Generative adversarial networks (GANs) are widely used for learning any data distribution and imitating it. GANs consist of two networks; one is the generator, which generates new synthetic instances of data from a normal or uniform distribution, while the other is the discriminator, which evaluates the generated instances and checks if they are authentic – that is, they belong to the original training data distribution or not. The generator and discriminator are pitted against each other in a counterfeiter and cop scenario where the goal of the counterfeiter is to fool the cop by generating false data and the cop's role is to detect the lies. The feedback from the discriminator is passed on to the generator so that it can improvise at each iteration. Note that although both networks optimize a different and opposite objective...
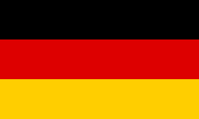
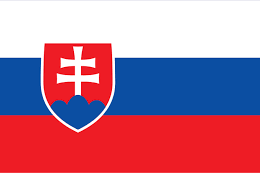
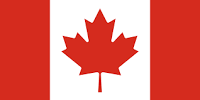
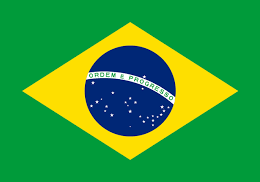

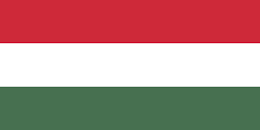
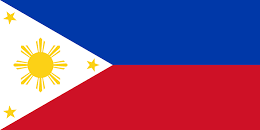
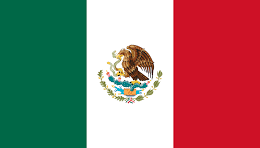
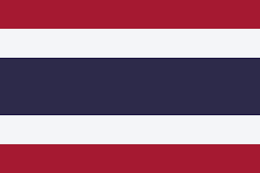
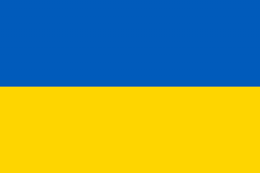
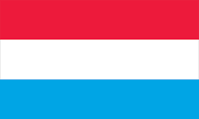
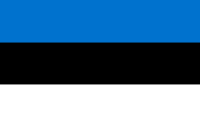
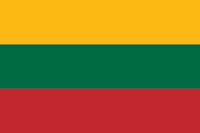
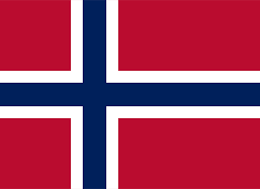
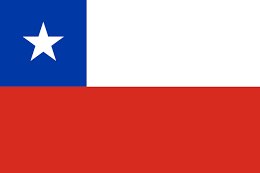
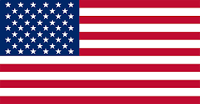
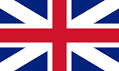
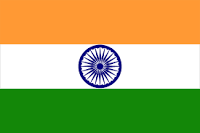
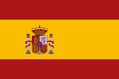
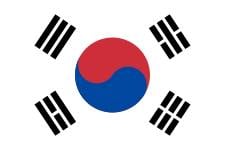
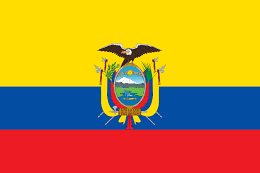
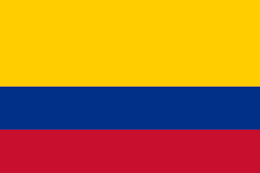
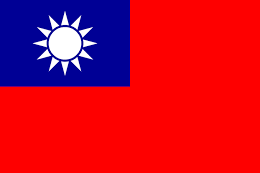
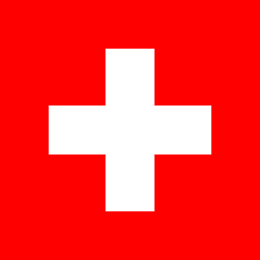
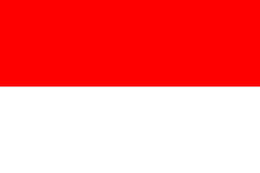
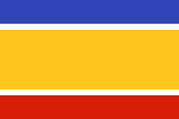
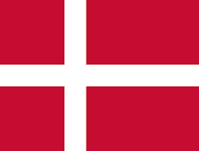
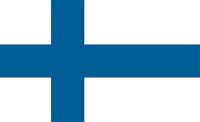
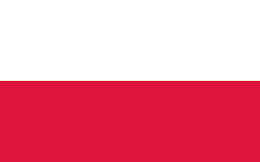
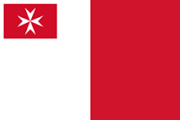
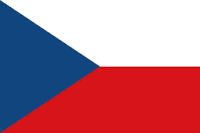
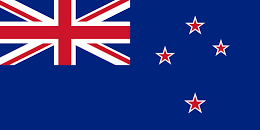
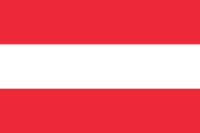
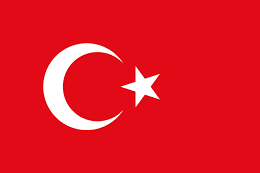
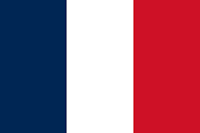
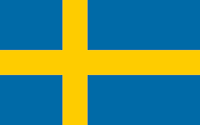
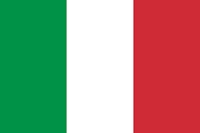
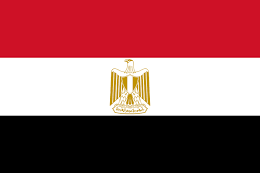
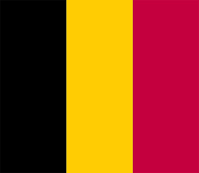
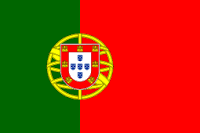
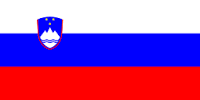
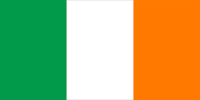
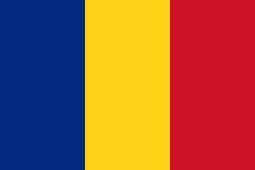
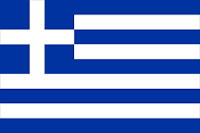
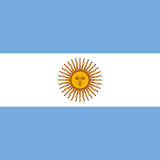
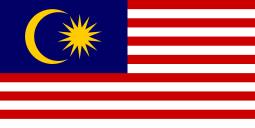
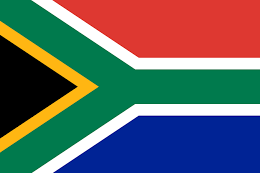
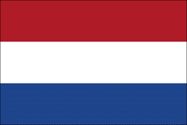
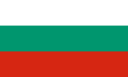
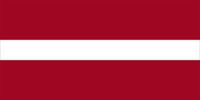
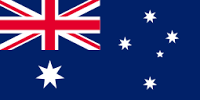
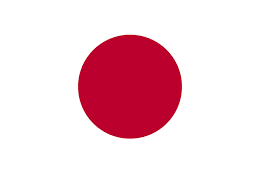
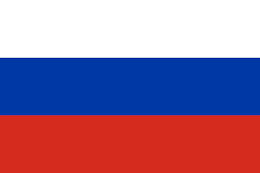