To appreciate what we can currently do with AI, we need to get a basic understanding of how the idea of emulating the human brain was born, and how this idea evolved to a point where we can easily solve tasks in vision and language with human-like capability through machines.
It all started in 1959 when a couple of Harvard scientists, Hubel and Wiesel, were experimenting with a cat's visual system by monitoring the primary visual cortex in the cat's brain.
The primary visual cortex is a collection of neurons in the brain placed at the back of the skull and is responsible for processing vision. It is the first part of the brain that receives input signals from the eye, very much like how a human brain would process vision.
The scientists started by showing complex pictures such as those of fish, dogs, and humans to the cat and observed its primary visual cortex. To their disappointment, they got no reading from the primary visual cortex initially. Consequently, to their surprise on one of the trials, as they were removing the slides, dark edges formed, causing some neurons to fire in the primary visual cortex:
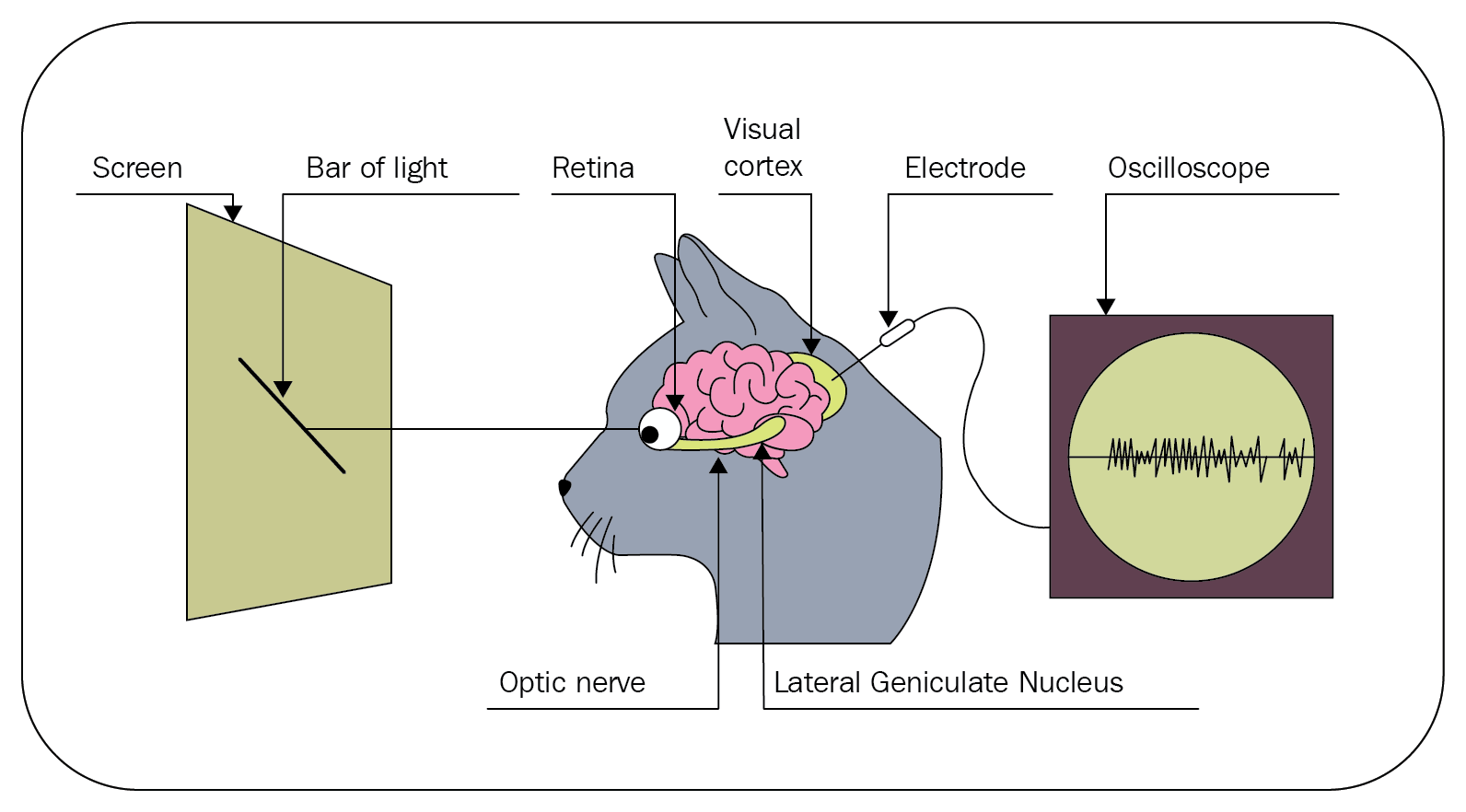
Their serendipitous discovery was that these individual neurons or brain cells in the primary visual cortex were responding to bars or dark edges at various specific orientations. This led to the understanding that the mammalian brain processes a very small amount of information at every neuron, and as the information is passed from neuron to neuron, more complex shapes, edges, curves, and shades are comprehended. So, all these independent neurons holding very basic information need to fire together to comprehend a complete complex image.
