PyCharm as a Python IDE
It’s all well and good to talk about tool comparisons common to other languages. But we don’t care about that, do we? We want to know our options for Python! The best IDEs are typically specialized. PyCharm is specialized in working with Python. That’s it. When you create a new project in PyCharm, you’ll see options for Python projects and nothing else. Contrast this experience with Visual Studio. In my opinion, Visual Studio is the only close competitor to PyCharm when it comes to working with Python projects. When a project is created in Visual Studio, you will most likely spend a good five minutes trying to wade through the myriad of options. The IDE supports dozens of languages, and that is compounded by a dozen project types such as web, desktop, and others. Visual Studio is trying to be all things to all developers. PyCharm only wants to play with Python developers.
PyCharm itself was created with a few design goals in mind:
- Intelligent coding assistance
- Streamlined programming tools
- Web development options
- Scientific computing support
- Visual debugging and performance profiling
We’ll take a look at each of these design goals in turn, but first, I need to point something out. At the time I’m writing this, PyCharm is about to go through a big change. JetBrains is working on a brand-new user experience. By the time this book is published, there is a strong chance that this new UI will be the default. If you’re new to PyCharm, you should understand that you’re going to see it in two different ways for a while. The classic UI will continue to be available in the product for a time, allowing us to ease into the new experience. I’ve decided I’m going to embrace the new UI given the time between the first edition and this one is a few years. That said, it bears mentioning that you’re going to see the classic UI alongside the new UI until probably late 2024 when the old UI is no longer maintained. It will become deprecated and one day will disappear into the sands of time like Shelley’s fabled statue of Ozymandias:
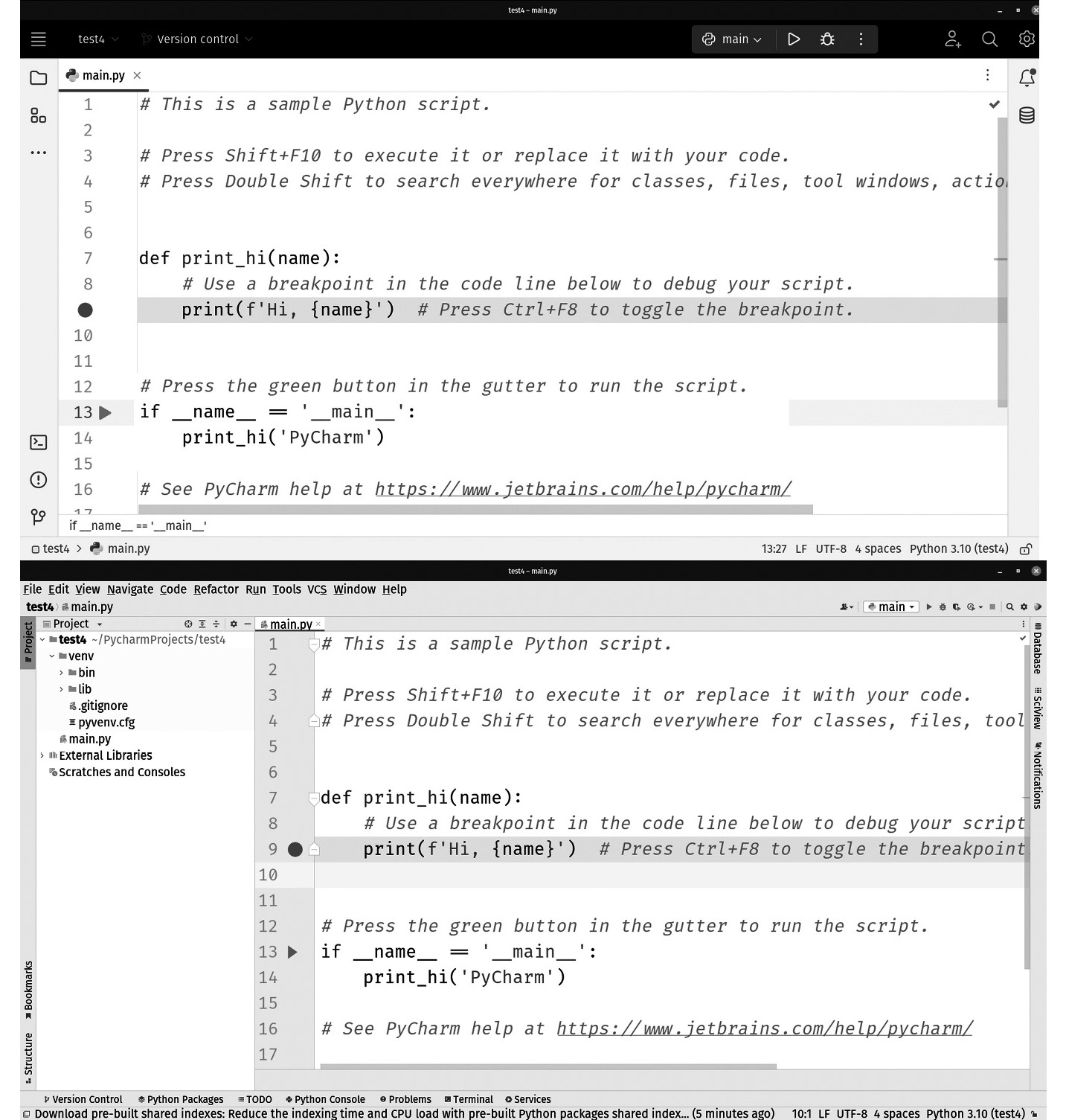
Figure 1.4: The new UI (top) compared to the classic UI (bottom)
Figure 1.4 shows the two UIs side by side. The design objective of the new UI is to reduce the clutter in the interface. They’re not wrong on that point. As the tool has grown over the years, more and more features have been crammed into the menus, making the UI a little bit daunting for new users. The biggest thing to realize is that most of the things you’d find in the menu are still there, but the menu system itself is hidden beneath the hamburger icon in the top-left corner of the screen. Don’t worry; I’ll cover this in detail later. As I write this, there is a setting we’ll review in Chapter 2, Installing and Configuring PyCharm, that allows you to toggle between the classic and new UIs.
I wanted to point this out now because you’re about to see some screenshots, and if you’ve seen the old UI, you might think you’ve picked up the wrong book. You haven’t. Just the opposite. If I time this right, you’ll be the only one with the right book.
Intelligent coding assistance
I’m going to tell you something my wife says all the time. I’m very lazy. Wait. That came out wrong. She is saying that I’m lazy. I’m not saying that she is saying she is lazy. Sheesh. Writing is hard! I almost dug a hole there, didn’t I?
