Feature engineering for the baseline model
In this section, you will learn how to select features that are important in order to develop the predictive model. So right now, just to begin with, we won't focus much on deriving new features at this stage because first, we need to know which input variables / columns / data attributes / features give us at least baseline accuracy. So, in this first iteration, our focus is on the selection of features from the available training dataset.
Finding out Feature importance
We need to know which the important features are. In order to find that out, we are going to train the model using the Random Forest classifier. After that, we will have a rough idea about the important features for us. So let's get straight into the code. You can refer to the code snippet in the following figure:
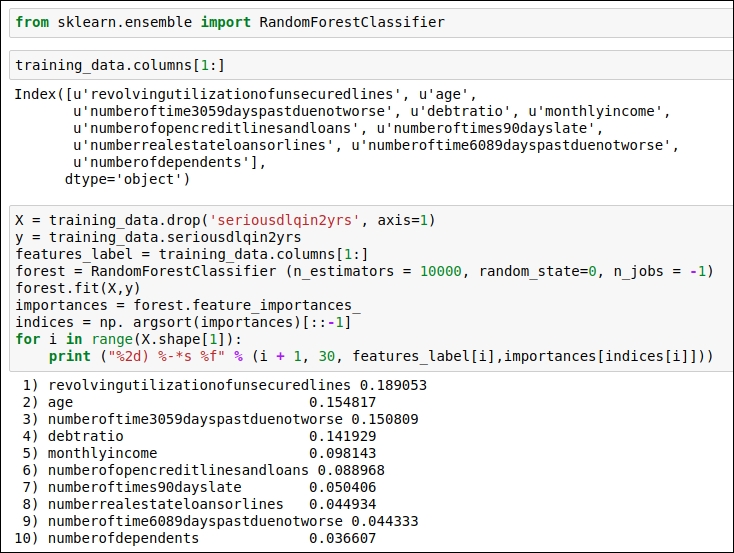
Figure 1.43: Derive the importance of features
In this code, we are using Random Forest Classifier from scikit-learn. We use the fit()
function to perform training, and then, in order to generate the importance of the features, we will use the feature_importances
_ function, which is available in the scikit-learn library. Then, we will print the features with the highest importance value to the lowest importance value.
Let's draw a graph of this to get a better understanding of the most important features. You can find the code snippet in the following figure:

Figure 1.44: Code snippet for generating a graph for feature importance
In this code snippet, we are using the matplotlib library to draw the graph. Here, we use a bar graph and feed in the values of all the data attributes and their importance values, which we previously derived. You can refer to the graph in the following figure:
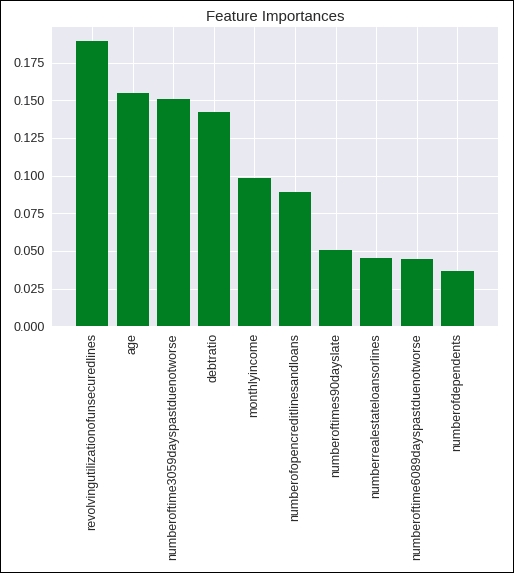
Figure 1.45: Graph of feature importance
For the first iteration, we did this quite some work on the feature engineering front. We will surely revisit feature engineering in the upcoming sections. Now it's time to implement machine learning algorithms to generate the baseline predictive model, which will give us an idea of whether a person will default on a loan in the next 2 years or not. So let's jump to the next section.