After that, there was a lull in the progress of how to emulate the mammalian brain until 1980, when Fukushima proposed neocognitron. Neocognitron is inspired by the idea that we should be able to create an increasingly complex representation using a lot of very simplistic representations – just like the mammalian brain!
The following is a representation of how neocognitron works, by Fukushima:
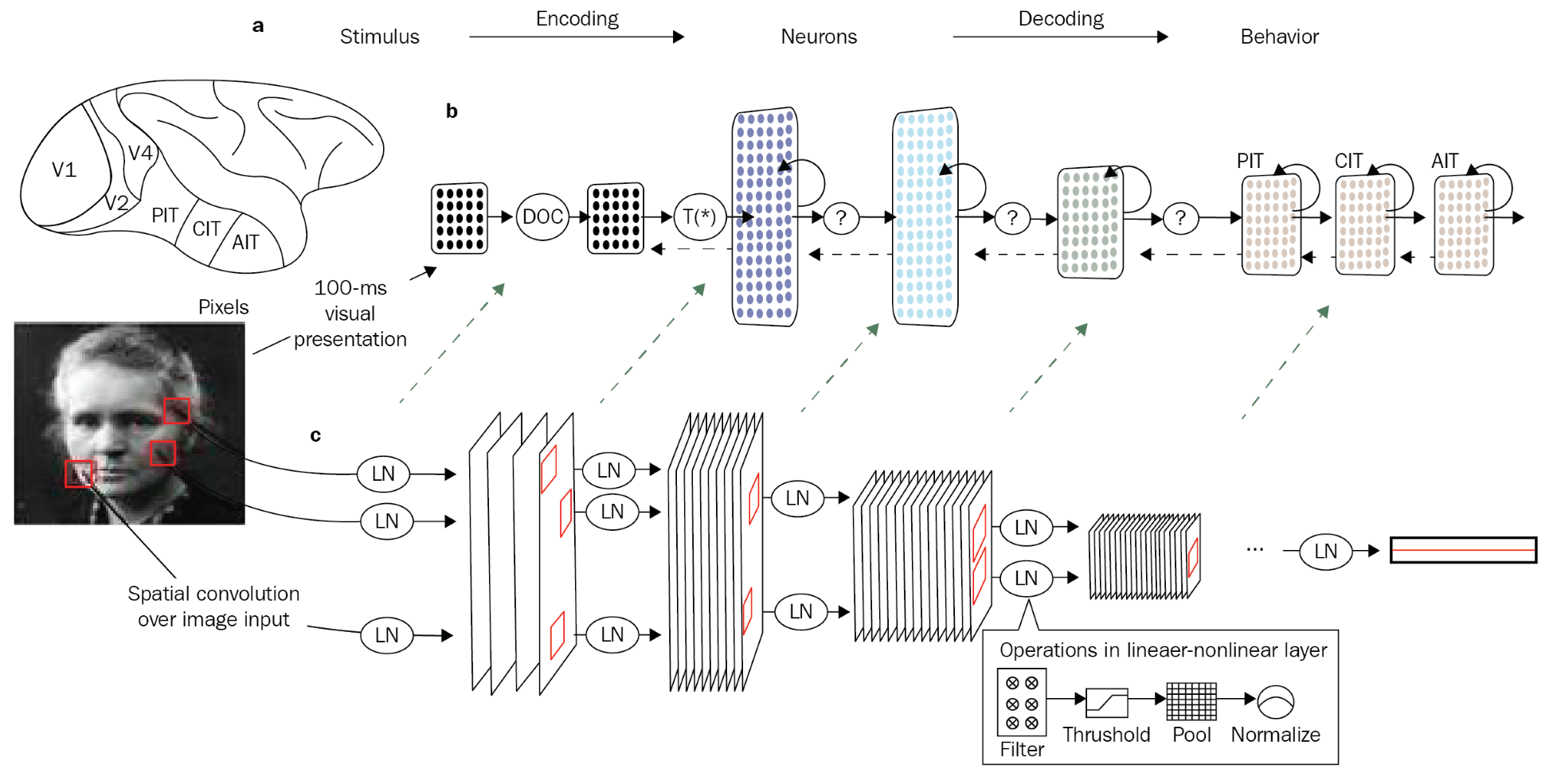
He proposed that to identify your grandmother, there are a lot of neurons that are triggered in the primary visual cortex, and each cell or neuron understands an abstract part of the final image of your grandmother. All of these neurons work in sequence, parallel, and tandem, and then finally hits a grandmother cell or neuron which fires only when it sees your grandmother.