She’s not wrong. As a developer, I am essentially very lazy. I refuse to spend hours or even minutes doing something the long way. The Greeks had a legend about a guy named Sisyphus who was cursed to push a stone up a steep hill. As soon as he reached the top, the stone would roll back down the hill. Sisyphus was stuck in an infinite loop with no Cmd/Ctrl + C option on his keyboard.
Here’s one thing I know: Sisyphus was not a software developer! Any software developer would have rolled that stone exactly twice, after which they would have spent eternity devising a system of pulleys and cranes controlled by an IoT device. Naturally, the microcontroller would be running a Python script. I digress.
What some (I say in my head looking in my unsuspecting wife’s direction) might call lazy, I call efficient! As a developer, I want to create maximum effect with minimal effort in everything I do. Writing code is complicated. You are writing instructions for the most stubborn and unintelligent object ever devised. Coding is worse than trying to teach a 2-year-old to tie their shoes. Trust me, I’ve done both! Coders must be extremely specific and verbose in their explanation of any operations they want to perform. Furthermore, things are made worse by the language developers out there who tend to want to force users to write a bunch of boilerplate code. I’m talking about the excise code that has nothing to do with the code you want to write or the problem you want to solve. Python generally avoids this, so let me give an example of possibly the worst offender: Java.
Back in the day when I was a wee lad, Java was all the rage. There was this caste of corporate programmers involved with Java who thought up something called Enterprise Java Beans (EJBs). EJBs were supposed to be the epitome of module programming with reusable objects. It was an absolute beating to implement. Rather than simply making a class, which is all you need, you had to create a special file structure with various folders and manifest files to expose what was in the bean, and it was all compiled into a special format. It turned out that the special format was nothing more than a ZIP file. It took a lot of work just to make an EJB, which meant developers had to make a ton of files and write a lot of code just to get started on the functionality they needed to express to get their work done. That’s what we mean by boilerplate. Boilerplate is generally useless but necessary because, without it, the code doesn’t work.
All IDEs have evolved because of this phenomenon. PyCharm evolved from JetBrains’ Java IDE, IntelliJ. Python doesn’t usually have a lot of boilerplate required for your code to work, but it does come up. There are two kinds of boilerplate. The boilerplate needed to make old-school EJBs work is the bad kind. The boilerplate generated as a means to jumpstart your project is the good kind. As we’ll see, PyCharm, as with most IDEs, generates a folder structure, a set of files, and some basic code to get you started. That can be considered boilerplate. But in this case, that code isn’t retained. It is replaced by the real code for your project. The code generated by the IDE is just a mental prompt to get you going. It prevents you from having to create your project’s starting point by hand.
All this is great, but boilerplate code generation isn’t what we usually think about when we hear “intelligent coding assistance.” We usually think of the feature pioneered by Microsoft called IntelliSense. If you’ll allow me to anthropomorphize the IDE for a moment, this feature watches as you type your code. All the while, the IDE is thinking about what you’re trying to do. When it sees a way it can help, such as by completing a word or line for you automatically, it presents that as an option. I have an intelligent person completing all my sentences for me: she’s my wife. When she completes my sentences for me, they are usually more organized and intelligent than they would have been if I were on my own. (This might be another reason she thinks I’m lazy.)
I want to point out that not all tools with an IntelliSense-like feature are created equally. When you see this feature in an enhanced editor, it usually works differently than it would in an IDE. In enhanced editors, they use keyword lists to highlight and autocomplete the elements of a language. Really good enhanced editors might index your code and recognize variable and function names and use statistics to give you the most likely completion first. That option is generally followed by a long list of noise comprising every possibility that exists for a given completion. Code completion is becoming very advanced with the introduction of AI tools, and this makes the difference between IDEs and enhanced editors a little muddier, at least on this point. Tools such as GitHub’s Copilot can not only autocomplete variable names and keywords but also write entire sections of your code automatically.
It is important to remember, at least as I write this, that those AI features are not part of the IDE or enhanced editor. They are implemented as plugins. Since this is true, I’ll continue espousing the merits of IDEs, and PyCharm in particular, based solely on the merits of the software by itself. We’ll discuss plugins in Chapter 16, More Possibilities with PyCharm Plugins.
While enhanced editors might present you with a long list of possibilities for your code completion, PyCharm can analyze your code and perform more intelligent autocompletion. You also get code analysis, such as duplicate code warnings. A common antipattern in software development is copying and pasting code across or even within the same project. It’s a terrible but common thing to see. PyCharm will spot duplicate code and flag it for you so that you can be reminded to refactor the duplicated code into a function or module that can be reused and maintained in one location.
PyCharm can also perform a static analysis of your code. This type of analysis is looking for antipatterns within the code itself; for example, PyCharm will detect dead code like that shown in Figure 1.5. Concerning Python development, PyCharm will automatically format your indentations and give you critical feedback on how your code conforms to PEP-8 conventions, which are stylistic requirements you must meet to be considered pythonic (that’s a good thing).
