Generalized linear regression
Recall that in linear regression, we assume the following functional form between the dependent variable Y and independent variable X:
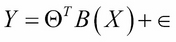
Here, is a set of basis functions and
is the parameter vector. Usually, it is assumed that
, so
represents an intercept or a bias term. Also, it is assumed that
is a noise term distributed according to the normal distribution with mean zero and variance
. We also showed that this results in the following equation:
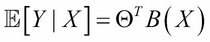
One can generalize the preceding equation to incorporate not only the normal distribution for noise but any distribution in the exponential family (reference 1 in the References section of this chapter). This is done by defining the following equation:

Here, g is called a link function. The well-known models, such as logistic regression, log-linear models, Poisson regression, and so on, are special cases of GLM. For example, in the case of ordinary linear regression, the link function would be . For logistic regression...