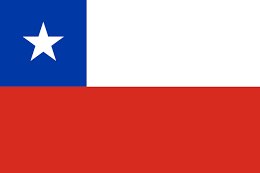
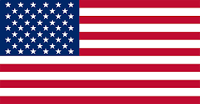
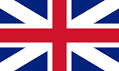
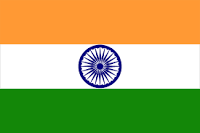
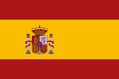
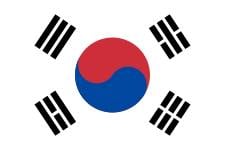
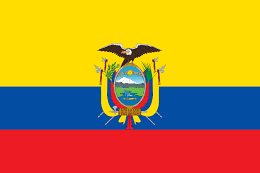
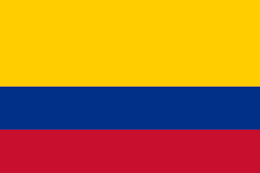
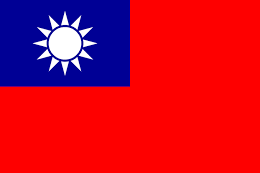
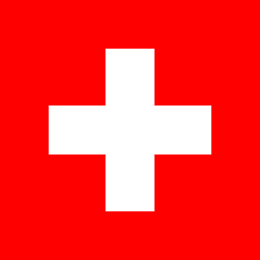
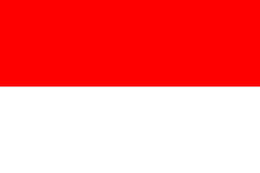
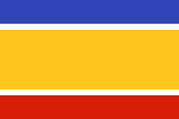
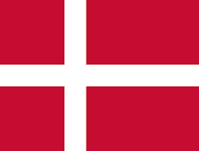
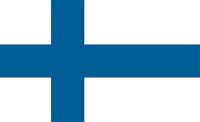
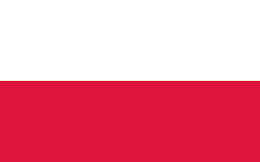
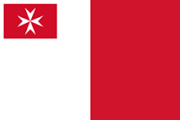
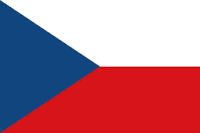
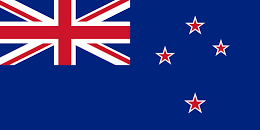
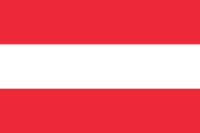
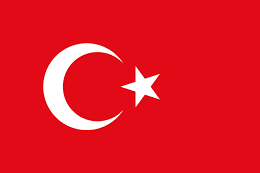
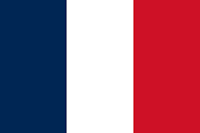
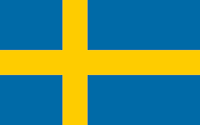
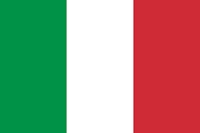
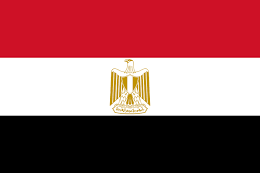
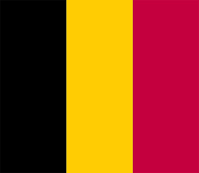
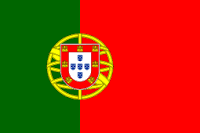
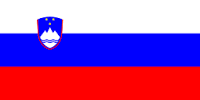
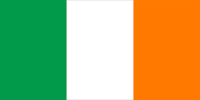
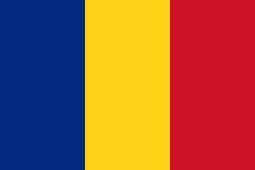
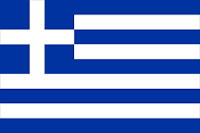
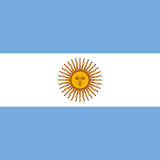
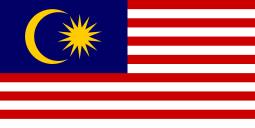
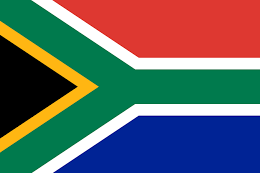
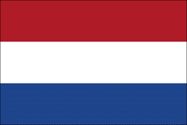
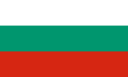
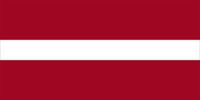
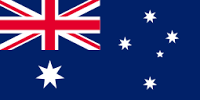
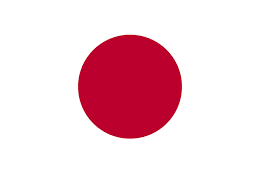
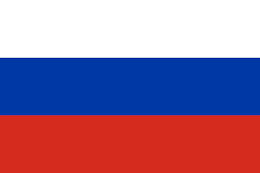