For example, if you were to type the following code into a new file in PyCharm, you’d see PyCharm’s warning that you have created unreachable code on line 13. The text on that line is highlighted. Hovering your mouse over this highlighted line reveals what is wrong:
def print_hi(name): print(f'Hi, {name}') for x in range(25): print(str(x)) if x == 12: return print("You'll never make it here") if __name__ == '__main__': print_hi('PyCharm')
The print_hi
function starts innocuously enough by printing to the console whatever is passed into the function within the name
argument. After that, we create a loop that will run 25 times. On each run of the loop, we print out x
, which contains the current iteration. When the counter variable, x
, reaches 12, the loop exits via the return
function, which, as luck has it, is on line 12. I assure you, this is purely a coincidence. Since the loop returns on line 12, the code on line 13 will never be reached:
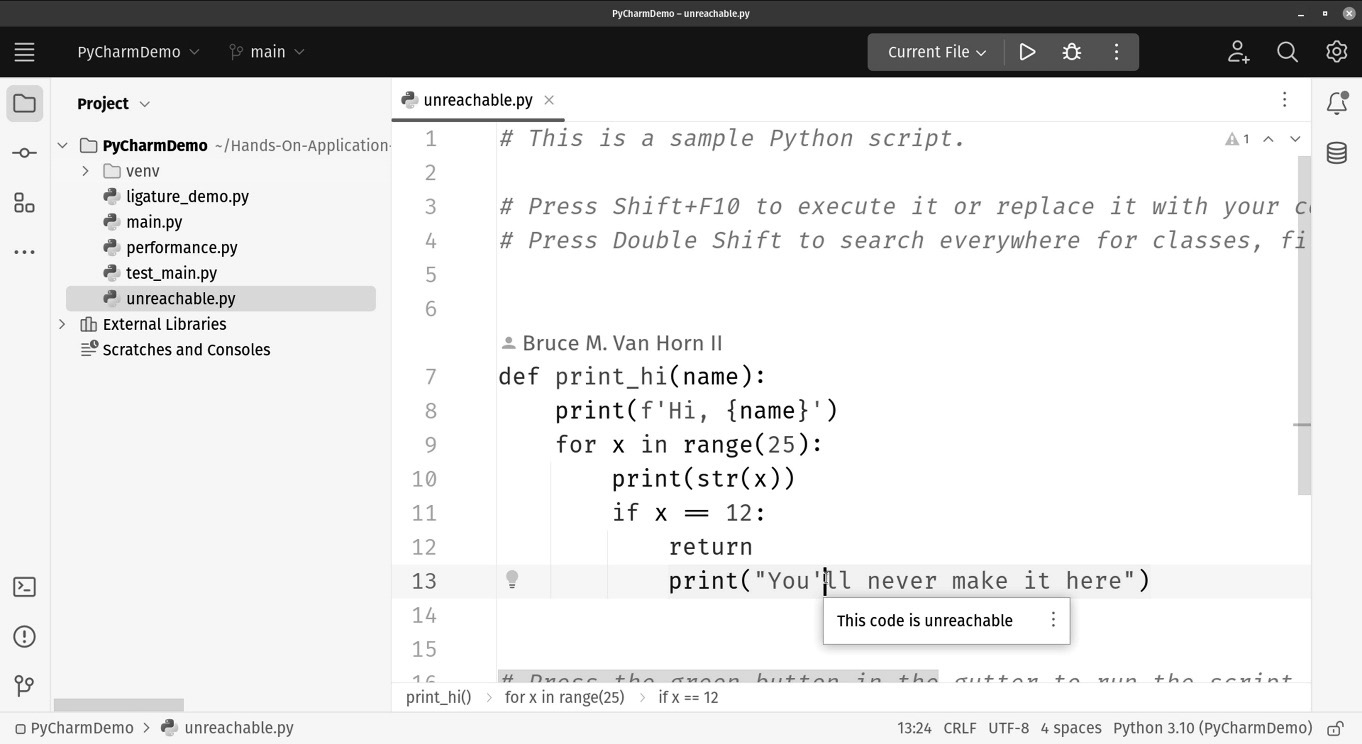
Figure 1.5: PyCharm will highlight many common coding mistakes such as this one. The code on line 13 is unreachable, which is indicated when you hover your mouse over the highlighted code
PyCharm also allows you to navigate between files in a complex project by helping you find where functions, variables, and classes are defined, as well as where they are used. Over time, you’ll learn a set of keystrokes that will allow you to move anywhere in your project without your fingers leaving the keyboard.
In essence, PyCharm’s intelligent coding assistance allows you to worry less about mistakes and more about your logic and requirements, which allows you to complete your code more quickly with fewer mistakes.
Streamlined programming tools
Writing code is but one activity a developer performs each day in pursuit of a project deadline. Great developers also spend time debugging, testing, and profiling their products to produce the best possible result. We also need to deal with pushing code to testing servers, refactoring (other people’s) bad code, working with databases, and dealing with containers. There is tooling in PyCharm for each of these processes and more. When I write complicated web applications in PyCharm, the only tools I usually have open are PyCharm and a web browser: two tools each on their own monitor.
The PyCharm debugger
My favorite feature and the one that got me excited the first time I used PyCharm is the debugger. PyCharm’s debugger is great. It is much better than the standard debugger you get with Python itself. Python ships with a debugger called Python Debugger (pdb). In my humble opinion, I’d rather eat bugs off the sidewalk than use this tool. I alluded to this earlier in this chapter. I grew up using Microsoft debuggers and simply nothing else will do. PyCharm’s debugger works exactly as I would expect. Click your mouse at the line where you want execution to stop to make a breakpoint and click the debug button in the IDE, and the program runs and stops at the indicated line. You will get a screen where you can inspect both the state of the stack as well as the terminal output. It’s very simple to use, and I’ll be showing you how to do so in Chapter 6, Seamless Testing, Debugging, and Profiling.
Running tests with the graphical test runner
Testing tools are integrated in the form of test runners. PyCharm supports all the major testing frameworks, including pytest, nose, and the regular unit test features from the standard library. Again, I’m coming from experiencing some very good IDEs, and in this case, I’m remembering Eclipse and Visual Studio, which both include graphical test runners. The adage If the bar is green, the code is clean is visually implemented in PyCharm. You can see an example in Figure 1.6. You can run your tests and see a list display showing what passed and what failed, though it is a list rather than a bar. You can then rerun your failing tests until they work.