Fast forward to today (2010-2018), with contributions from Yoshua Bengio, Yann LeCun, and Geoffrey Hinton, who are commonly known as the fathers of deep learning. They contribute massively to the AI space we work in today. They have given rise to a whole new approach to machine learning where feature engineering is automated.
The idea of not explicitly telling the algorithm what it should be looking for and letting it figure this out by itself by feeding it a lot of examples is the latest development. The analogy to this principle would be that of teaching a child to distinguish between an apple and an orange. We would show the child pictures of apples and oranges rather than only describing the two fruits' features, such as shape, color, size, and so on.
The following diagram shows the difference between ML and deep learning:
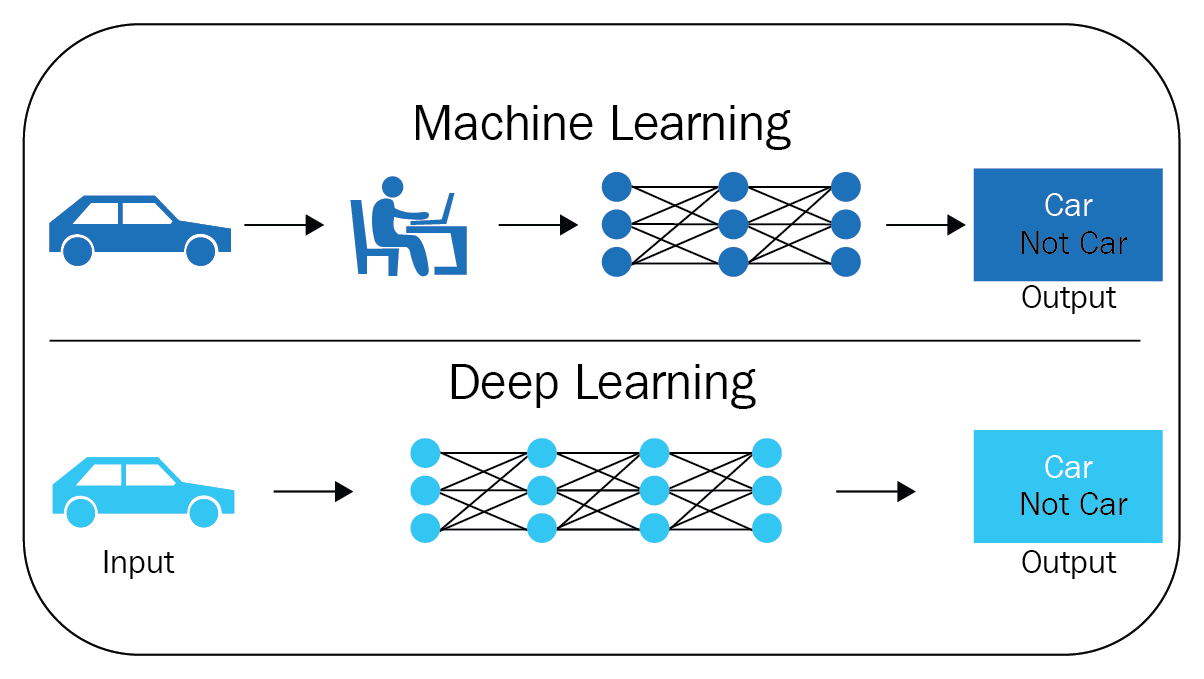
This is the primary difference between traditional ML and ML using neural networks (deep learning). In traditional ML, we provide features along with labels, but using ANNs, we let the algorithm decipher the features.
We live in an exciting time, an era we share with the fathers of deep learning, so much so that there are exchanges online in places such as Stack Exchange, where we can see contributions even from Yann LeCun and Geoffrey Hinton. This is analogous to living in the time of, and writing to, Nicholas Otto, the father of the internal combustion engine, who started the automobile revolution that we see evolving even to this day. The automobile revolution will be dwarfed by what could be possible with AI in the future. Exciting times, indeed!