So, let's try to better understand how the different parts of the GAN work together to generate synthetic data. Consider the parameterized function (G) (you know, the kind we usually approximate using a neural network). This will be our generator, which samples its input vectors (z) from some latent probability distribution, and transforms them into synthetic images. Our discriminator network (D), will then be presented with some synthetic images produced by our generator, mixed among real images, and attempt to classify real from forgery. Hence, our discriminator network is simply a binary classifier, equipped with something like a sigmoid activation function. Ideally, we want the discriminator to output high values when presented with real images, and low values when presented with generated fakes. Conversely, we want our generator network to try...
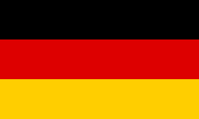
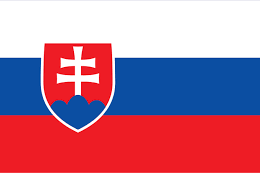
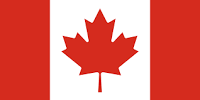
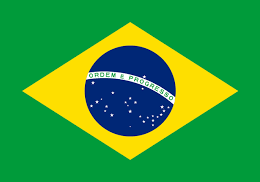

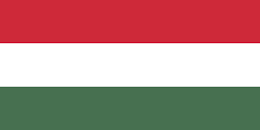
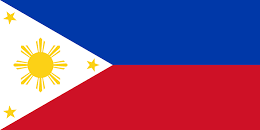
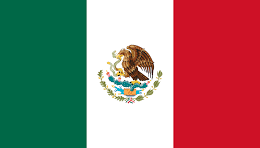
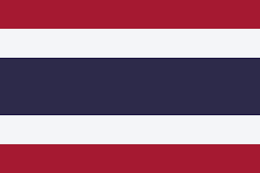
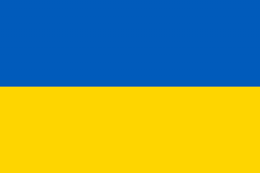
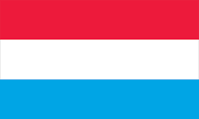
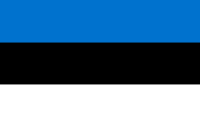
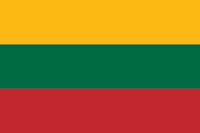
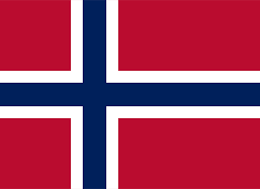
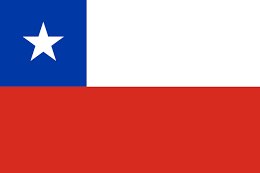
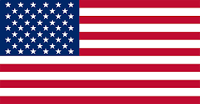
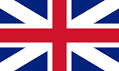
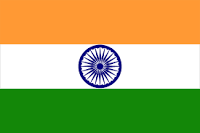
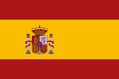
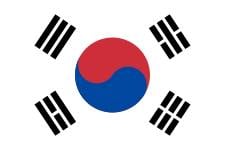
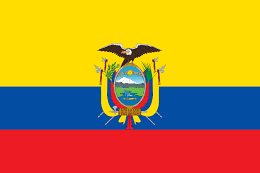
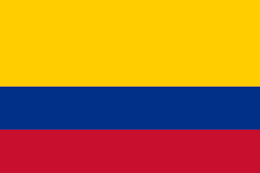
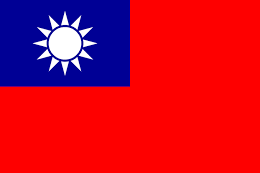
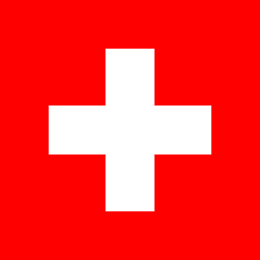
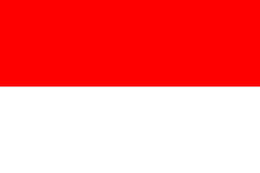
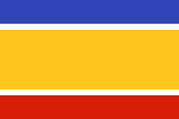
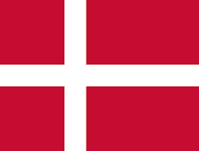
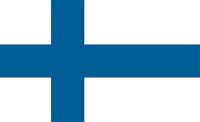
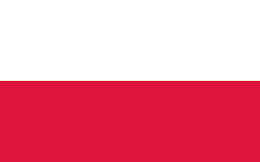
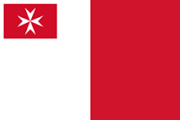
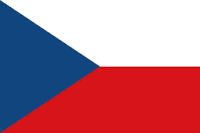
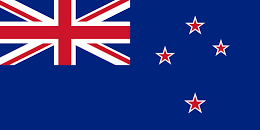
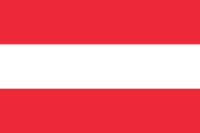
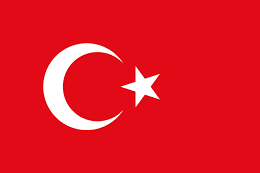
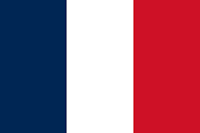
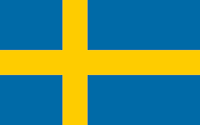
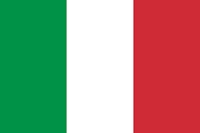
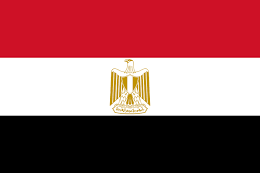
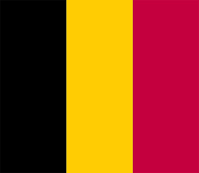
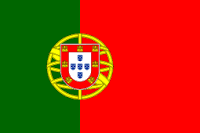
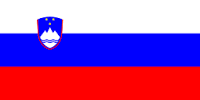
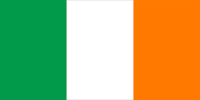
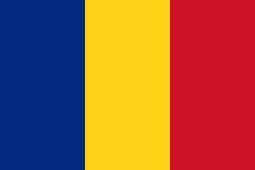
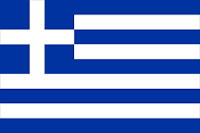
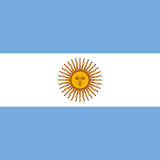
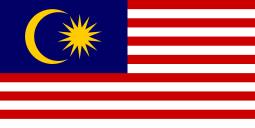
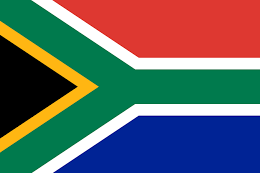
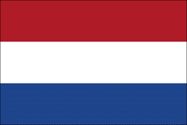
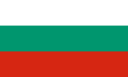
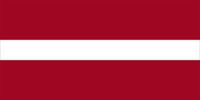
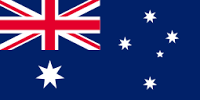
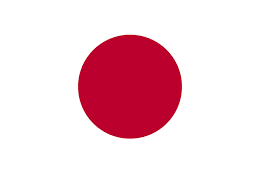
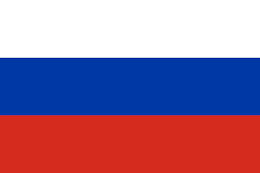