I’ll give you a simple example. In main.py
within this chapter’s source code, I have one file called main.py
and another called test_main.py
. The content of main.py
is a simple function that adds two numbers together:
def add_two_numbers(a: int, b: int) -> int: return a + b
Within the test_main.py
file, there is a simple unit test:
from unittest import TestCase from main import add_two_numbers class Test(TestCase): def test_add_two_numbers(self): self.assertTrue(add_two_numbers(a=5, b=6) == 11, \ "Should be 11") def test_show_a_fail(self): self.fail()
The Test
class contains two tests: one that will pass and one that will automatically fail. I usually make the automatically failing test first just to make sure I have my test class set up properly. Then, later, I remove the fail because of my dopamine addiction, which is only satisfied by green checkmarks in the test runner, as seen in Figure 1.6. If I right-click on test_main.py
, as seen in Figure 1.5, I’ll get an option to run the tests within the file:
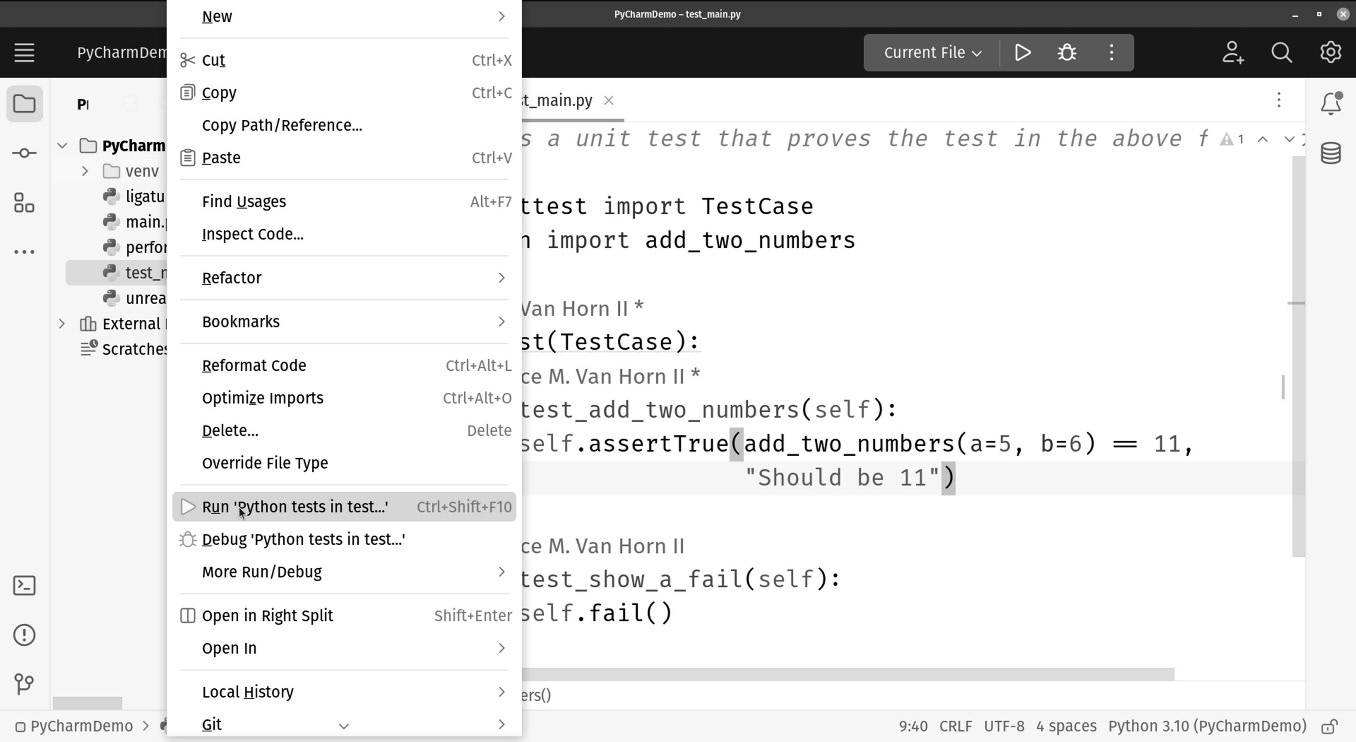
Figure 1.6: Right-click the test_main.py file and click Run ‘Python tests in test…’ to run the unit tests contained within the file
Look to the lower-left corner in Figure 1.7, which shows the test run’s completion, and you’ll see a list of tests that passed or failed with either a green check or a yellow X showing failure. Like all figures in this book, which are printed in black and white, you won’t see the colors. Color ink is expensive, and your father is right, money doesn’t grow on trees:
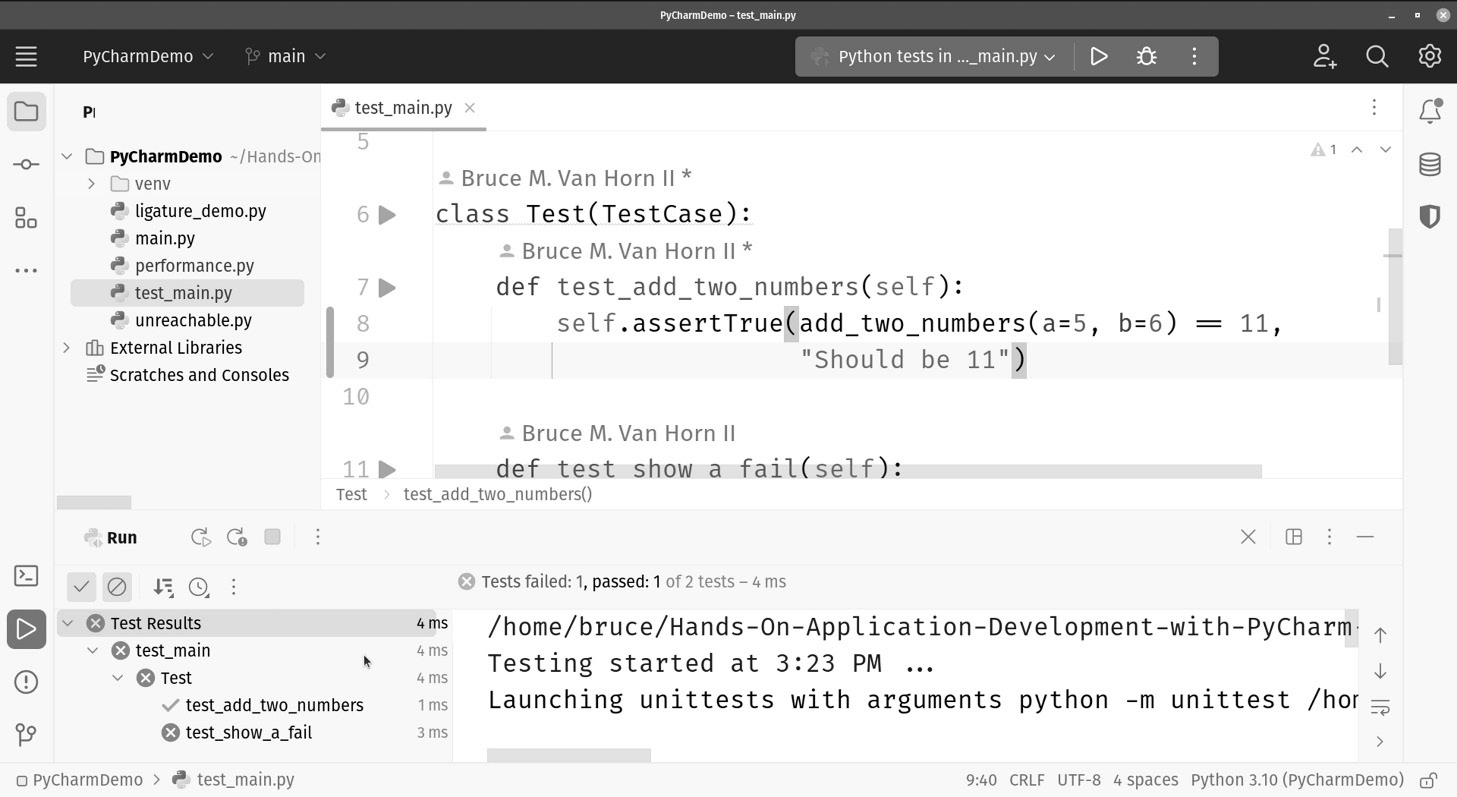
Figure 1.7: PyCharm’s built-in test runner shows a traditional pass/fail list (lower-left pane) to indicate passing and failing tests
PyCharm’s profiling tools
Similarly, code profiling is built in and easy to use. You can click the Profile button to run the code. When the program exits, you will get a graph of each function call, along with the time and resources consumed by the call. This makes it easy to spot unrealized opportunities for improvement concerning the speed of execution and resource consumption.
