Time series data – characteristics and components
There are many uses for data that include a time component, be it minutes, days, months, or seasons. Some uses are for forecasting demand for products that are influenced by seasonality, or for predicting maintenance schedules for manufacturing equipment. Data in the form of measurements taken at intervals indicated by a date or time value is known as time series data. This type of data can present trends where values are rising or falling over time, seasonality where there is a repeating pattern by the time of year, cyclical patterns, or even random unpredictable variation. Consider the following line plot (created by the author) as an example:
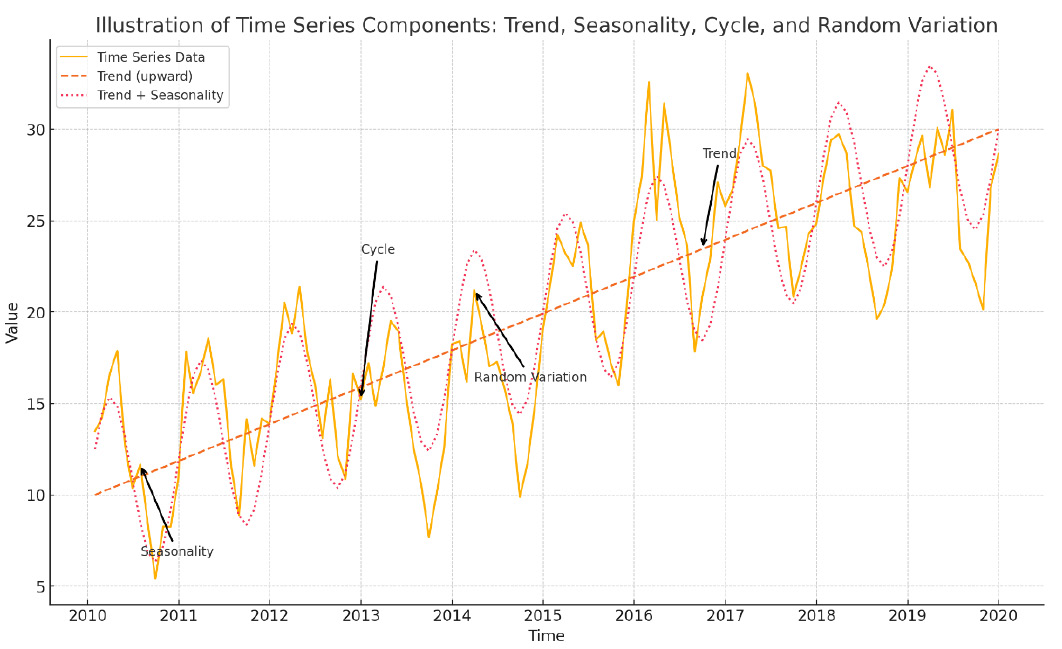
Figure 9.1 – A line plot with time on the x-axis (spanning multiple years) and a sample metric (such as sales or temperature) on the y-axis
As demonstrated in the preceding figure, there are four key components to the time series:
- Trend: A...