Consider the possibility that you have an algorithm in your program that might not be performing as you’d like. I know, I know, it would never happen to you, so supposed you just got hired, and the person they fired wrote this horribly performing algorithm. Maybe pretend it is 1956, and the guy who got fired from your new employer, New York Life Insurance Company, was one Edward Harry Friend. Friend wrote a paper titled Sorting on Electronic Computer Systems, which is likely the first published instance of an algorithm we know today as bubble sort. If Friend had written his algorithm in Python 3, it might look a little like this:
def bubble_sort(input_array): length_of_array = len(input_array)
Friend has just created a function that accepts a list as an argument, which in our case will be an array of integers. The objective is to sort these numbers. To do this, let’s create two loops, one inside the other:
for i in range(length_of_array): for j in range(0, length_of_array - i - 1): if input_array[j] > input_array[j + 1]: input_array[j], input_array[j + 1] = \ input_array[j + 1], input_array[j]
Within these loops, each number is compared with the number before it. If it is determined those two numbers are out of order, they are swapped. In the next run of the loop, this happens again with the next two numbers, and this continues until it reaches the end of the list.
If you’ve studied algorithms at all, you’ve probably heard of bubble sort, and you’ve been warned as to why it is not used. It is very slow. We have a for
loop within another for
loop, which is fine if the size of your unsorted list is small. But this algorithm slows down at a logarithmic rate as the list of numbers grows. Algorithm performance is measured using big O notation. I don’t want to turn this into an algorithm book, so I’ll just tell you that a loop inside another loop will scale poorly in terms of performance. In big O notation, we classify this algorithm as O(n2). That’s bad.
In plain English, this means that if you double the count of numbers to sort (n), then your algorithm will take 22 or 4 times longer to process. If you multiply your count’s size by 5, then it becomes 52 or 25 times slower. The bigger the list, the slower it sorts.
To show off the performance tool, we’re going to give this test run a list of 100,000 numbers to sort. Now is a good time to point out I’m running an Intel i9 processor. If you’re a student or some other budget-constrained consumer rocking an i3 processor (or worse), you might want to take the list of numbers down a few zeros if you want to try this out. It takes a good while on my i9:
test_array = [] for x in range(100000): test_array.append(random.randint(1, 10000))
Let’s finish the test code by calling the function and printing the results:
bubble_sort(test_array) print("The result of the sort is:") for i in range(len(test_array)): print(test_array[i])
We’ll cover the profiling tool extensively in Chapter 6, Seamless Testing, Debugging, and Profiling, but for now, let’s just run this code with the profiler and review the result. To run the profiler on the performance.py
file, simply right-click the file and click More Run/Debug, then Profile ‘performance’, as shown in Figure 1.8:
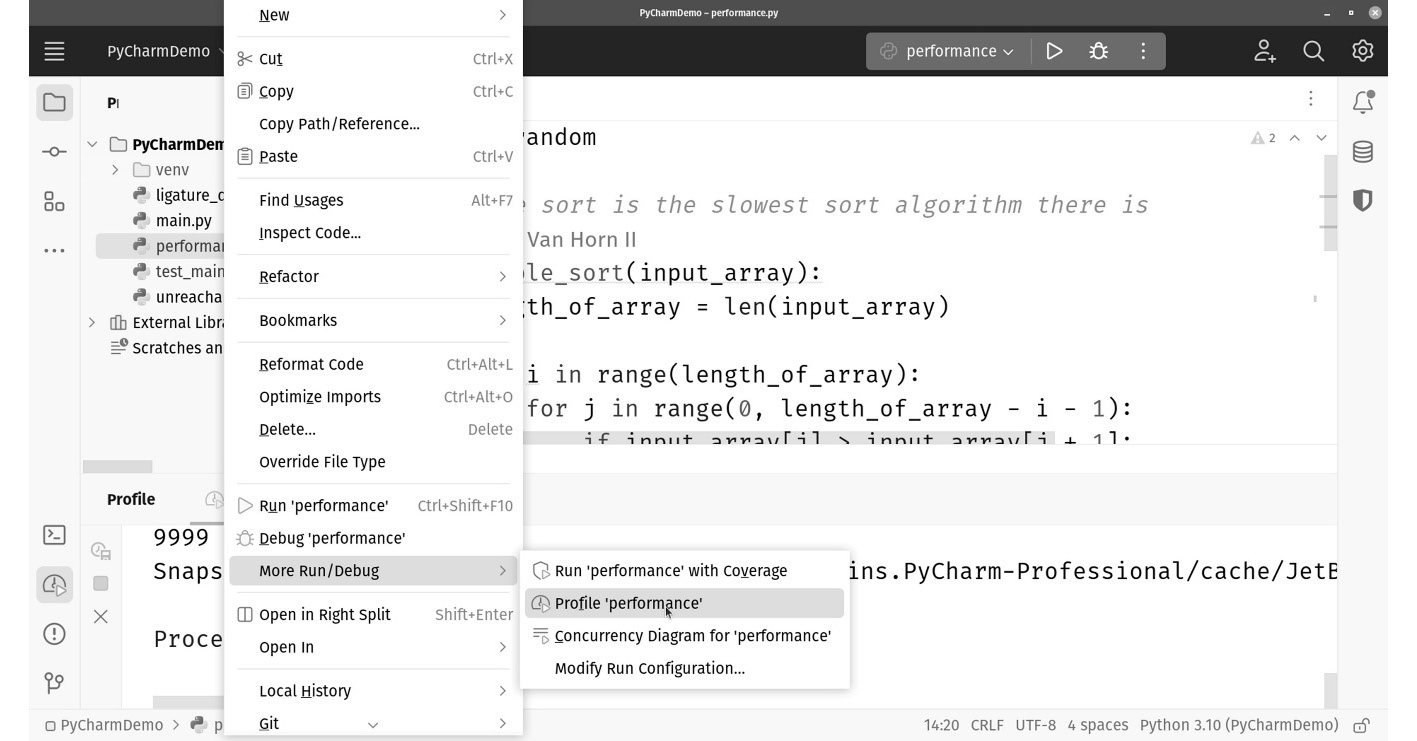
Figure 1.8: Right-click the file you’d like to profile and click More Run/Debug | Profile ‘performance’ to see a performance profile
Remember, if you use the same code I did, this will take a long time to run, especially on a slower computer. Feel free to adjust the size of the list downward if it’s taking too long to run. The result is a .pstat
file that displays as a table in PyCharm. Again, we’ll cover this more extensively in Chapter 6, Seamless Testing, Debugging, and Profiling. You can see the performance report in Figure 1.9:
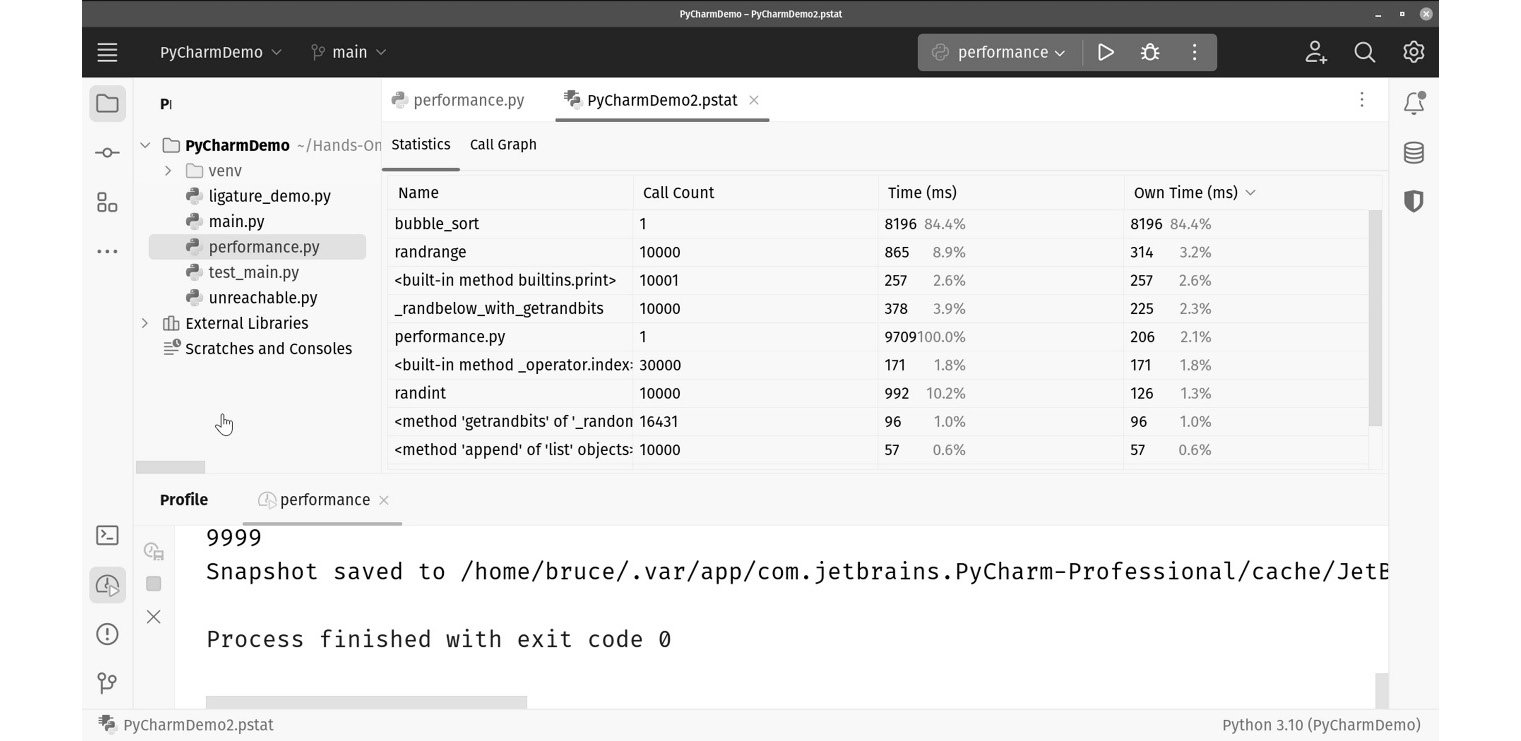
Figure 1.9: PyCharm’s resource profiler shows the performance bottlenecks in a running program
As you can see, 84.4% of the program’s time is spent in the bubble_sort
function, which is the bottleneck. PyCharm has told you where to concentrate your refactoring efforts to improve the performance of your program.
Publishing from the IDE
When you need to publish your code to a testing server, and you aren’t using a continuous integration system for that, you can use PyCharm. To be clear, you should use a continuous integration system, but I often use the PyCharm features early in a project before the continuous integration system is operational to get code up for stakeholders to play with. You can deploy using file transfer protocol (FTP) or secure file transfer protocol (SFTP), or copy directly to a network share for a quick and easy way to share your progress with anyone who might want to review it.
Refactoring tools
PyCharm has robust refactoring tools you’d expect from a proper IDE. If you want to change a variable name, or even a method signature on a function, right-click and select the Refactor tool. Rest assured the changes you make will carry over to all related instances in your project, not just in the file you are editing. Figure 1.10 shows an example of this in action:
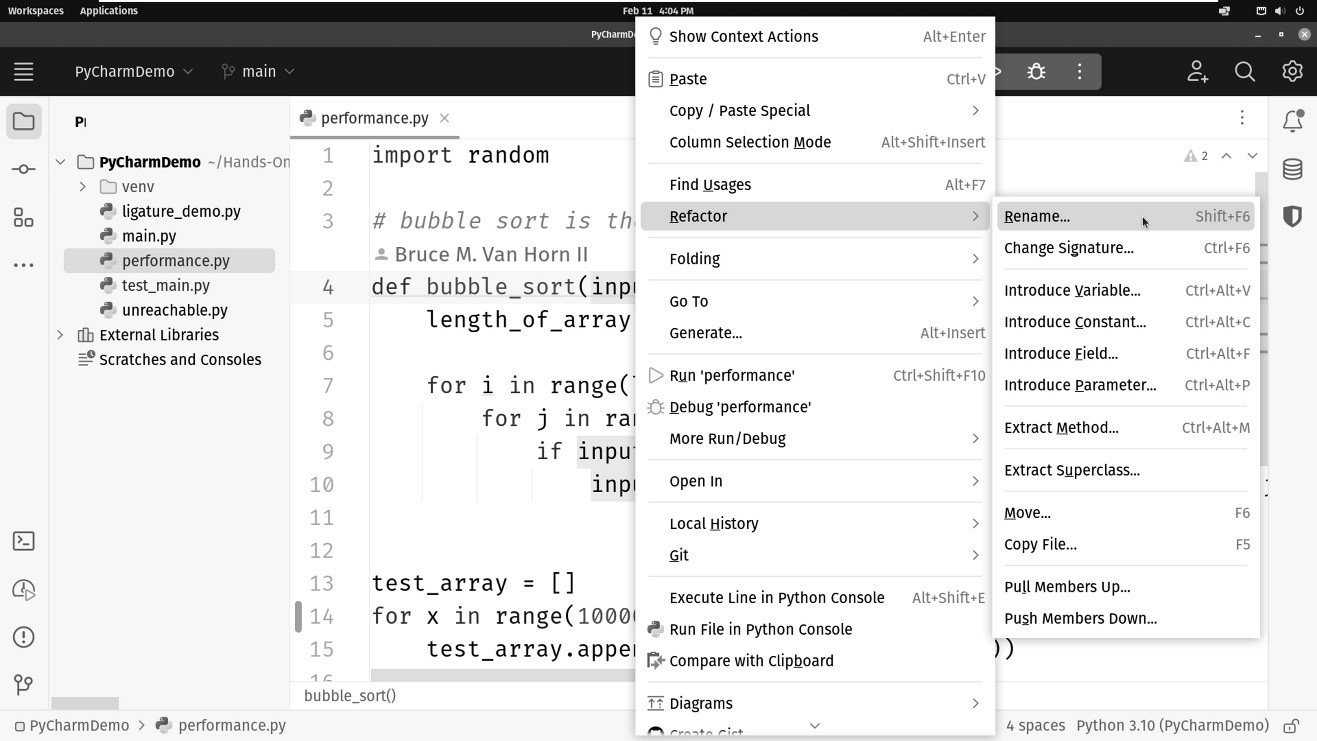
Figure 1.10: PyCharm has a full selection of refactoring tools available
In addition to renaming a variable or function, there are other actions you can perform, such as changing the method signature and changing the structure of your object-oriented classes.
Working with databases in PyCharm
If you work with databases, the Professional edition of PyCharm includes a graphical table editor and SQL support for dozens of popular databases. Check it out in Figure 1.11. I’ll talk more about the Professional edition of PyCharm a little later in this chapter; we’ll have a whole chapter on the database features in Chapter 11, Understanding Database Management with PyCharm:
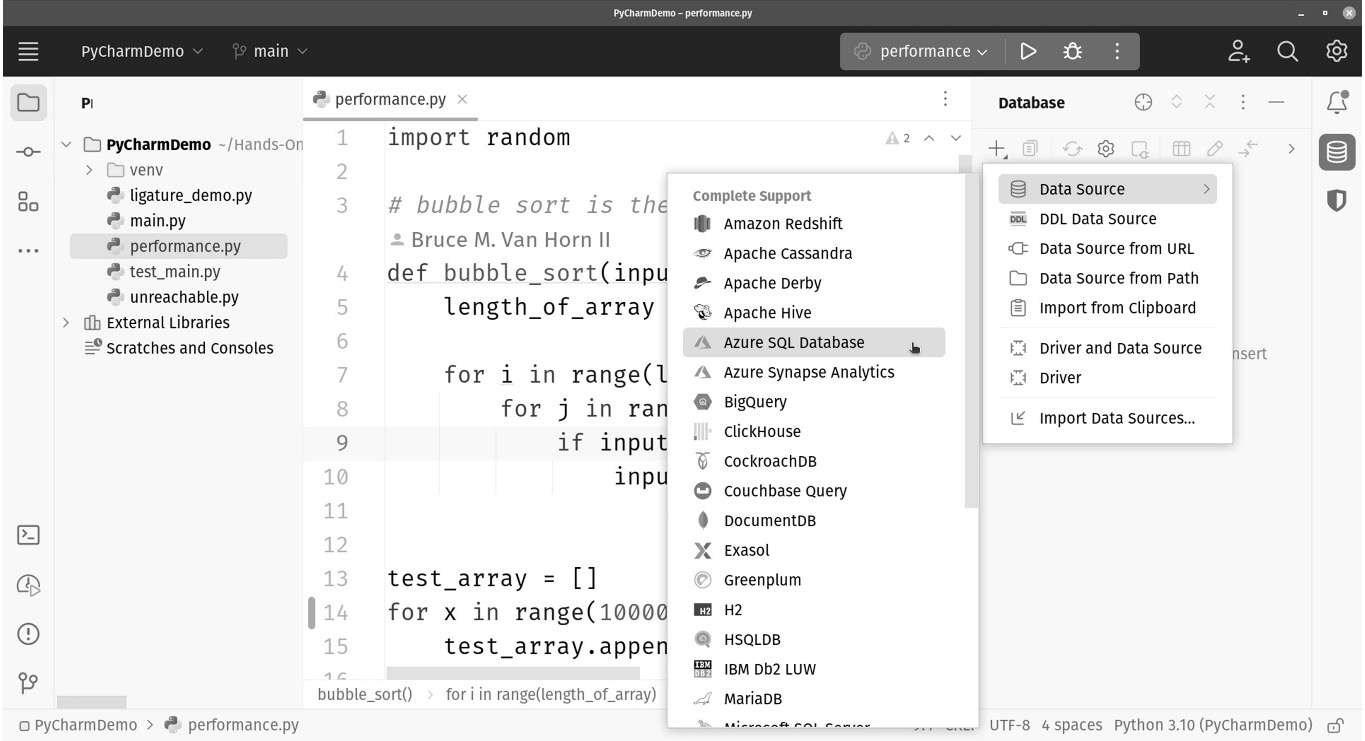
Figure 1.11: PyCharm has a robust and complete set of tools for working with relational databases such as Oracle, SQL Server, Postgres, and many more
As you can see, a great many relational and NoSQL databases are directly supported.
Remote development, containers, and virtual machines
Finally, but not exhaustively, PyCharm has features for working with remote systems via SSH, local virtual machines using HashiCorp’s Vagrant, and extensive support for Docker containers.
This isn’t an exhaustive list of what PyCharm can do for you, but by now, you get the point. Every tool you might need is integrated into the development environment. That’s probably why they called it an integrated development environment.
Web development options
I’d wager that more than half the developers working in Python need, at some point, a web project. Whether you’re like me and you’re making a SaaS offering as a fully realized web application, or you are doing rocket science and you need a way to visualize and interactively share your latest fast-Fourier transform on deep space radio emissions data, web projects are usually inevitable. I’m not a scientist and I made that up. If the last sentence made sense to anyone, it was pure coincidence.
Working with web projects offers a new and separate layer of complexity. Most use three-tier designs commonly expressed with the model-view-controller (MVC) pattern. If you’re not sure what this means, stay tuned because there’s a whole section of this book dedicated to web development. For now, this means the application has a frontend where the user can interact, a middle tier containing connective logic, and a database tier for structured data storage and retrieval. Only the middle tier is done in Python. We’ll extensively cover web development in later chapters, but for now, I want to tell you about the level of tooling you get with PyCharm.
The company that made PyCharm, JetBrains, makes a variety of IDEs targeted at different languages. One of their IDEs is specifically targeted toward web development. It is called WebStorm. I said earlier that good IDEs target one language. WebStorm targets JavaScript; specifically, we’re talking about full stack JavaScript. Modern JavaScript execution occurs in two places. Traditionally, JavaScript was always executed in the browser. About 10 years ago, Node.js was released and JavaScript was released from the confines of the browser window and allowed to run on the backend.
Earlier, I alluded to a robust set of features in PyCharm for working with databases. JetBrains also has an IDE that targets SQL database developers called DataGrip. As it happens, the Professional edition of PyCharm includes the entire feature set available in WebStorm and DataGrip. When you buy the Professional edition, you’re getting three JetBrains products in one package: PyCharm, WebStorm, and DataGrip. When you use PyCharm to work on web projects, you need all three feature sets and they are there for you in the Professional edition.
Scientific computing support
The growth of the data science field has played an important role in the growth of Python itself, and Python is now the most common programming tool used in scientific projects (even more common than R). Notable functionalities included in PyCharm that facilitate data science work are the integration of IPython, Jupyter notebooks, and an interactive console. The support for scientific computing in PyCharm is detailed in section four of this book, starting from Chapter 13, Turning On Scientific Mode. PyCharm also provides a customized view that optimally organizes workspaces in a scientific project called SciView, which is shown in Figure 1.12:
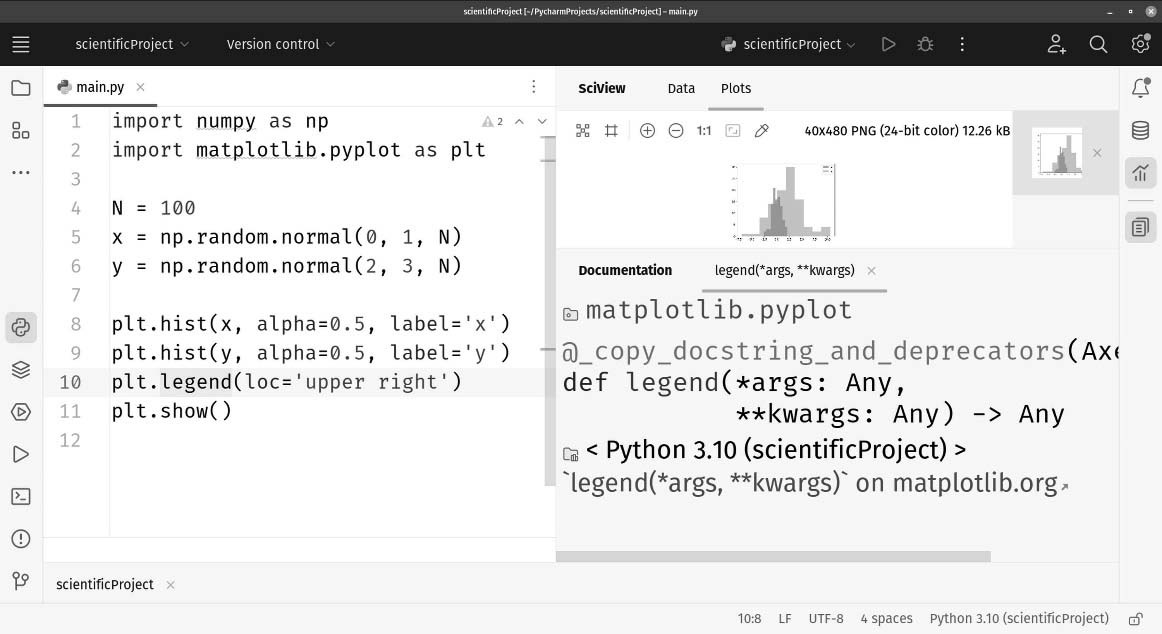
Figure 1.12: SciView in PyCharm grants access to scientific visualization tools via a slick interface
Understanding the Professional, Community, and Educational editions
There are three editions to PyCharm. I’ve alluded to two, because the third is a special version that is only useful to teachers, and the focus of this book is application development, not software development instruction. I’ll tell you about each, but I know what you want is a feature comparison chart. You’ll find it in Figure 1.13:
PyCharm Professional |
PyCharm Community |
|
Cost |
Paid |
Free |
Intelligent Python editor |
|
|
Graphical debugger and test runner |
|
|
Code navigation and refactor tools |
|
|
Code inspection |
|
|
Git, Subversion, and other source control tools |
|
|
Scientific tools |
|
|
Web development with HTML, JavaScript, CSS, and so on |
|
|
Python web framework support |
|
|
Performance profiling |
|
|
Remote development, containers, and so on |
|
|
Database and SQL support |
|
Figure 1.13: A feature comparison chart showing the features contained in the free Community edition versus the paid Professional version
The Community edition is free but only offers a limited set of features compared to the Professional edition. It is perfect for working on projects that only entail working with Python. The product I work on has a set of Python scripts that batch-process large amounts of data. Everything happens in Python and this is the perfect use case for the Community edition. If all you need is a terrific Python IDE, use the free version. The Community edition is also perfect if you are just working on automation scripts, such as graphics pipelines for 3D computer graphics, or general IT task automation.
The Professional edition has all the features of the free version but adds web development, database, remote development, containerization, and scientific project types. This is aimed at professionals who produce publishable software projects. While it is not free, JetBrains is pretty good about keeping it affordable with several pricing options, depending on how you are using the tool. Solo developers may obtain licenses at a lower price point than corporate developers. There are also ways to get the Professional edition free of charge, such as proving that you are using PyCharm on a fully open source software (FOSS) project. Start-up companies might be eligible for a 50% discount, and if you’re teaching in a code boot camp or a university setting, you may also qualify for free professional licenses. Since these things can fluctuate over time, you should check the JetBrains website for full details at https://www.jetbrains.com/pycharm/.
I said earlier there are three editions of PyCharm, and we’ve only covered two. The Educational edition is aimed at teachers and university professors developing curricula to teach Python. This edition can create and play back interactive lessons right in the IDE. It is only valuable to teachers, instructors, and content creators.
In this book, I will focus on the features present in the Community edition and the Professional edition.